How to train a post-processor for tandem mass spectrometry proteomics database search while maintaining control of the false discovery rate
biorxiv(2024)
摘要
Canonical procedures to control the false discovery rate (FDR) among the list of putative discoveries rely on our ability to compute informative p-values. Competition-based approach offers a fairly novel and increasingly popular alternative when computing such p-values is impractical. The popularity of this approach stems from its wide applicability: instead of computing p-values, which requires knowing the entire null distribution for each null hypothesis, a competition-based approach only requires a single draw from each such null distribution. This drawn example is known as a "decoy" in the mass spectrometry community (which was the first to adopt the competition approach) or as a "knockoff" in the statistics community. The decoy is competed with the original observation so that only the higher scoring of the two is retained. The number of decoy wins is subsequently used to estimate and control the FDR among the target wins. In this paper we offer a novel method to extend the competition-based approach to control the FDR while taking advantage of side information, i.e., additional features that can help us distinguish between correct and incorrect discoveries. Our motivation comes from the problem of peptide detection in tandem mass spectrometry proteomics data. Specifically, we recently showed that a popular mass spectrometry analysis software tool, Percolator, can apparently fail to control the FDR. We address this problem here by developing a general protocol called "RESET" that can take advantage of the additional features, such as the ones Percolator uses, while still theoretically and empirically controlling the FDR.
### Competing Interest Statement
The authors have declared no competing interest.
更多查看译文
AI 理解论文
溯源树
样例
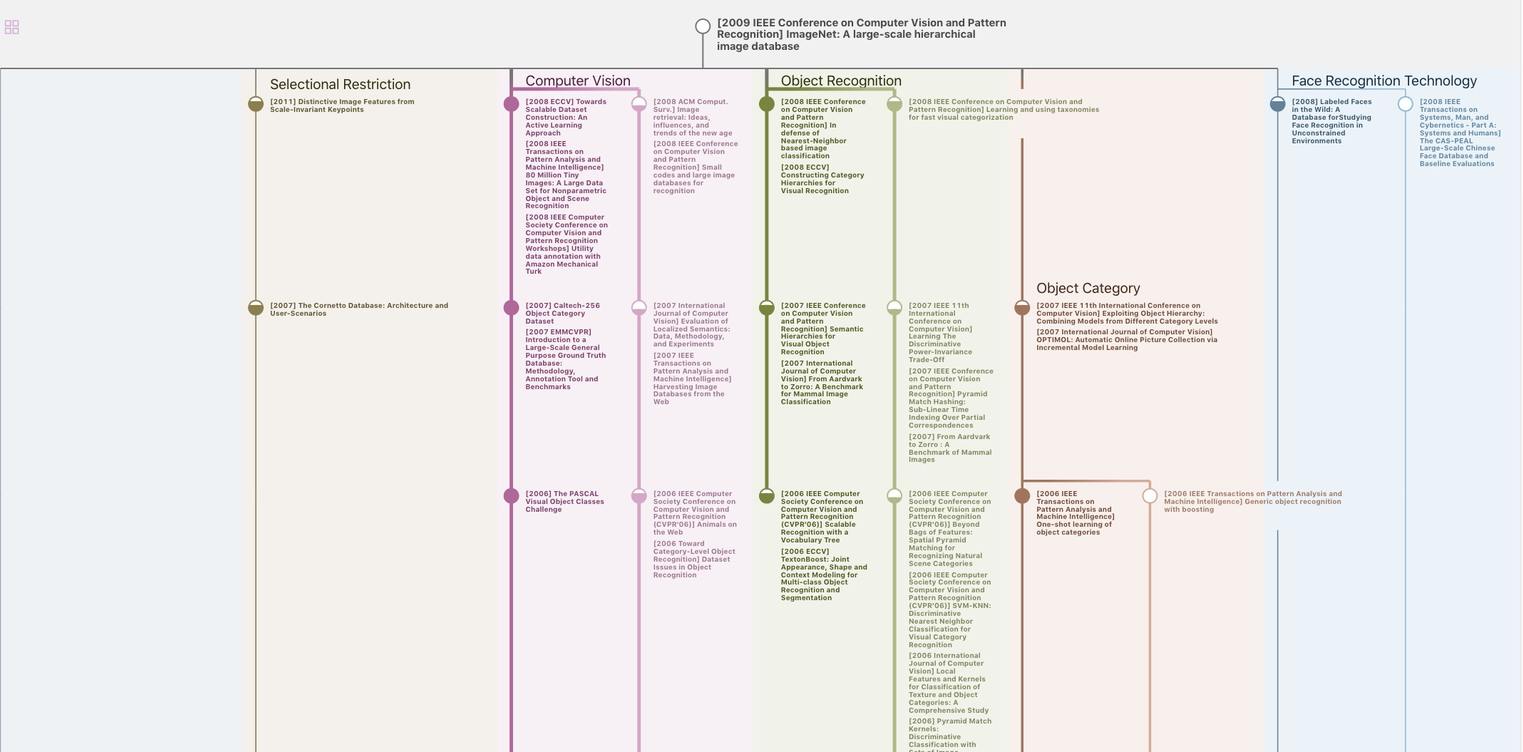
生成溯源树,研究论文发展脉络
Chat Paper
正在生成论文摘要