Multi-center application of a convolutional neural network for preoperative detection of cavernous sinus invasion in pituitary adenomas
Neuroradiology(2024)
摘要
Objective Cavernous sinus invasion (CSI) plays a pivotal role in determining management in pituitary adenomas. The study aimed to develop a Convolutional Neural Network (CNN) model to diagnose CSI in multiple centers. Methods A total of 729 cases were retrospectively obtained in five medical centers with (n = 543) or without CSI (n = 186) from January 2011 to December 2021. The CNN model was trained using T1-enhanced MRI from two pituitary centers of excellence (n = 647). The other three municipal centers (n = 82) as the external testing set were imported to evaluate the model performance. The area-under-the-receiver-operating-characteristic-curve values (AUC-ROC) analyses were employed to evaluate predicted performance. Gradient-weighted class activation mapping (Grad-CAM) was used to determine models' regions of interest. Results The CNN model achieved high diagnostic accuracy (0.89) in identifying CSI in the external testing set, with an AUC-ROC value of 0.92 (95% CI, 0.88–0.97), better than CSI clinical predictor of diameter (AUC-ROC: 0.75), length (AUC-ROC: 0.80), and the three kinds of dichotomizations of the Knosp grading system (AUC-ROC: 0.70–0.82). In cases with Knosp grade 3A (n = 24, CSI rate, 0.35), the accuracy the model accounted for 0.78, with sensitivity and specificity values of 0.72 and 0.78, respectively. According to the Grad-CAM results, the views of the model were confirmed around the sellar region with CSI. Conclusions The deep learning model is capable of accurately identifying CSI and satisfactorily able to localize CSI in multicenters.
更多查看译文
关键词
Pituitary Adenoma,Deep Learning,Cavernous sinus,Invasion
AI 理解论文
溯源树
样例
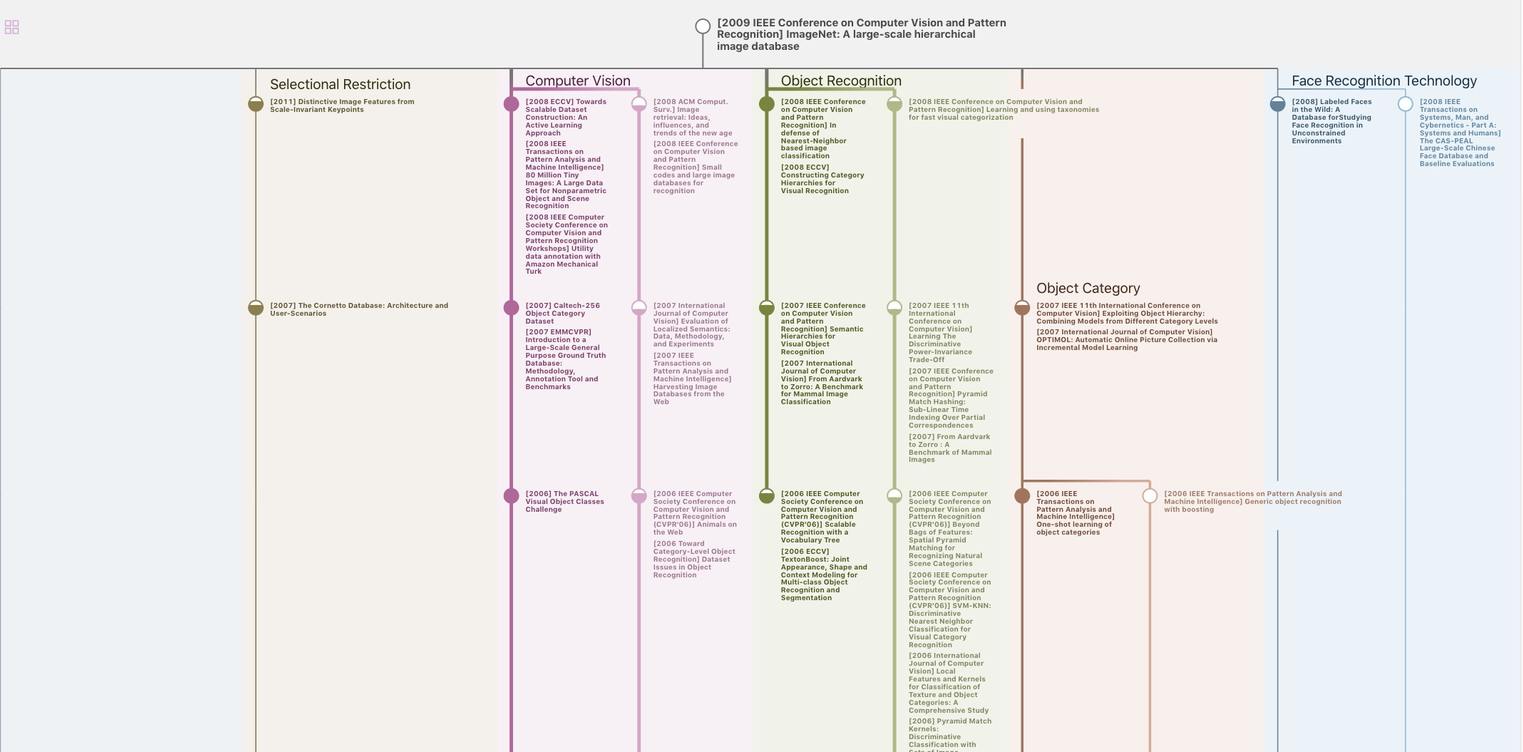
生成溯源树,研究论文发展脉络
Chat Paper
正在生成论文摘要