Bayesian Approach Integrating Prior Knowledge for Identifying miRNA-mRNA Interactions in Hepatocellular Carcinoma.
2023 IEEE International Conference on Bioinformatics and Biomedicine (BIBM)(2023)
摘要
Oncogenesis, a complex and multifaceted process, is profoundly modulated by miRNA’s regulatory role in gene expression. Over the years, a substantial body of knowledge concerning miRNA and mRNA has been accumulated, drawing from both rigorous biological experiments and intricate statistical analyses. In the realm of statistical modeling, the integration of such information as "prior knowledge" often amplifies the model’s ability to pinpoint molecular targets of significance. This study seeks to leverage prior knowledge of miRNA-mRNA regulatory interactions to map the dynamic landscape of interactions in the specific context of hepatocellular carcinoma (HCC).To address this, we introduce an evolved iteration of a Bayesian two-step integrative method previously established in the literature. This augmented approach includes improved computing efficiency when dealing with high dimensional data and a novel mechanistic submodel, which operates autonomously, devoid of prior knowledge. Employing this method, we identified two discrete gene lists: one informed by prior knowledge and the other independently inferred. This bifurcated strategy provides a comprehensive perspective on gene interactions.Our methodological advancement allows for a nuanced analysis of gene networks, distinguishing between direct and indirect gene relationships and considering miRNA influences with two available sub-mechanistic submodels. We introduce an approach to validate our findings using a biological interaction network, emphasizing the quality and relevance of identified gene-gene relationships. Metrics like the Matthews Correlation Coefficient (MCC) and the true discovery rate (TDR) further attest to the robustness of our findings.In summation, aside from improving the existing sub-mechanistic model that requires prior knowledge, this paper presents an innovative prior knowledge-free sub-mechanistic model as an alternative. It champions the use of biological networks for validation, underscoring the significance of methodological advancements in genomics research.
更多查看译文
关键词
Hepatocellular carcinoma,graphical models,integrative models,Bayesian variable selection,biological network analysis
AI 理解论文
溯源树
样例
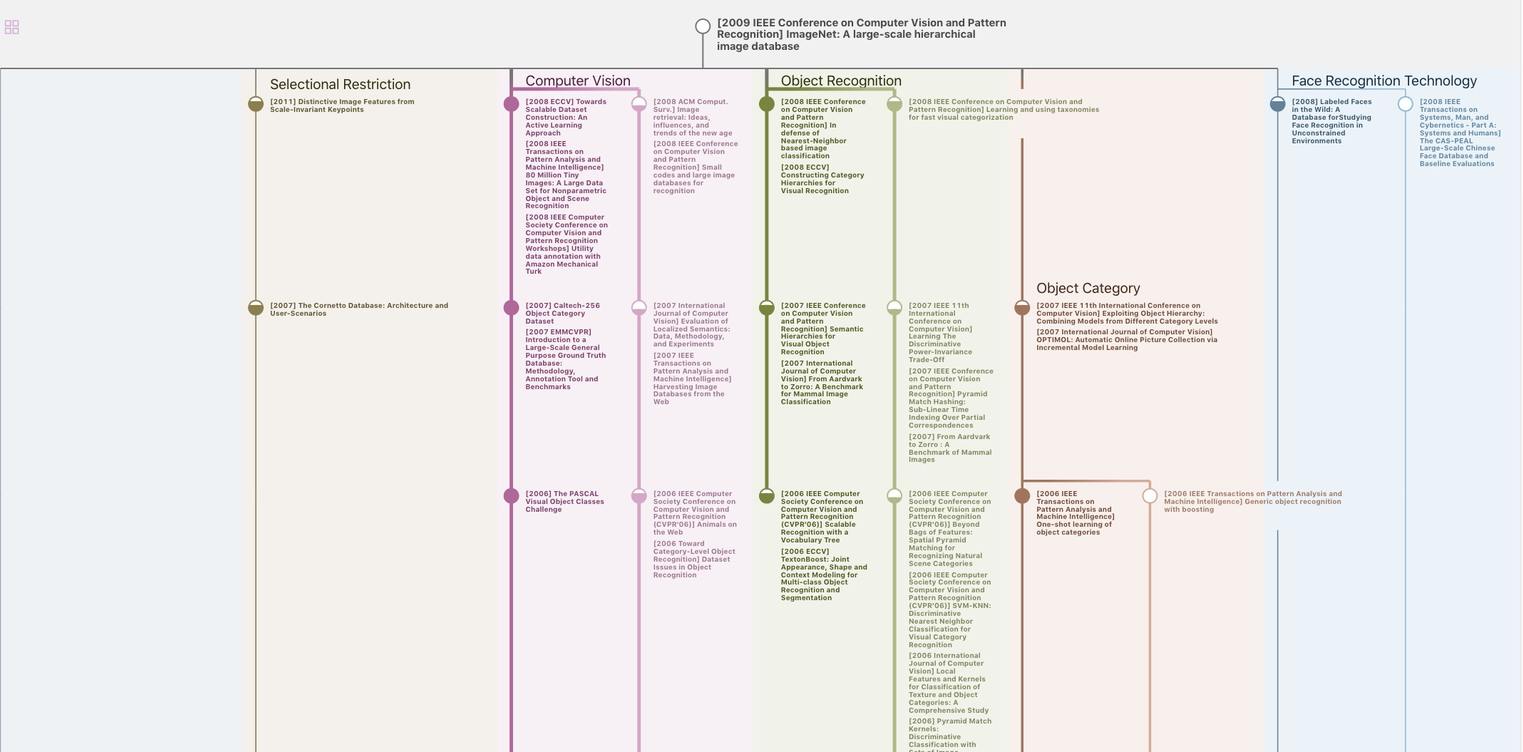
生成溯源树,研究论文发展脉络
Chat Paper
正在生成论文摘要