A Novel Deep Learning Approach Featuring Graph-Based Algorithm for Cell Segmentation and Tracking.
2023 IEEE International Conference on Bioinformatics and Biomedicine (BIBM)(2023)
摘要
The precise segmentation and tracking of cells in microscopy image sequences play a pivotal role in biomedical research, facilitating the study of tissue, organ, and organism development. However, manual segmentation and tracking of cells is time-consuming and often require professional experiences. Besides, segmenting cells in the images with a low signal-to-noise ratio remains difficult. While deep learning (DL) has become a common method for cell segmentation, few DL- based methods address concurrent cell segmentation and tracking. In this paper, we propose a novel DL approach featuring graph-based tracking for cell segmentation and tracking in microscopy images. We combine Deeplabv3+ for semantic segmentation and ResNet50 for enhanced feature extraction, enabling comprehensive cell detection and instance segmentation. Post-processing, involving non-maxima suppression and outlier detection, refines predictions and produces final segmentation. The tracking method is based on the relative position of graph nodes to track segmented cells, encompassing cell division and apoptosis. We conduct our experiments on the induced pluripotent stem (iPS) cell datasets, and the results show that the segmentation and tracking performance of our method yields superior performance compared to the benchmark models. More specifically, our approach achieved DET values of 0.955 and 0.913, TRA values of 0.951 and 0.906, and SEG values of 0.690 and 0.665 on two iPS dataset videos, respectively. Additionally, the performance assessment encompasses four real microscopy datasets from the Cell Tracking Challenge (CTC). Our method greatly reduces the cost of manual labeling and labor-intensive costs.
更多查看译文
关键词
cell tracking,cell segmentation,deep learning,instance segmentation,iPS cell reprogramming,Deeplabv3+
AI 理解论文
溯源树
样例
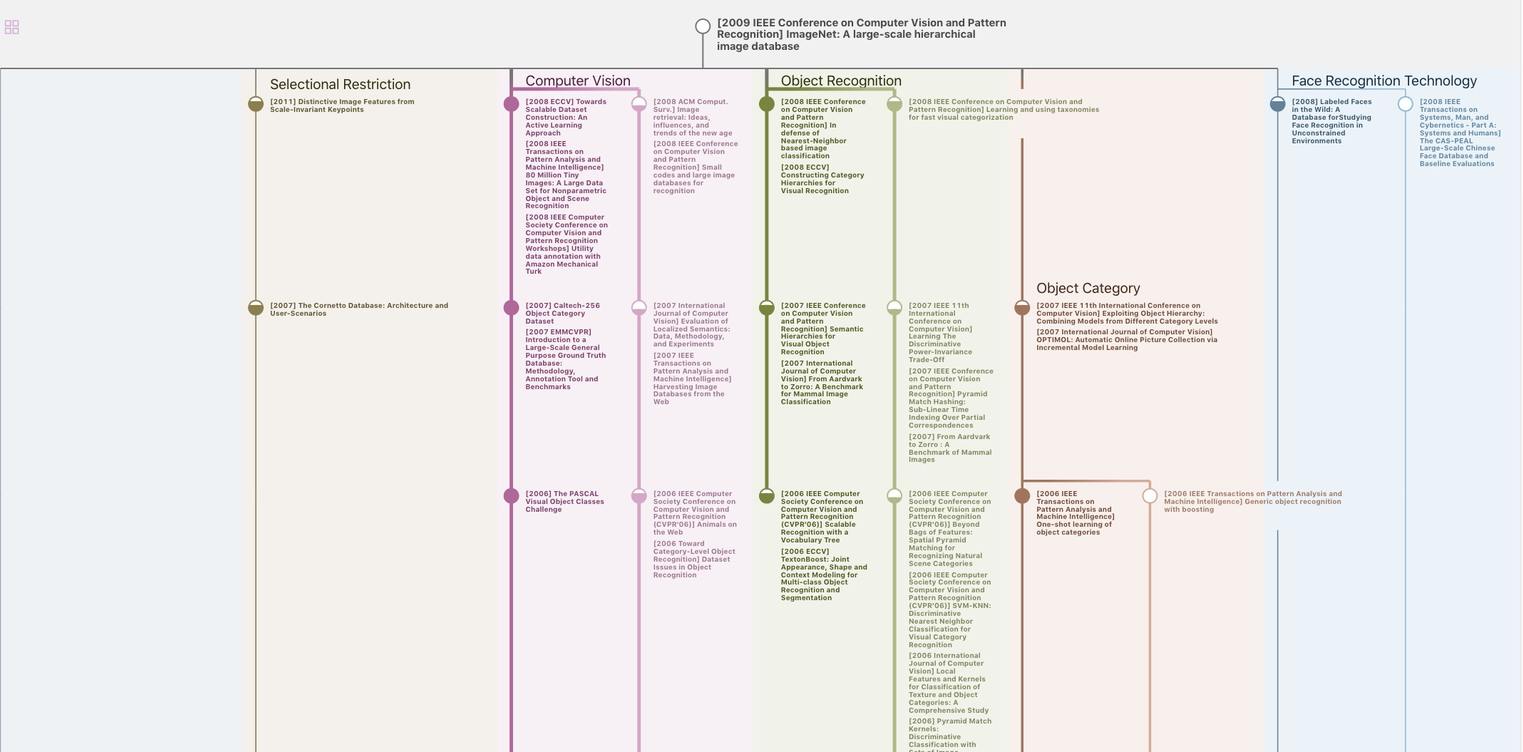
生成溯源树,研究论文发展脉络
Chat Paper
正在生成论文摘要