A particle swarm optimization algorithm based on modified crowding distance for multimodal multi-objective problems
APPLIED SOFT COMPUTING(2024)
摘要
Multimodal multi -objective optimization problems (MMOPs) are commonly encountered in practice. The difficulty in solving MMOPs is obtaining all of the Pareto optimal sets without degrading the performance of the Pareto optimal front. To address this challenge, this study proposes a particle swarm optimization algorithm based on modified crowding distance (MOPSO_MCD). In MOPSO_MCD, a modified method for calculating the crowding distance (MCD) is devised, which allows for a more comprehensive assessment of the crowding relationship between individuals in the decision space and the objective space. Moreover, a cosine similaritybased elite selection mechanism is designed to identify the neighborhood optimal individuals of the individuals in the population and improve the decision space diversity. Additionally, an offspring competition mechanism is proposed to keep the population from trapping in the local optimum and enhance the global search ability of the MOPSO_MCD algorithm. Experimental results and statistical analysis show that MOPSO_MCD performs better than the other comparison algorithms on sixteen test functions and a map -based practical problem.
更多查看译文
关键词
MMOPs,Particle swarm optimization,Modified crowding distance,MMEAs
AI 理解论文
溯源树
样例
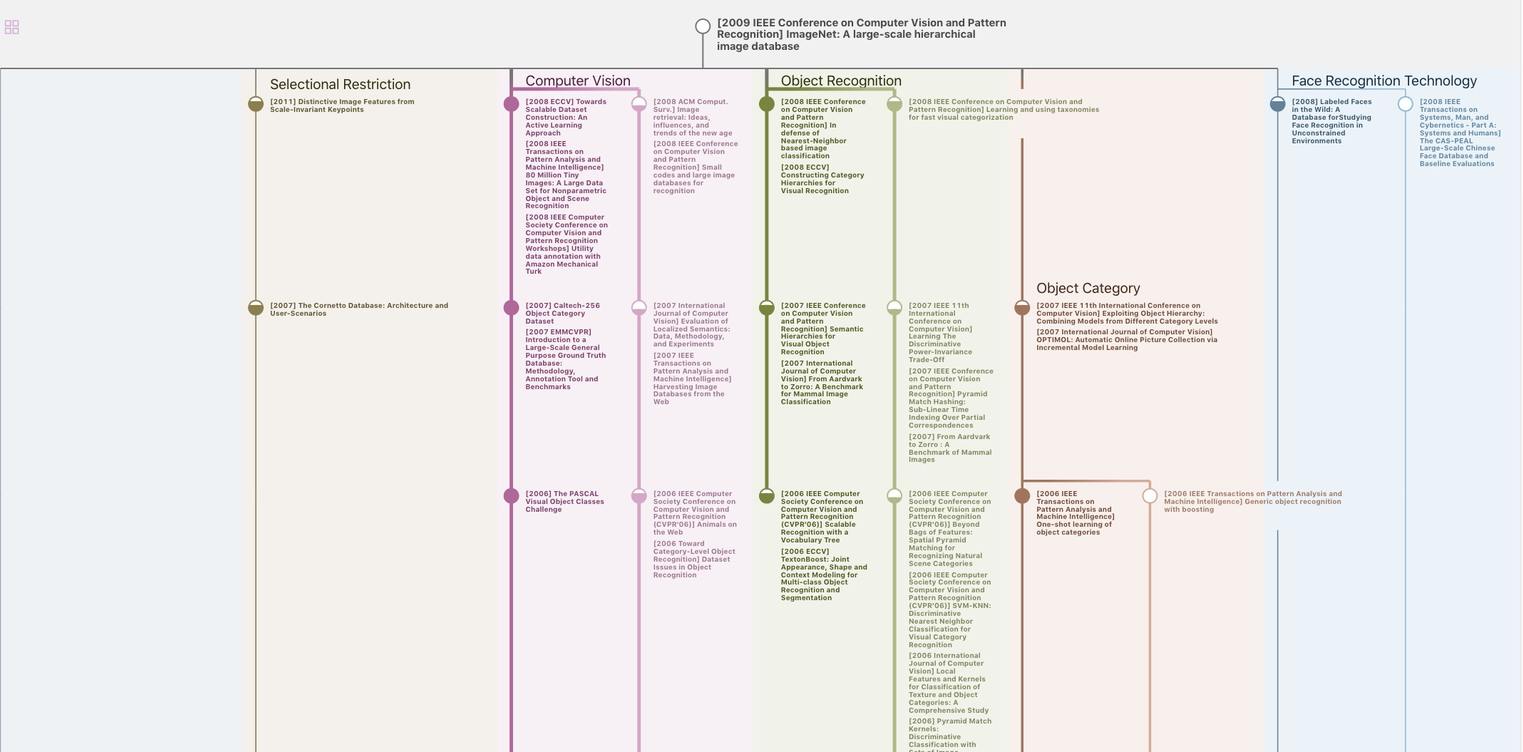
生成溯源树,研究论文发展脉络
Chat Paper
正在生成论文摘要