Material-Response-Informed DeepONet and its Application to Polycrystal Stress-strain Prediction in Crystal Plasticity
CoRR(2024)
摘要
Crystal plasticity (CP) simulations are a tool for understanding how
microstructure morphology and texture affect mechanical properties and are an
essential component of elucidating the structure-property relations. However,
it can be computationally expensive. Hence, data-driven machine learning models
have been applied to predict the mean-field response of a polycrystal
representative volume element to reduce computation time. In this work, we
proposed a novel Deep Operator Network (DeepONet) architecture for predicting
microstructure stress-strain response. It employs a convolutional neural
network in the trunk to encode the microstructure. To account for different
material properties, boundary conditions, and loading, we proposed using single
crystal stress-strain curves as inputs to the branch network, furnishing a
material-response-informed DeepONet. Using four numerical examples, we
demonstrate that the current DeepONet can be trained on a single material and
loading and then generalized to new conditions via transfer learning. Results
show that using single crystal responses as input outperforms a similar model
using material properties as inputs and overcomes limitations with changing
boundary conditions and temporal resolution. In all cases, the new model
achieved a R^2 value of above 0.99, and over 95% of predicted stresses have
a relative error of ≤ 5%, indicating superior accuracy. With as few as 20
new data points and under 1min training time, the trained DeepONet can be
fine-tuned to generate accurate predictions on different materials and loading.
Once trained, the prediction speed is almost 1×10^4 times faster the
CP simulations. The efficiency and high generalizability of our DeepONet render
it a powerful data-driven surrogate model for CP simulations in multi-scale
analyses.
更多查看译文
AI 理解论文
溯源树
样例
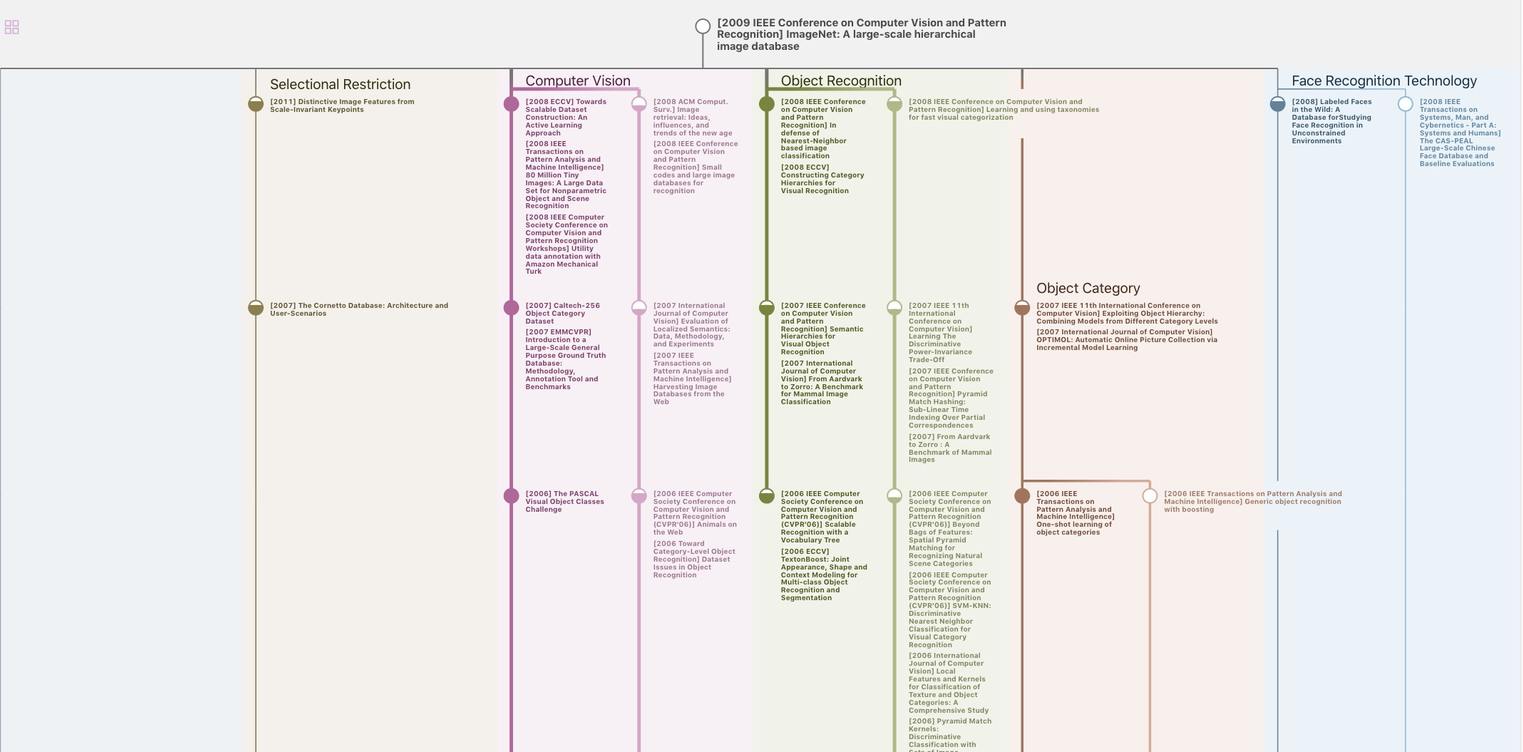
生成溯源树,研究论文发展脉络
Chat Paper
正在生成论文摘要