Graph structure few-shot prognostics for machinery remaining useful life prediction under variable operating conditions
ADVANCED ENGINEERING INFORMATICS(2024)
摘要
Graph neural network (GNN) based decision-making can easily capture edge and node traits to construct and extract relevant deep features, but domain shifts among graph data will remain particularly prominent when the amount of data decreases sharply. In this study, GNN based machinery prognostics is further explored with partial monitoring data for the first time, and the graph structure based few-shot prognostics framework is established to overcome the challenge of limited graph samples based predictions under variable operation conditions. In particular, spatial-temporal graph structure data that aggregate multi-source, multi-channel signals are first constructed. Then a novel graph prediction algorithm embedded in the meta-learning training model, named graph structure data enhanced meta-learning network, is proposed and has the functions of spatial-temporal graph data processing and cross-domain generalization under few-shot training mode. Finally, two real-measured run-to-failed mechanical durability tests are utilized to prove the superiority of the proposed prognostics method.
更多查看译文
关键词
Graph neural network,Few -shot prognostics,Meta learning,Remaining useful life prediction
AI 理解论文
溯源树
样例
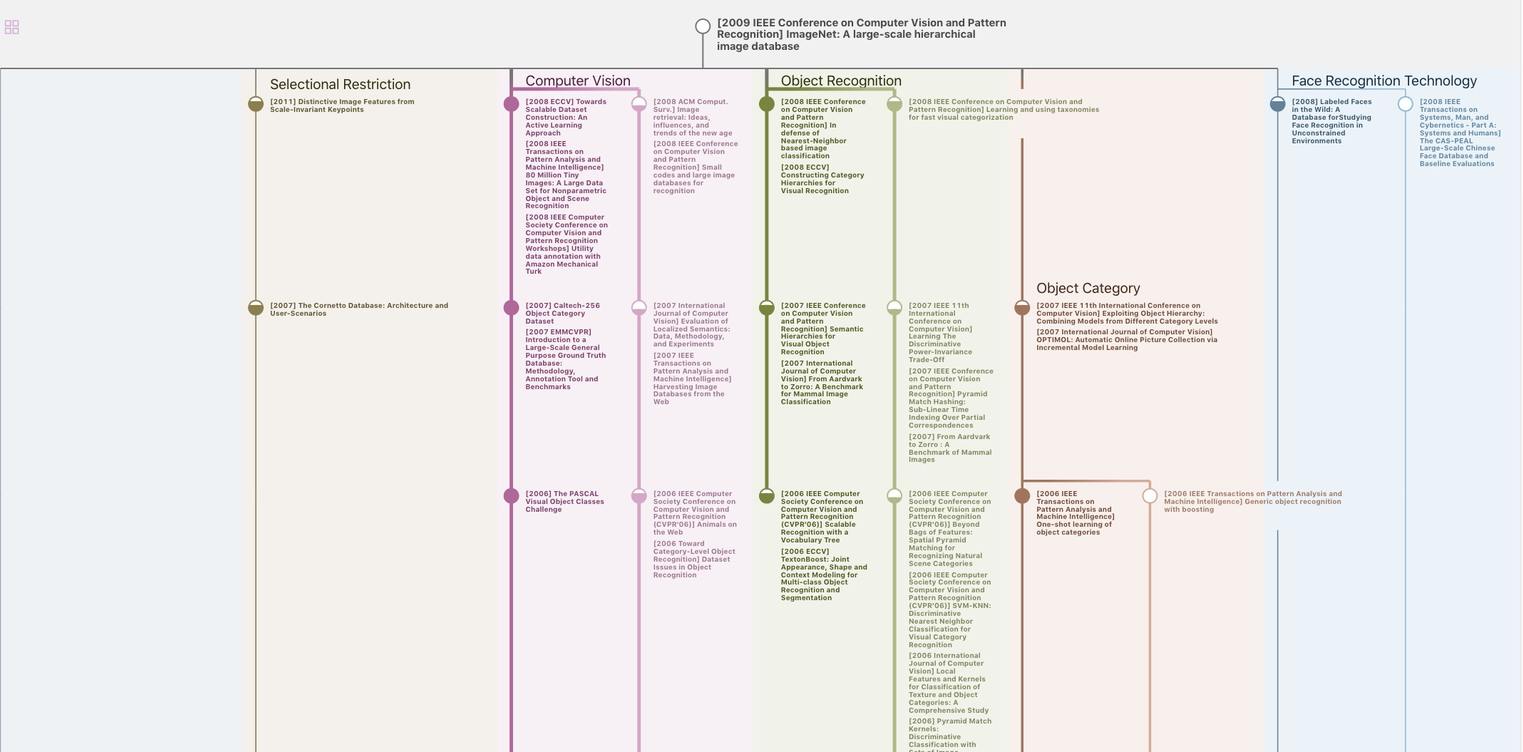
生成溯源树,研究论文发展脉络
Chat Paper
正在生成论文摘要