Bayesian Quickest Change-Point Detection With an Energy Harvesting Sensor and Asymptotic Analysis
IEEE TRANSACTIONS ON SIGNAL PROCESSING(2024)
摘要
This paper studies the problem of the quickest change-point detection by a sensor powered by randomly available energy harvested from the environment under a Bayesian framework. In particular, the sensor observes a stochastic process by taking and processing samples at discrete times. We assume the distribution of the sampled data changes at an unknown random time, and the pre and post-change distributions are stationary and known. In the proposed framework, the sensor takes a new sample if there is enough evidence of a change. Otherwise, the sensor saves energy for the future and does not take new samples. The optimal policy is obtained by dynamic programming that minimizes the average detection delay for fixed upper bounds on the false alarm rate and an average number of samples taken before the change point. We model the test statistics as a perturbed random walk and study the asymptotic performance of the proposed method applying non-linear renewal theory under two different scenarios. First, (H) over bar >= E-s, where (H) over bar is the average harvested energy in one sampling period and E-s is the energy needed to take and process a new sample, and second, (H) over bar < E-s. For the first scenario, the optional policy turns out to be greedy, i.e., the sensor takes samples when sufficient energy is available. However, under the second scenario, the proposed method performs better than the greedy approach since it prepares for the future and uses available energy parsimoniously. We have provided several numerical results to support the derived theory.
更多查看译文
关键词
Bayesian quickest change-point detection,non-linear Markov chain,non-linear renewal theorem,irreducibility,recurrence,sequential detection,energy harvesting sensors
AI 理解论文
溯源树
样例
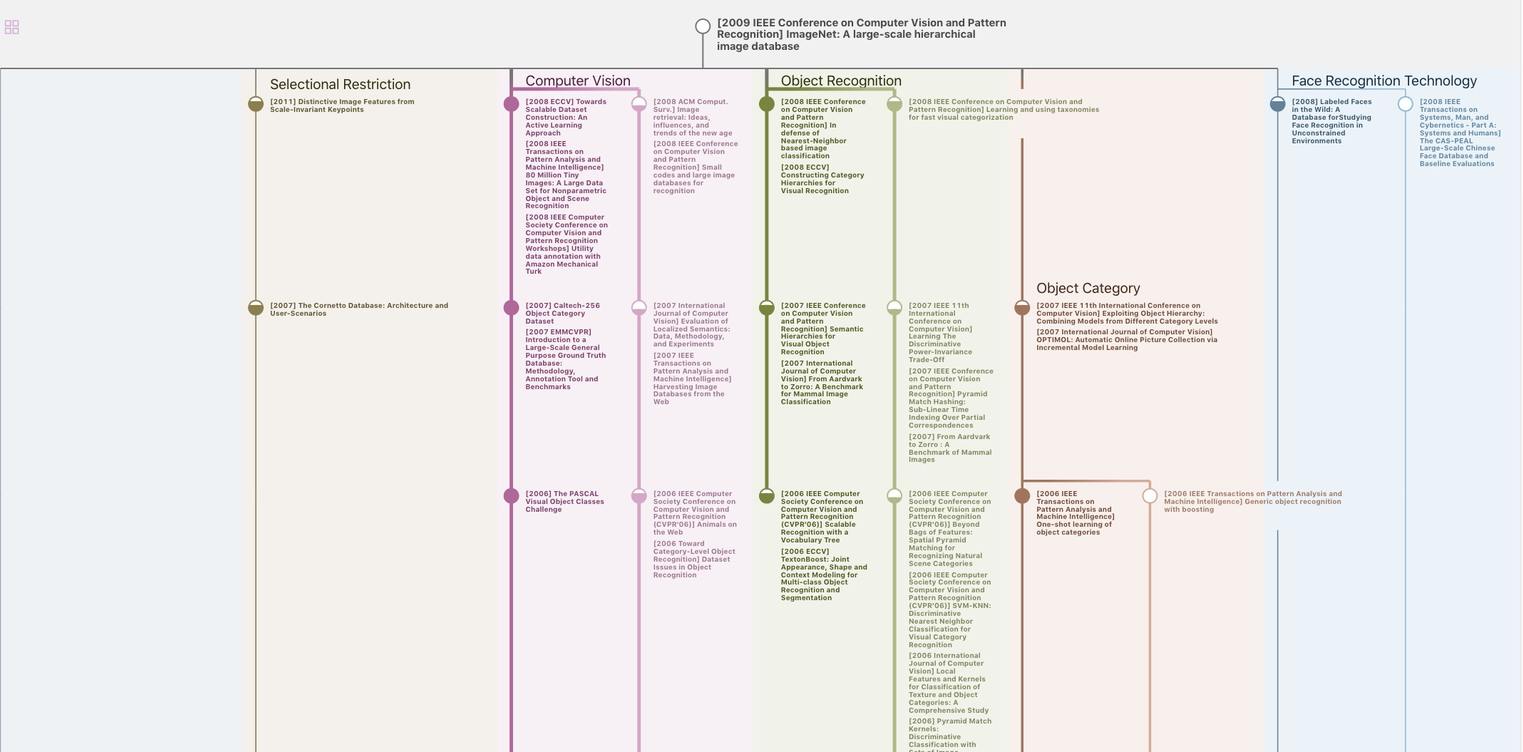
生成溯源树,研究论文发展脉络
Chat Paper
正在生成论文摘要