Deep Learning-Based Prediction and Revenue Optimization for Online Platform User Journeys
QUANTITATIVE FINANCE AND ECONOMICS(2024)
摘要
In today's digital landscape, businesses must allocate online resources efficiently. Data-driven AI methods are increasingly adopted for customer journey management. This study enhances existing frameworks with three key propositions, integrating deep learning and optimization to create a three-step revenue optimization model using online customer data. First, we apply K-means clustering to analyze online user data, constructing a behavior model. Then, convolutional neural networks (CNN) and long short-term memory (LSTM) networks predict user behavior and conversion values from sequential data. Finally, the heuristic algorithm optimizes revenue within budget constraints based on conversions. From an academic perspective, our study provides an empirical, theory-grounded model for service and marketing management. Technologically, we identify three key findings: stacking LSTM with CNN effectively processes sequential online user data, outperforming traditional machine learning methods; optimization methods and decision trees improve model interpretability and address marketing attribution challenges by understanding user behavior and channel impacts; and traditional integer programming models fall short in solving high-dimensional online channel planning problems, necessitating heuristic algorithms. Our model aids companies in setting online channel standards and budgets, offering valuable insights and practical guidance to decision-makers.
更多查看译文
关键词
customer journeys managements,e-commerce platform,deep learning,optimization methods
AI 理解论文
溯源树
样例
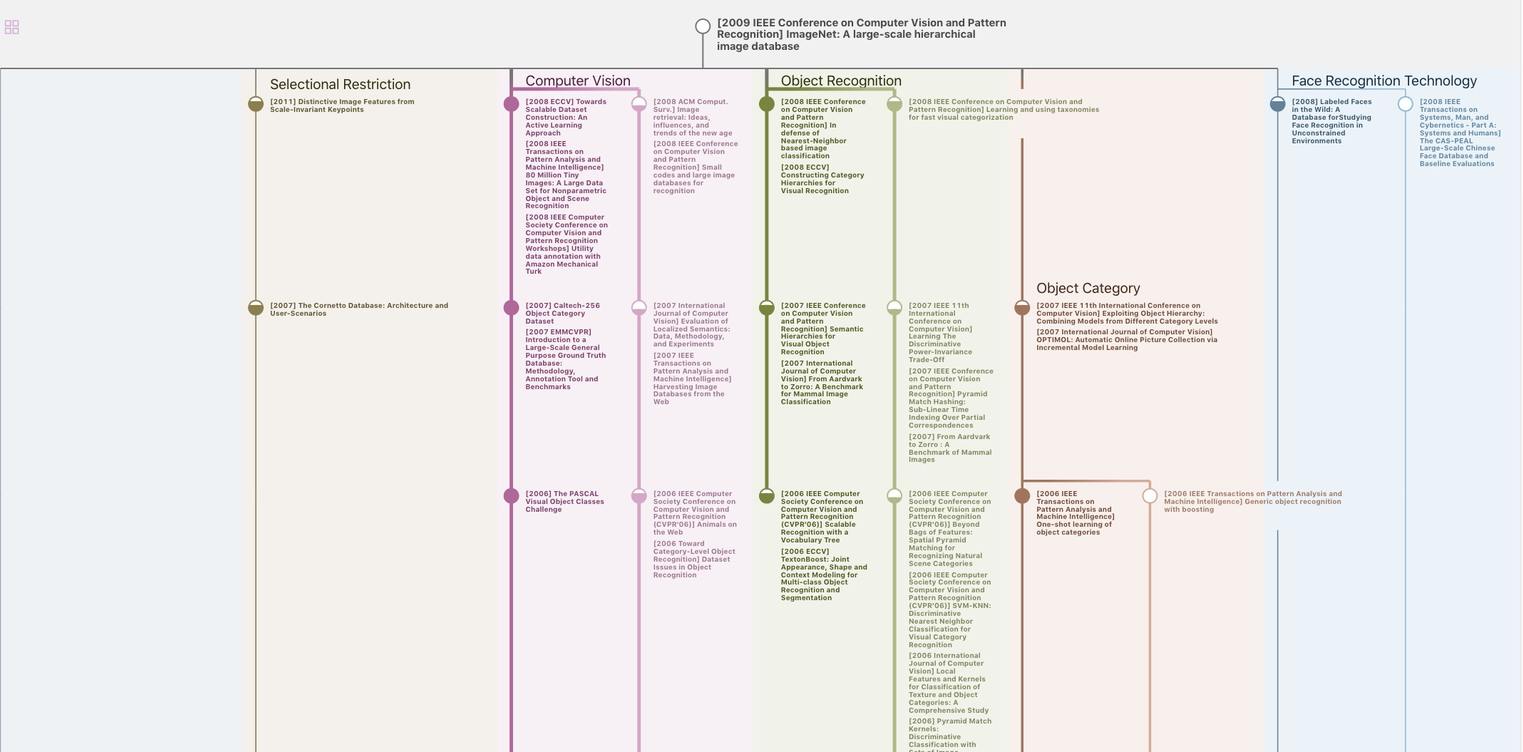
生成溯源树,研究论文发展脉络
Chat Paper
正在生成论文摘要