TetraCVD: A Temporal-Textual Transformer based Model for Cardiovascular Disease Diagnosis.
2023 IEEE International Conference on Bioinformatics and Biomedicine (BIBM)(2023)
摘要
Cardiovascular disease (CVD) is one of the leading causes of death globally. There is considerable clinical significance and an emerging need of assisting doctors to diagnose cardiovascular disease and identify the subtype of it, from which doctors can provide different treatments and medications to increase the cure rate. The goal of this paper is to develop a deep learning model to predict cardiovascular disease and classify its subtype, which by handling data from two modalities of time-series vital signs and text report. We propose a temporal-textual transformer based model for cardiovascular disease diagnosis, TetraCVD, to address the challenges of irregular temporal feature extraction and medical long-text feature extraction respectively. TetraCVD is a multimodal deep learning model, consisting of two networks, cvdGNN and cvdHierBERT, as its time-series and language backbones, which leverage knowledge from temporal vital signs and text reports of the individuals respectively. Our results show that TetraCVD achieves promising performance in predicting subtypes of cardiovascular disease using the P18-ECER dataset and obtains state-of-the-art results. This study is among the first efforts that use both time-series vital signs and text report data to predict cardiovascular disease and its subtype. We argue that our approach can be generalized to predict and diagnose other diseases easily, and it can potentially play a significant role in the domain of general disease diagnosis in the future.
更多查看译文
关键词
Cardiovascular disease,Multimodal fusion,Disease prediction,Vital signs,Text report
AI 理解论文
溯源树
样例
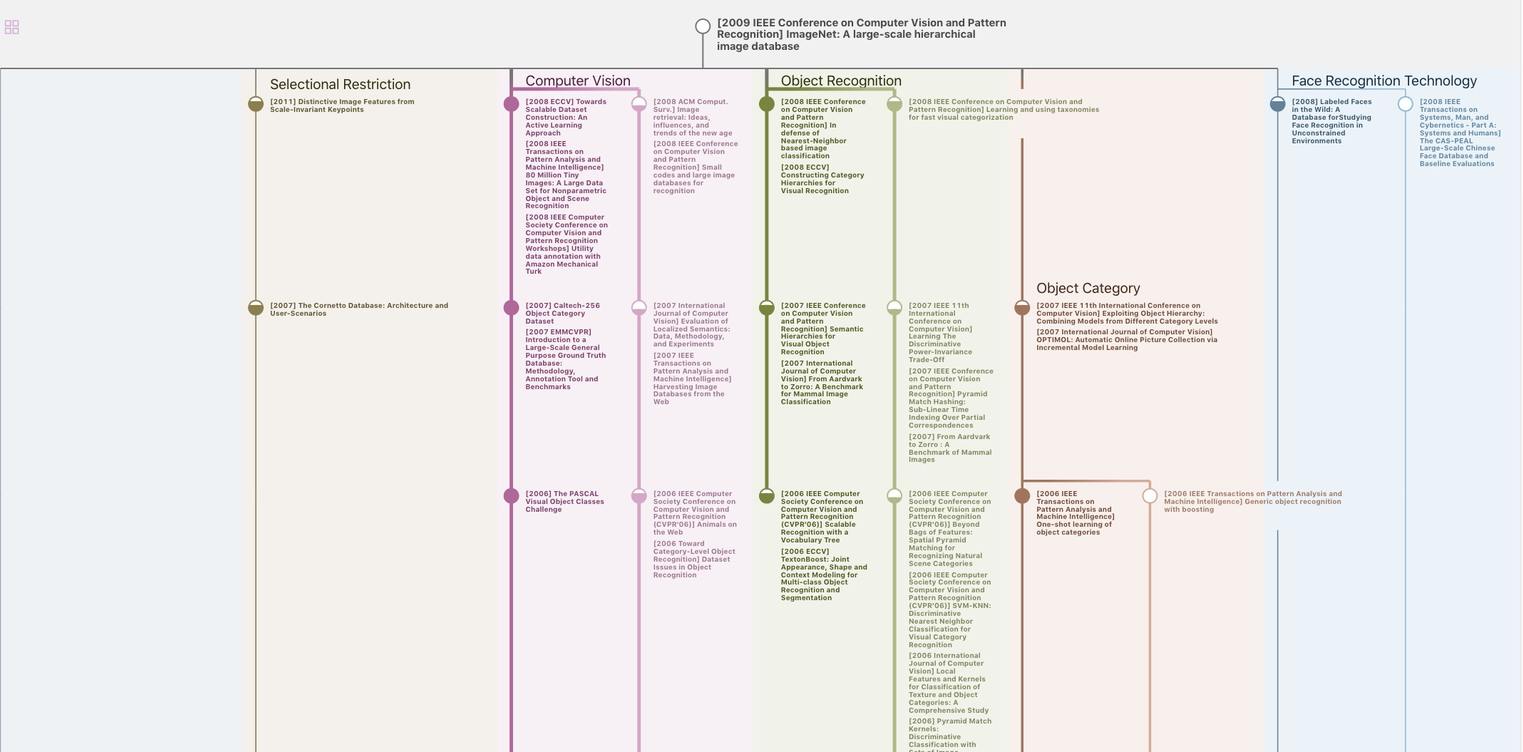
生成溯源树,研究论文发展脉络
Chat Paper
正在生成论文摘要