Improving Speaker-independent Speech Emotion Recognition Using Dynamic Joint Distribution Adaptation
CoRR(2024)
摘要
In speaker-independent speech emotion recognition, the training and testing
samples are collected from diverse speakers, leading to a multi-domain shift
challenge across the feature distributions of data from different speakers.
Consequently, when the trained model is confronted with data from new speakers,
its performance tends to degrade. To address the issue, we propose a Dynamic
Joint Distribution Adaptation (DJDA) method under the framework of multi-source
domain adaptation. DJDA firstly utilizes joint distribution adaptation (JDA),
involving marginal distribution adaptation (MDA) and conditional distribution
adaptation (CDA), to more precisely measure the multi-domain distribution
shifts caused by different speakers. This helps eliminate speaker bias in
emotion features, allowing for learning discriminative and speaker-invariant
speech emotion features from coarse-level to fine-level. Furthermore, we
quantify the adaptation contributions of MDA and CDA within JDA by using a
dynamic balance factor based on 𝒜-Distance, promoting to
effectively handle the unknown distributions encountered in data from new
speakers. Experimental results demonstrate the superior performance of our DJDA
as compared to other state-of-the-art (SOTA) methods.
更多查看译文
关键词
speaker-independent,speech emotion recognition,multi-source domain adaptation,joint distribution adaptation
AI 理解论文
溯源树
样例
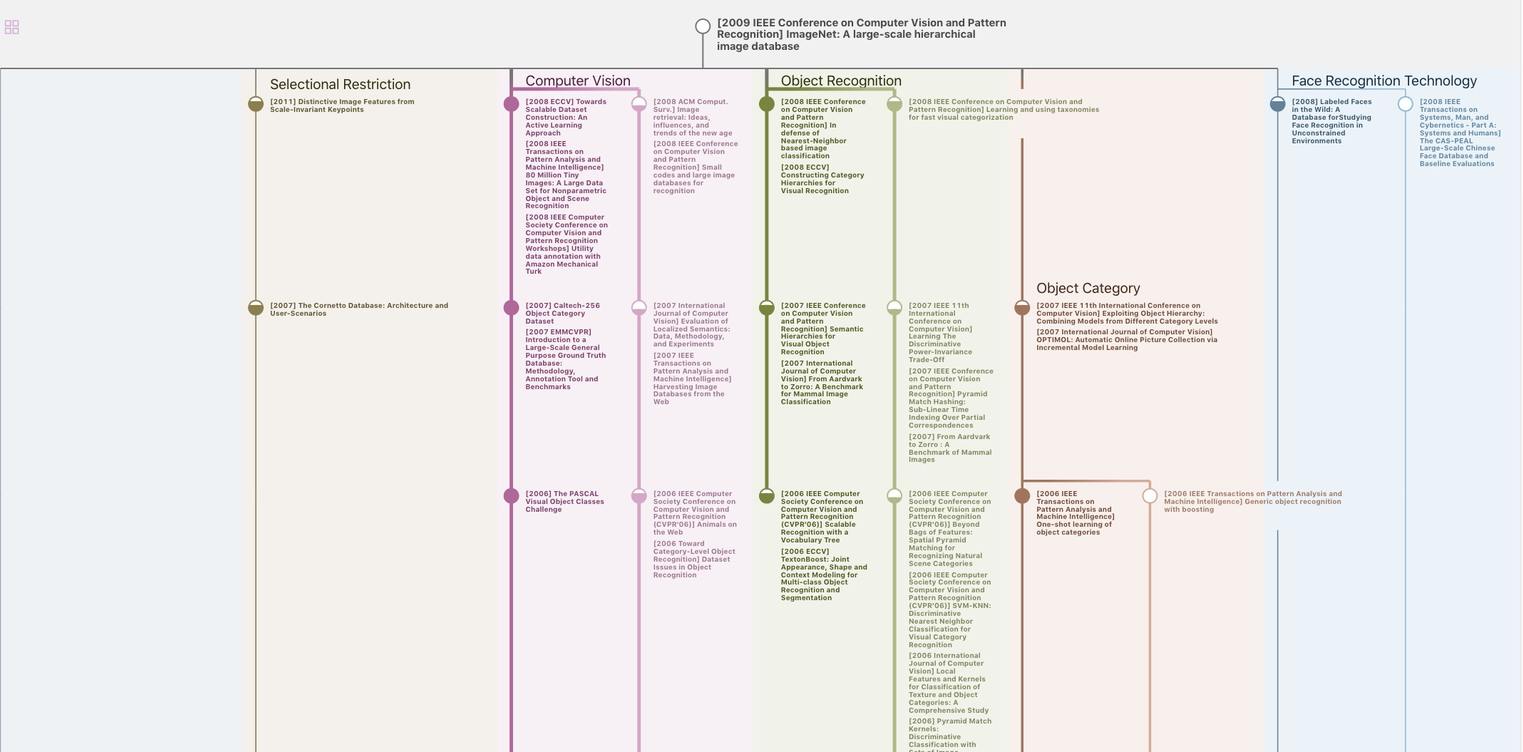
生成溯源树,研究论文发展脉络
Chat Paper
正在生成论文摘要