On a meta-learning population-based approach to damage prognosis
MECHANICAL SYSTEMS AND SIGNAL PROCESSING(2024)
摘要
The current work studies the application of population -based structural health monitoring (PBSHM) to the problem of damage prognosis. Two methods are proposed for populationinformed damage prognosis and they are evaluated according to their performance using an experimental dataset. The first method is an attempt to define a functional subspace, which includes the potential behaviour of members of the population subjected to the phenomenon of damage evolution. The second approach is a meta -learning method, the deep kernel transfer (DKT) method, which seeks to exploit information from a population in order to enhance the predictive performance of a Gaussian process. The predictive capabilities of the two methods are tested in an experimental crack -growth problem. The results reveal that the two methods are properly informed by the population to make predictions about new structures and show potential in dealing with the problem of damage evolution, which is a problem of imbalanced and difficult -to -acquire data.
更多查看译文
关键词
Population-based structural health monitoring (PBSHM),Damage prognosis,Machine learning,Meta-learning
AI 理解论文
溯源树
样例
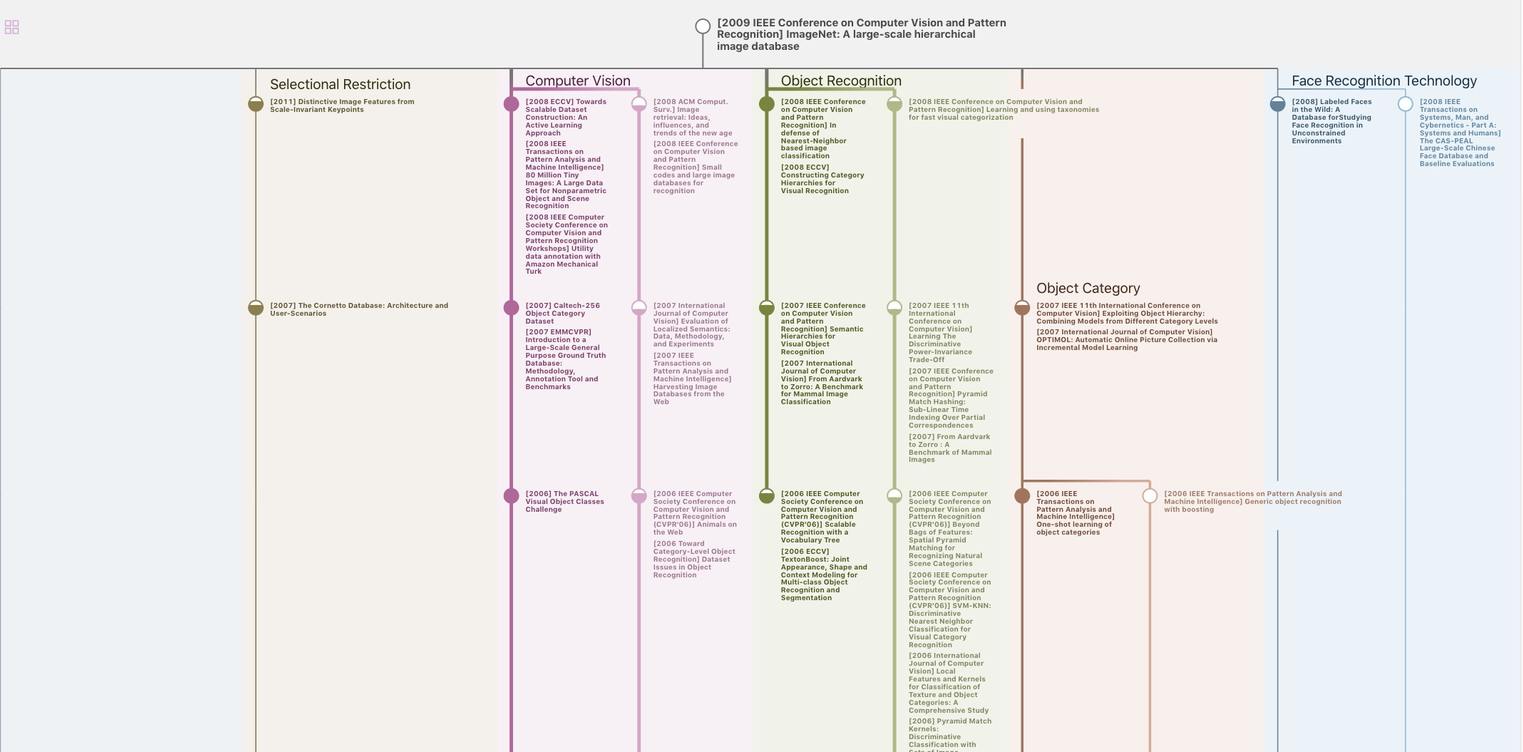
生成溯源树,研究论文发展脉络
Chat Paper
正在生成论文摘要