Towards retraining-free RNA modification prediction with incremental learning
INFORMATION SCIENCES(2024)
摘要
RNA modifications are important for deciphering the function of cells and their regulatory mechanisms. In recent years, researchers have developed many deep learning methods to identify specific modifications. However, these methods require model retraining for each new RNA modification and cannot progressively identify the newly identified RNA modifications. To address this challenge, we propose an innovative incremental learning framework that incorporates multiple incremental learning methods. Our experimental results confirm the efficacy of incremental learning strategies in addressing the RNA modification challenge. By uniquely targeting 10 RNA modification types in a class incremental setting, our framework exhibits superior performance. Notably, it can be extended to new category methylation predictions without the need for retraining with previous data, improving computational efficiency. Through the accumulation of knowledge, the model is able to evolve and continuously learn the differences across methylation, mitigating the problem of catastrophic forgetting during deep learning model training. Overall, our framework provides various alternatives to enhance the prediction of novel RNA modifications and illuminates the potential of incremental learning in tacking numerous genome data.
更多查看译文
关键词
RNA modification,Deep learning,Incremental learning
AI 理解论文
溯源树
样例
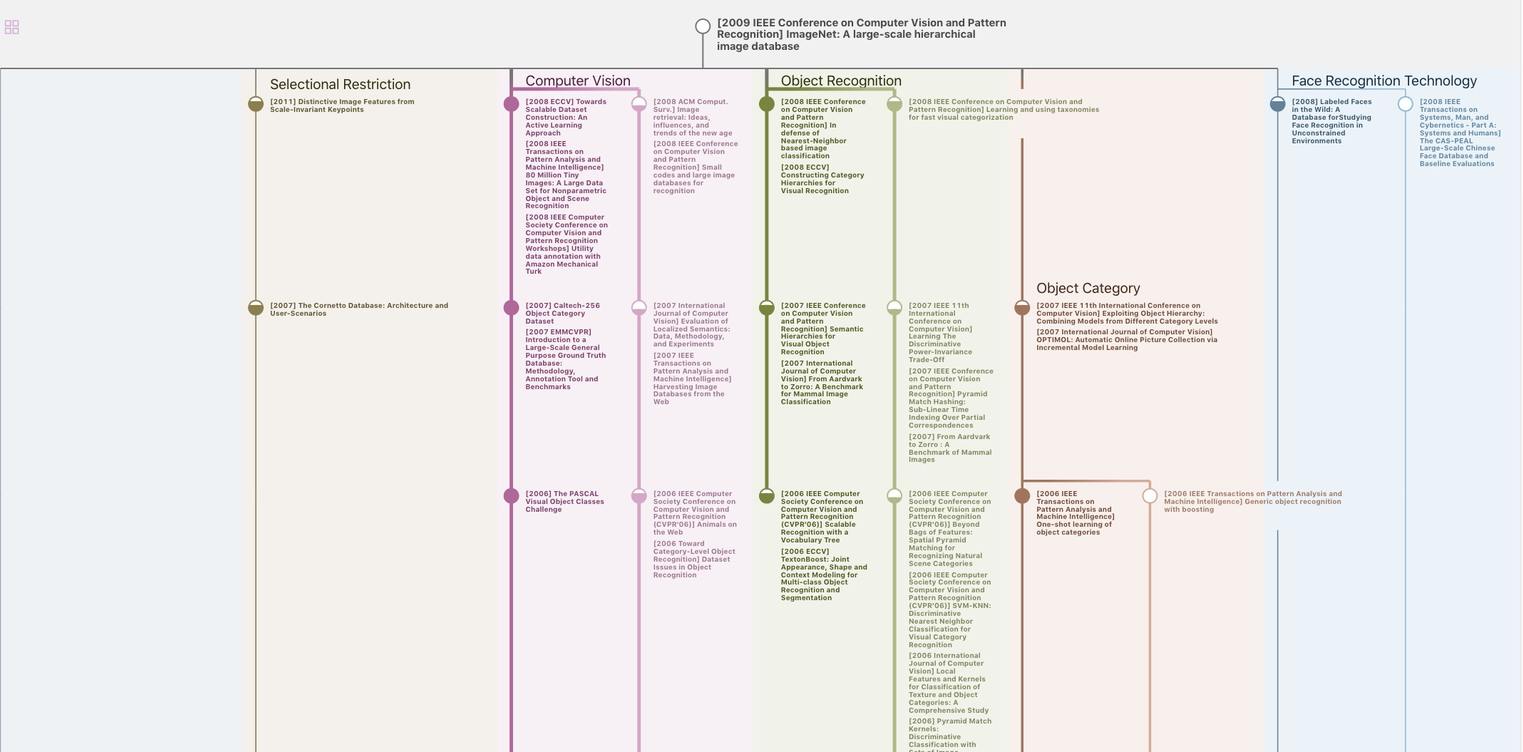
生成溯源树,研究论文发展脉络
Chat Paper
正在生成论文摘要