A deep learning-based Monte Carlo simulation scheme for stochastic differential equations driven by fractional Brownian motion
NEUROCOMPUTING(2024)
摘要
Stochastic differential equations (SDEs) are widely used models to describe the evolution of stochastic processes. Among them, SDEs driven by fractional Brownian motion (fBm) have been shown to be capable of describing systems with temporal dependencies. In this paper, we develop a neural network based Monte Carlo methodology in which we can efficiently simulate SDEs that are governed by fBm. Particularly, we focus on large time step simulations. A property of fBm that complicates the development of such Monte Carlo schemes is the long-range temporal correlation. To this end, we build the network based on the encoder-decoder framework and employ the attention mechanism to learn the temporal relationships in the historical paths of such SDEs. In addition, a loss function based on the quantile loss is used, where the quantile levels to be predicted are determined by means of the stochastic collocation method. Experimental results show that this kind of loss function is superior to conventional loss functions in terms of solution accuracy, and the resulting scheme can learn and simulate SDEs driven by fBm accurately and highly efficiently.
更多查看译文
关键词
Deep learning,Stochastic differential equations,Fractional Brownian motion,Fractional Ornstein-Uhlenbeck process,Numerical simulation,Option pricing
AI 理解论文
溯源树
样例
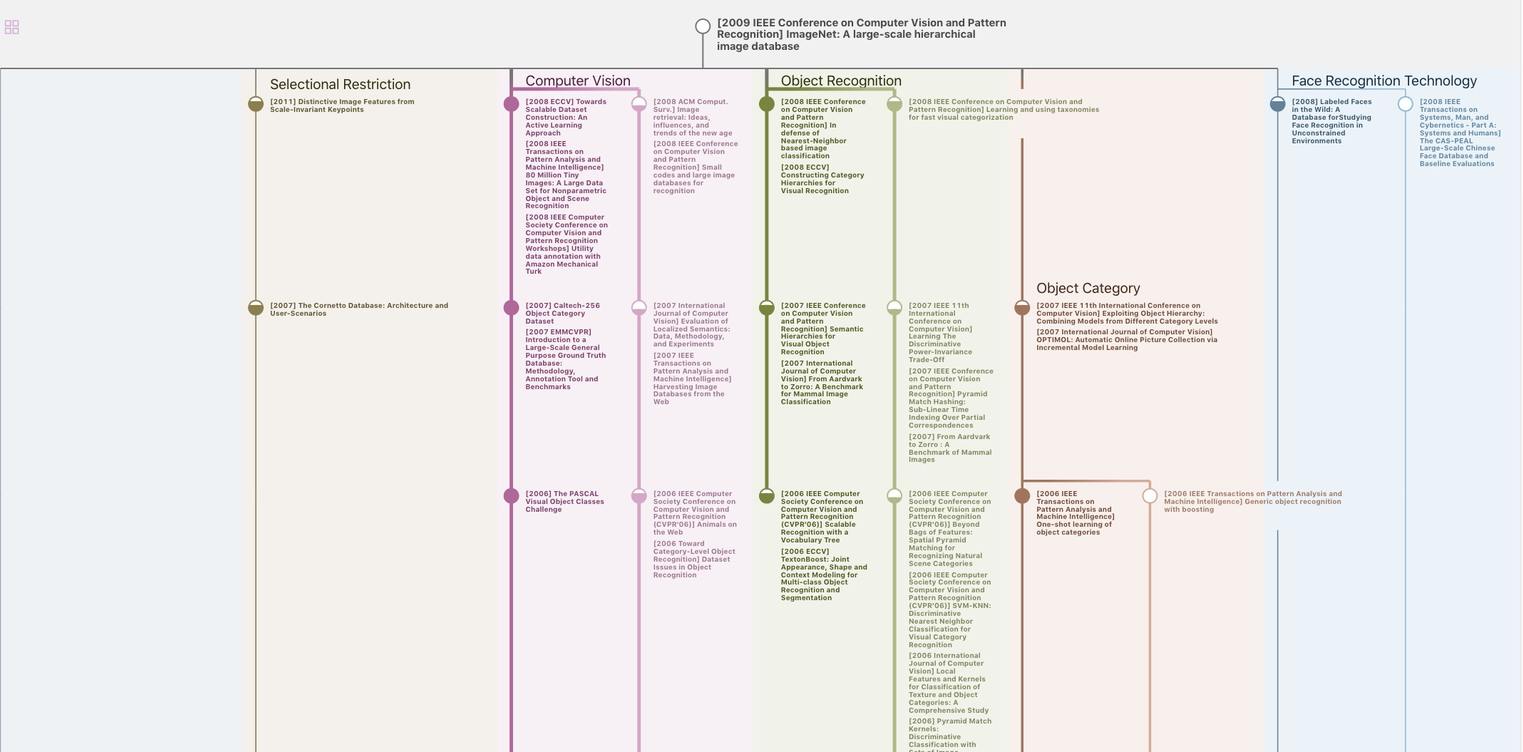
生成溯源树,研究论文发展脉络
Chat Paper
正在生成论文摘要