LA-Net: layer attention network for 3D-to-2D retinal vessel segmentation in OCTA images
PHYSICS IN MEDICINE AND BIOLOGY(2024)
摘要
Objective. Retinal vessel segmentation from optical coherence tomography angiography (OCTA) volumes is significant in analyzing blood supply structures and the diagnosing ophthalmic diseases. However, accurate retinal vessel segmentation in 3D OCTA remains challenging due to the interference of choroidal blood flow signals and the variations in retinal vessel structure. Approach. This paper proposes a layer attention network (LA-Net) for 3D-to-2D retinal vessel segmentation. The network comprises a 3D projection path and a 2D segmentation path. The key component in the 3D path is the proposed multi-scale layer attention module, which effectively learns the layer features of OCT and OCTA to attend to the retinal vessel layer while suppressing the choroidal vessel layer. This module also efficiently captures 3D multi-scale information for improved semantic understanding during projection. In the 2D path, a reverse boundary attention module is introduced to explore and preserve boundary and shape features of retinal vessels by focusing on non-salient regions in deep features. Main results. Experimental results in two subsets of the OCTA-500 dataset showed that our method achieves advanced segmentation performance with Dice similarity coefficients of 93.04% and 89.74%, respectively. Significance. The proposed network provides reliable 3D-to-2D segmentation of retinal vessels, with potential for application in various segmentation tasks that involve projecting the input image. Implementation code: https://github.com/y8421036/LA-Net.
更多查看译文
关键词
retinal vessel segmentation,3D-to-2D,multi-scale layer attention,reverse boundary attention,OCTA volume
AI 理解论文
溯源树
样例
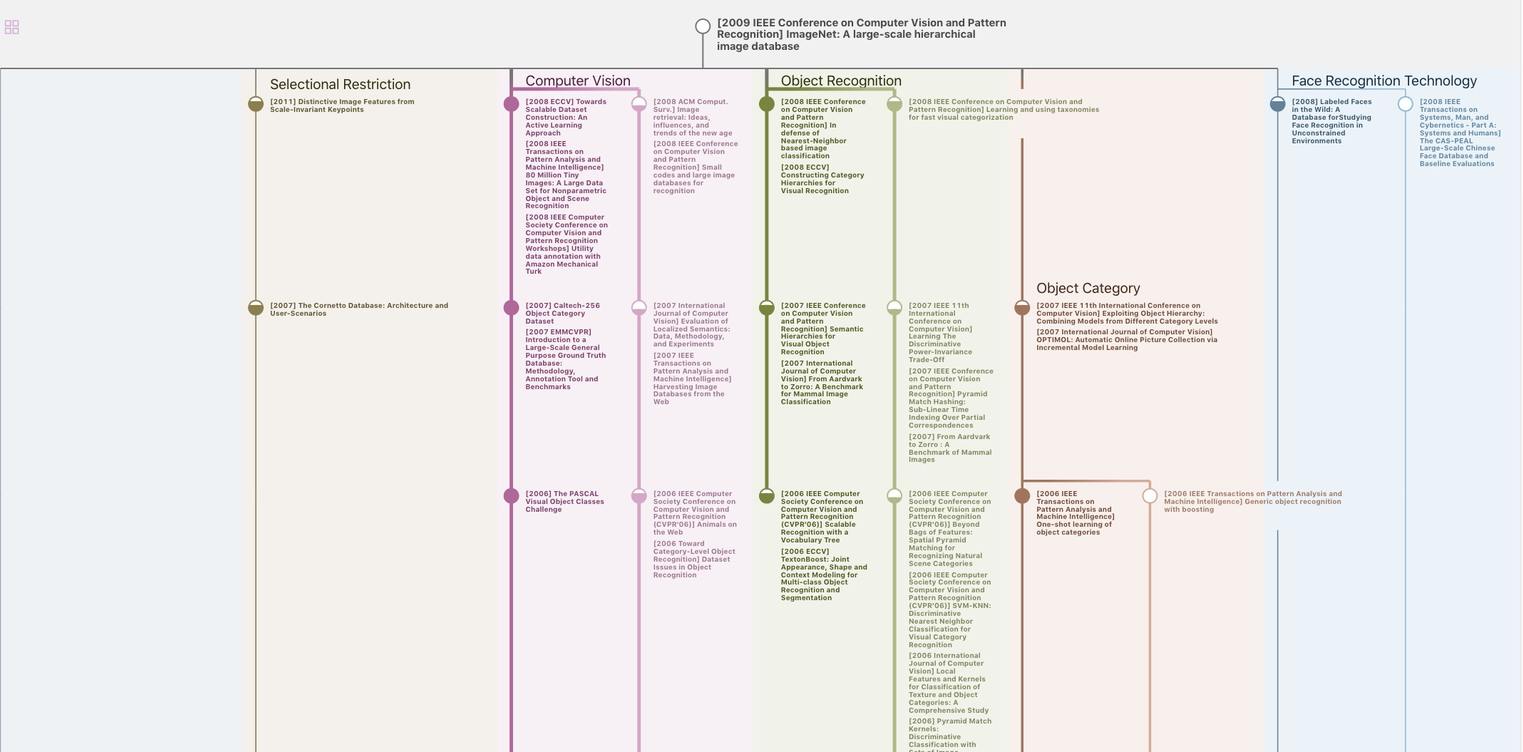
生成溯源树,研究论文发展脉络
Chat Paper
正在生成论文摘要