Towards a Unified End-to-End Language Understanding System for Speech and Text Inputs.
2023 IEEE Automatic Speech Recognition and Understanding Workshop (ASRU)(2023)
摘要
End-to-end (E2E) spoken language understanding (SLU) systems facilitate mapping speech inputs directly to semantic outputs, eliminating the need for modular processing of speech-to-text and text-to-semantics sub-tasks using separate models. However, they are now limited to processing speech inputs only, and are not flexible to deal with plain texts. In this paper, we propose an E2E spoken and natural language understanding (SNLU) system that can handle both speech and text within a unified architecture. The system follows the Mask-CTC non-autoregressive approach, and the input flexibility is acquired by partially sharing the decoder between SLU and NLU tasks. Experiments on the SLURP dataset show that the proposed architecture achieves similar performance to using separate E2E SLU and NLU modules, but with relatively 43.7 % less model parameters. We also explore the use of pre-trained speech and language models into the SNLU system, and show that they further improve the performance.
更多查看译文
关键词
spoken language understanding,natural language understanding,non-autoregressive modelling
AI 理解论文
溯源树
样例
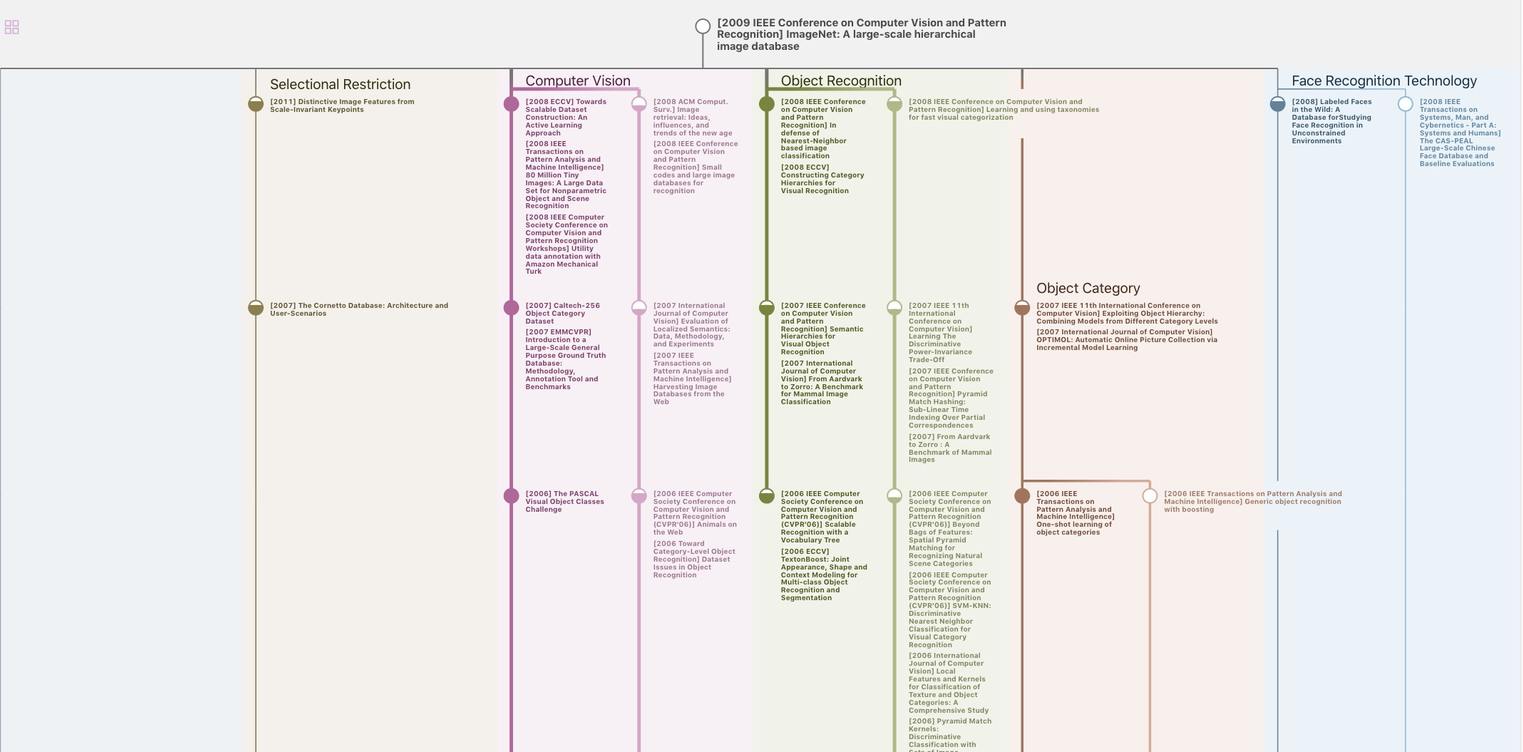
生成溯源树,研究论文发展脉络
Chat Paper
正在生成论文摘要