Large language models for biomolecular analysis: From methods to applications
TRAC-TRENDS IN ANALYTICAL CHEMISTRY(2024)
摘要
Large language models (LLMs) are proving to be very useful in many fields, especially chemistry and biology, because of their amazing capabilities. Biomolecular data is often represented sequentially, much like textual data used to train LLMs. However, developing LLMs from scratch requires a substantial amount of data and computational resources, which may not be feasible for most researchers. A more workable solution to this problem is to change the inputs or parameters so that the previously trained general LLMs can pick up the specific knowledge needed for biomolecular analysis. These adaption strategies lower the amount of data and hardware needed, providing a more affordable option. This review provides the introduction of two popular LLM adaptation techniques: fine-tuning and prompt engineering, along with their uses in the analysis of molecules, proteins, and genes. A thorough overview of current common datasets and pre-trained models is also provided. This review outlines the possible advantages and difficulties of LLMs for biomolecular analysis, opening the door for chemists and biologists to effectively utilize LLMs in their future studies.
更多查看译文
关键词
Large language model,Biomolecular analysis,Fine-tuning,Prompt engineering,Parameter -efficient fine-tuning,In -context learning,Protein structure analysis,Protein sequence generation,Gene sequence analysis,Molecular representation learning
AI 理解论文
溯源树
样例
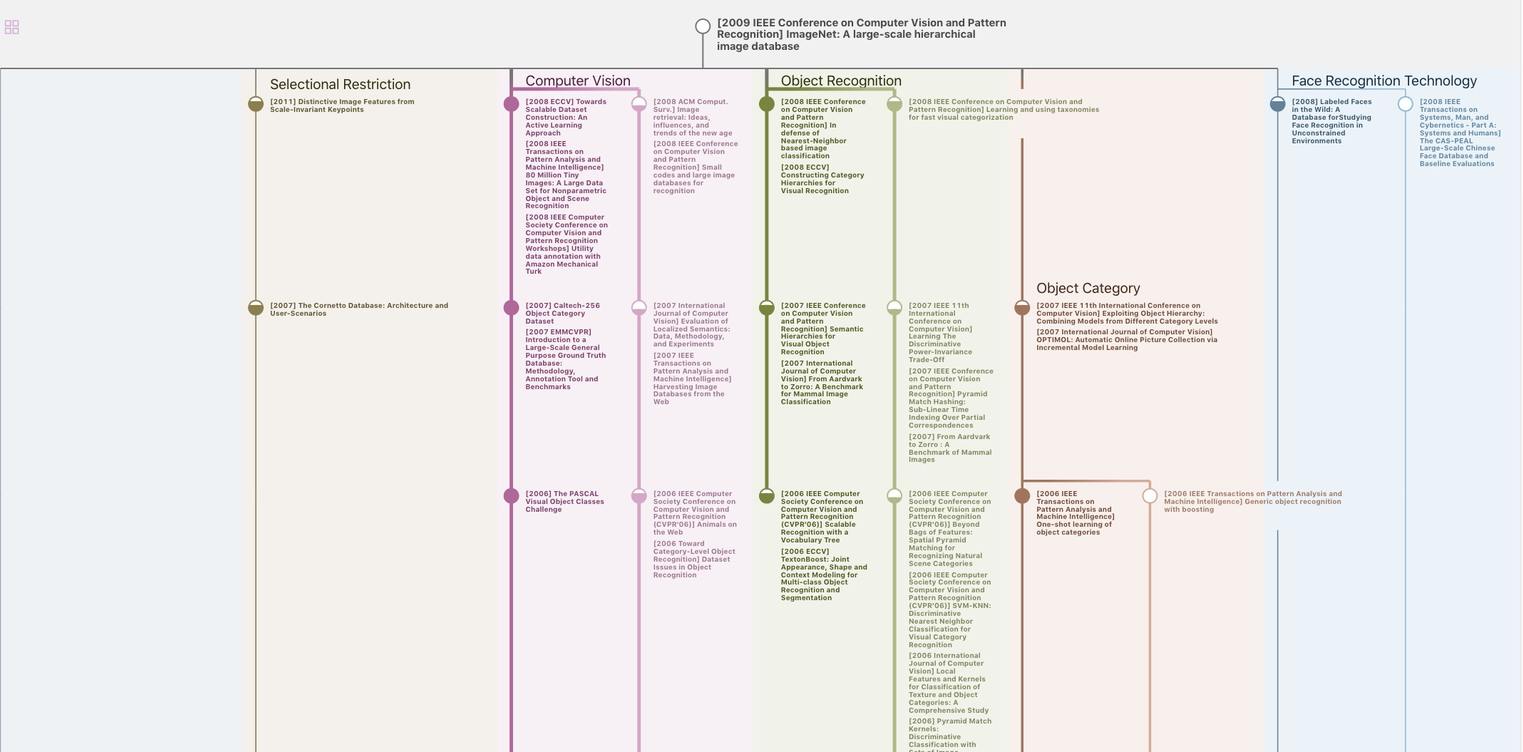
生成溯源树,研究论文发展脉络
Chat Paper
正在生成论文摘要