Communication-Efficient Personalized Federated Learning for Speech-to-Text Tasks
CoRR(2024)
摘要
To protect privacy and meet legal regulations, federated learning (FL) has
gained significant attention for training speech-to-text (S2T) systems,
including automatic speech recognition (ASR) and speech translation (ST).
However, the commonly used FL approach (i.e., FedAvg) in S2T tasks
typically suffers from extensive communication overhead due to multi-round
interactions based on the whole model and performance degradation caused by
data heterogeneity among clients.To address these issues, we propose a
personalized federated S2T framework that introduces FedLoRA, a
lightweight LoRA module for client-side tuning and interaction with the server
to minimize communication overhead, and FedMem, a global model
equipped with a k-nearest-neighbor (kNN) classifier that captures
client-specific distributional shifts to achieve personalization and overcome
data heterogeneity. Extensive experiments based on Conformer and Whisper
backbone models on CoVoST and GigaSpeech benchmarks show that our approach
significantly reduces the communication overhead on all S2T tasks and
effectively personalizes the global model to overcome data heterogeneity.
更多查看译文
关键词
Federated learning,speech-to-text,personalization,memorization retrieval,LoRA
AI 理解论文
溯源树
样例
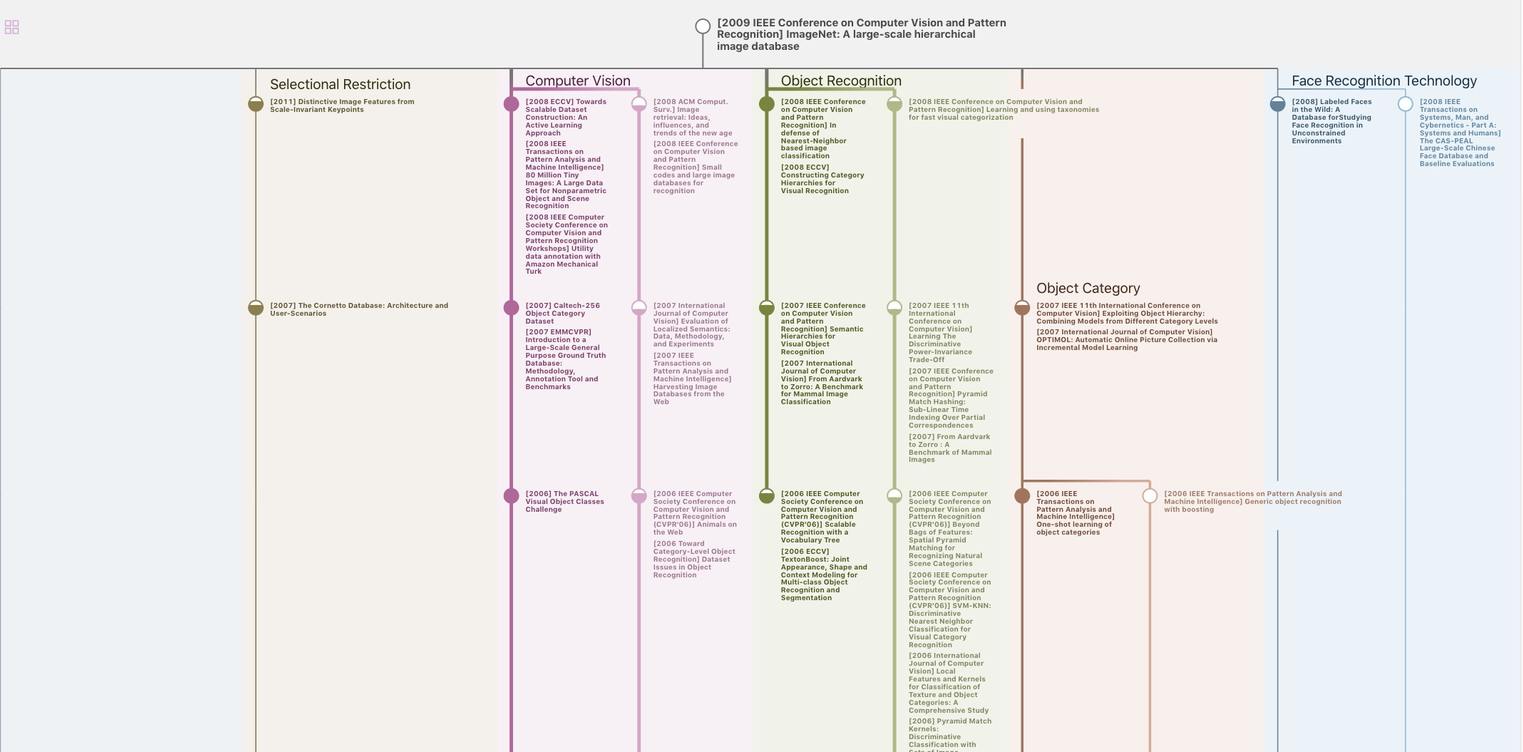
生成溯源树,研究论文发展脉络
Chat Paper
正在生成论文摘要