Exploring Model Compression Limits and Laws: A Pyramid Knowledge Distillation Framework for Satellite-on-Orbit Object Recognition
IEEE TRANSACTIONS ON GEOSCIENCE AND REMOTE SENSING(2024)
摘要
Extremely constrained storage and computational resources are one of the difficulties of satellite-on-orbit computing, which leads to over-parametric high-performance models not performing properly on-orbit. Knowledge distillation (KD) is an effective method for model compression; yet, there is a gap in the study of the limits and laws of KD-based model compression. To bridge this gap, we propose a novel KD framework, pyramid KD (PKD) and define a knowledge explosion and knowledge offset. Specifically, the pyramid distillation framework is built by stacking multiple sets of deep mutual learning (DML) models, with the smaller models on the top of the larger ones, and the overall structure is like a pyramid; hence, it is called PKD. To avoid knowledge explosion, we design a hybrid online-offline smooth distillation (HOSD) strategy by combining online distillation and offline distillation and reducing the difference between models. To avoid knowledge offset, we design an adaptive multiteacher distillation method to obtain multiteacher weighted knowledge by adaptively learning the weight of each teacher's knowledge. We introduce an evolutionary algorithm to automatically find the optimal PKD configuration. We conduct ablation experiments and compare PKD with state-of-the-art distillation methods using ResNet series networks and VGG series networks as base models on Aircraft and FGSC-23 datasets, respectively. The experimental results show the effectiveness and advancement of PKD and reveal the law that the object recognition accuracy varies with the model compression rate.
更多查看译文
关键词
Computational modeling,Adaptation models,Training,Satellites,Feature extraction,Stacking,Remote sensing,Knowledge distillation (KD),model compression,object recognition,remote sensing image,satellite-on-orbit computing
AI 理解论文
溯源树
样例
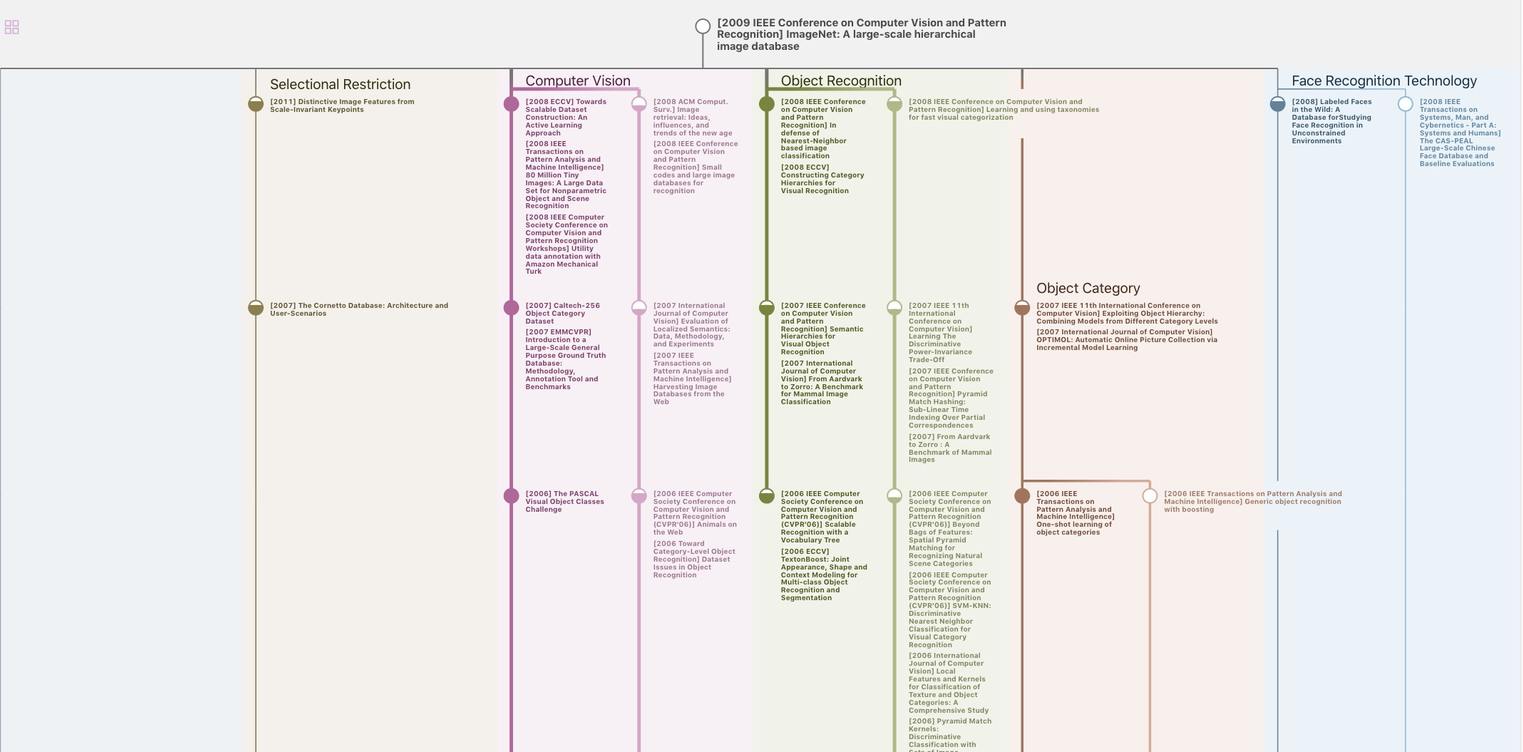
生成溯源树,研究论文发展脉络
Chat Paper
正在生成论文摘要