FLex Chill: Improving Local Federated Learning Training with Logit Chilling
CoRR(2024)
摘要
Federated learning are inherently hampered by data heterogeneity: non-iid
distributed training data over local clients. We propose a novel model training
approach for federated learning, FLex Chill, which exploits the Logit Chilling
method. Through extensive evaluations, we demonstrate that, in the presence of
non-iid data characteristics inherent in federated learning systems, this
approach can expedite model convergence and improve inference accuracy.
Quantitatively, from our experiments, we observe up to 6X improvement in the
global federated learning model convergence time, and up to 3.37
in inference accuracy.
更多查看译文
AI 理解论文
溯源树
样例
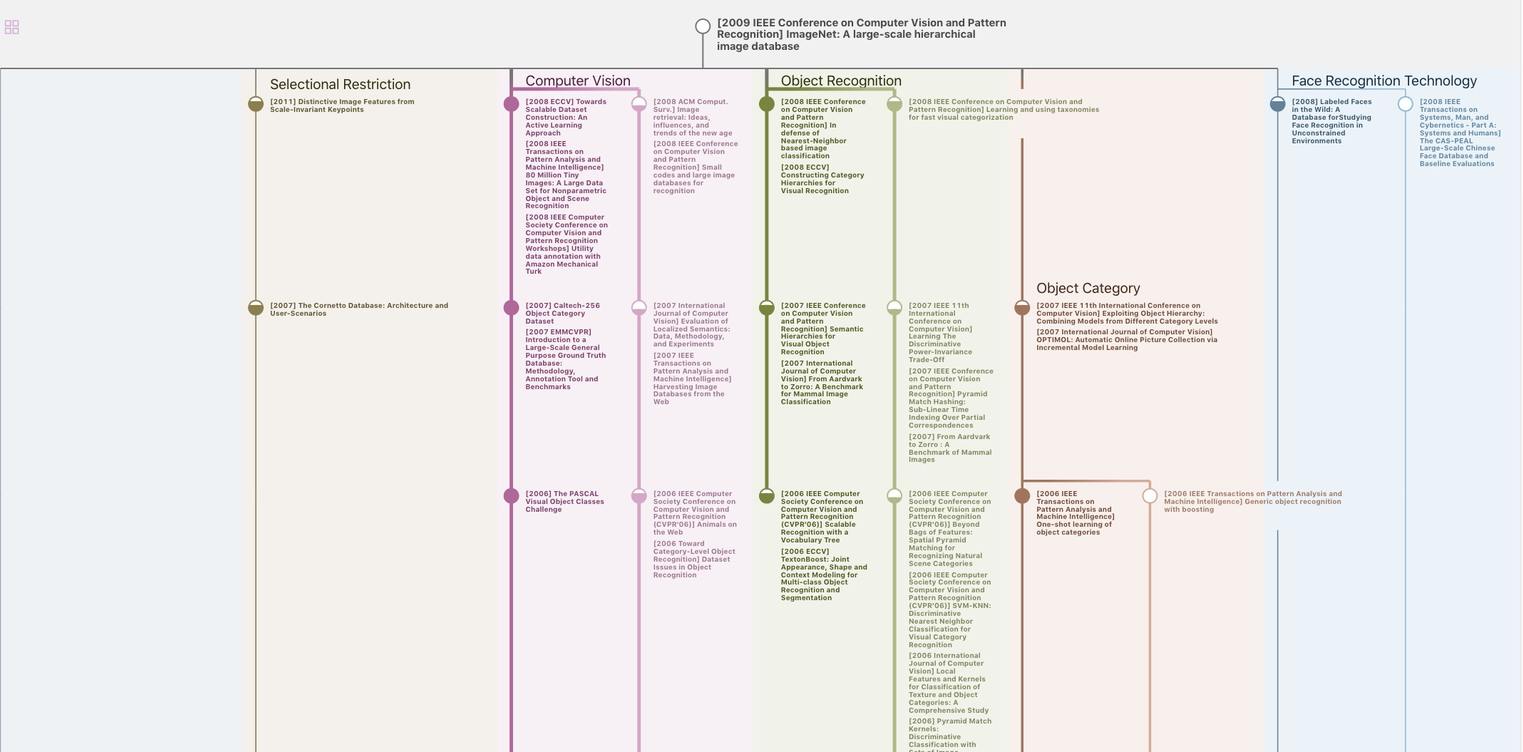
生成溯源树,研究论文发展脉络
Chat Paper
正在生成论文摘要