DOCNet: Dual-Domain Optimized Class-Aware Network for Remote Sensing Image Segmentation
IEEE GEOSCIENCE AND REMOTE SENSING LETTERS(2024)
摘要
The spatial attention mechanism has been frequently employed for the semantic segmentation of remote sensing images, given its renowned capability to model long-range dependencies. As remote sensing images often exhibit intricate backgrounds, significant intraclass variability, and a foreground-background imbalance, spatial attention mechanism-based methods somehow tend to introduce an extensive amount of background context through intensive affinity operations, causing unsatisfactory segmentation outcomes. While several class-aware methods attempt to attenuate the interference of background context by generating class representations as representative features, they still encounter challenges related to independent correlation calculation and single-confidence scale class representations. We introduce a dual-domain optimized class-aware network designed to address these challenges. In the semantic domain, we use category confidence as a scaling criterion to derive class representations at multiple confidence scales, effectively modeling pixel-class relationships. In the spatial domain, we leverage pixel-class relationships and their consensus to enhance relevant correlations while suppressing erroneous ones. Experimental results on three datasets demonstrate that the proposed method surpasses previous state-of-the-art ones for remote sensing image segmentation. Code is available at https://github.com/xwmaxwma/rssegmentation.
更多查看译文
关键词
Class-aware,pixel-class relation,remote sensing,semantic segmentation
AI 理解论文
溯源树
样例
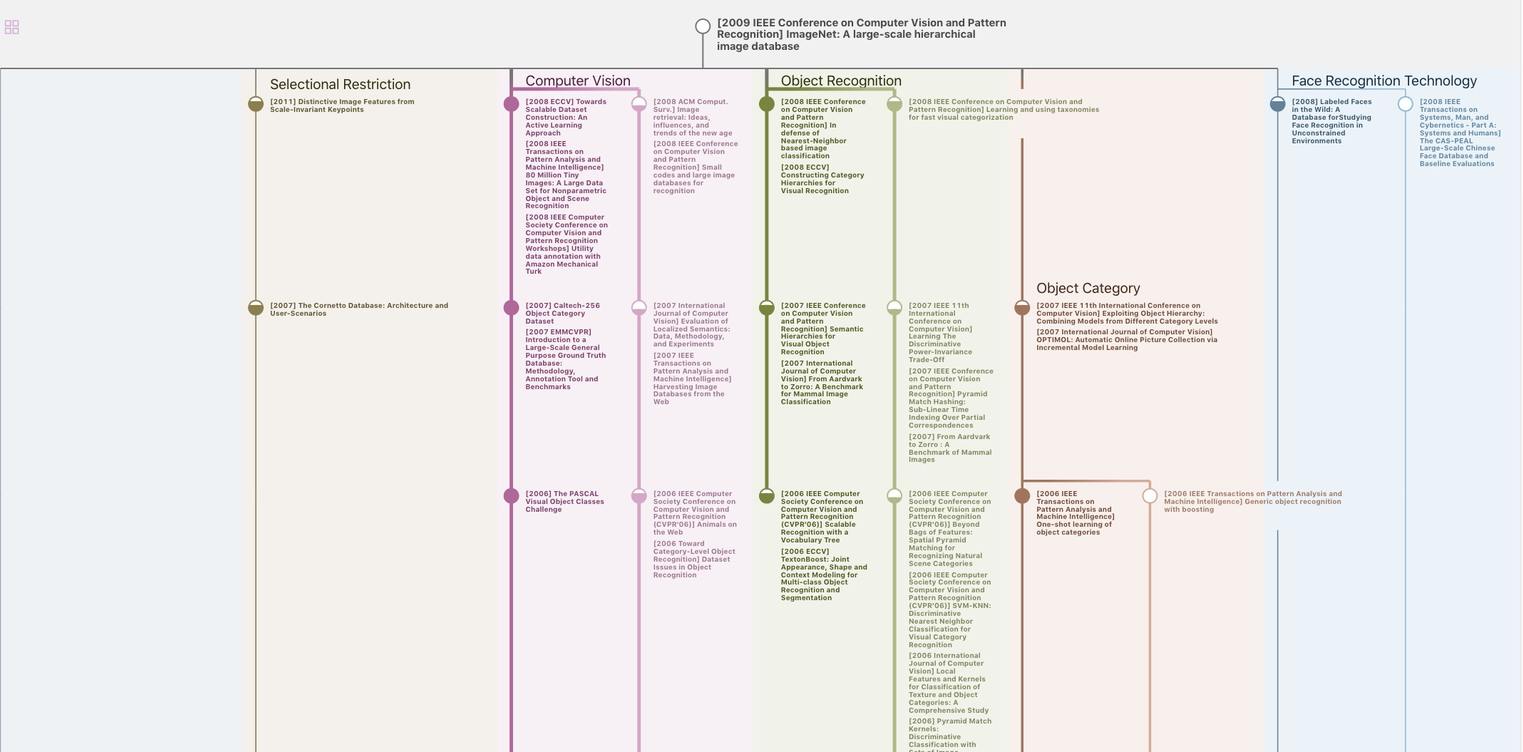
生成溯源树,研究论文发展脉络
Chat Paper
正在生成论文摘要