Object Detection of Remote Sensing Image Based on Multi-Scale Feature Fusion and Attention Mechanism
IEEE ACCESS(2024)
摘要
In view of the small size and dense distribution of remote sensing image targets, this paper adds a detection head P2 specifically for small-scale targets on the basis of the three detection layers of the original YOLOv5 model, and involves the shallow high-resolution feature map in the subsequent multi-scale feature fusion module. The problem of losing the key feature information of the small-scale target in the process of multiple downsampling is effectively avoided. Firstly, an enhanced multi-scale feature fusion pyramid network DSI-FPN is designed. The FPN+PAN network is optimized by using DepthwiseSparable Convolution and Involution operators with fewer parameters and computations, as well as a spatial attention mechanism to generate feature graphs with richer information for network detection tasks. Secondly, we propose an adaptive channel spatial attention mechanism SCBAM, which introduces a self-attention mechanism into CBAM module to add non-local information to the interaction that originally had only local information, breaks the convolution kernel limit, expands the model receptive field, and improves the feature expression ability of the model. Thirdly, in order to solve the problem of insufficient computing power when deploying the target detector for equipment, we propose a network knowledge distillation framework for joint teachers based on the feature layer. The distillation loss of teacher is designed, and the trend of student online learning is adjusted dynamically by balancing the contributions of teacher network and truth value. The detection accuracy of the student network is obviously improved, and the parameters and model size of the network are effectively reduced. Finally, Comparing with other remote sensing image object detection methods, the experimental results show that the approach presented has better detection effect for small-scale targets of remote sensing images under different lighting conditions. The detection accuracy reached 43.9%, and 7.4% higher than that of the original model. After knowledge distillation, the model parameters are reduced to 1/3 of the original, and the detection accuracy is 40.2%.
更多查看译文
关键词
Deep Learning,object detection,remote sensing image,multi-scale feature fusion pyramid network,adaptive channel spatial attention mechanism,joint teacher knowledge distillation
AI 理解论文
溯源树
样例
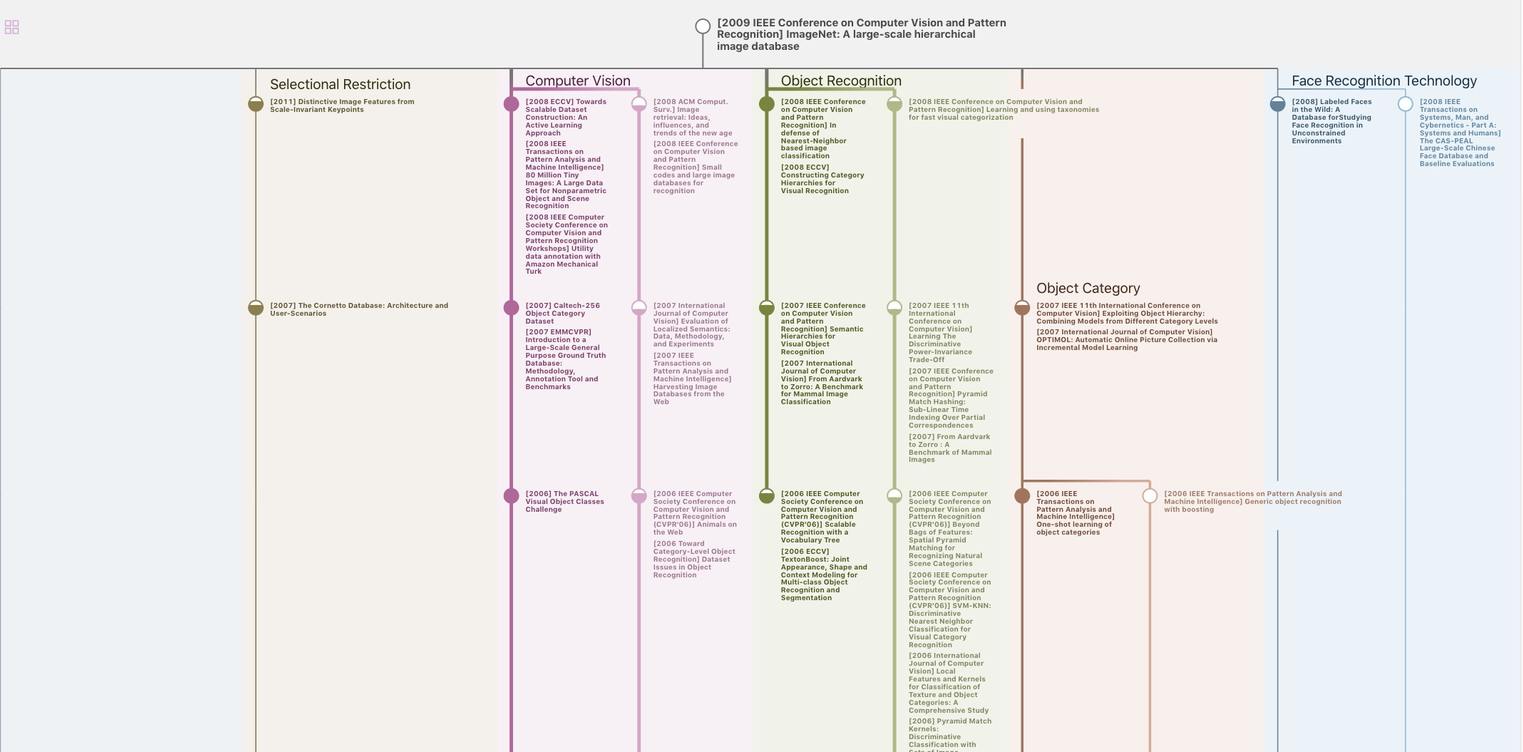
生成溯源树,研究论文发展脉络
Chat Paper
正在生成论文摘要