Auditing Machine Behaviors: Does a diverse crowd result in different social norms?
2023 10th International Conference on Behavioural and Social Computing (BESC)(2023)
摘要
Many intelligent systems depend on black-box services for achieving goals. For example, Decision Support Systems (DSS) incorporate image tagging services for translating the content of an image into useful information that serves the purpose of the system under development (e.g., facilitating hiring decisions). Auditing the results of DSS for fairness can thus become a cumbersome process as they are correlated with the output of the black-box image tagger. In this work, we assume that tags generated by an image tagger are part of the DSS and in terms of fairness can be considered socially acceptable or unacceptable conforming to the varying goals of a DSS. For example, the tag "good-looking" can be considered appropriate in a dating context but inappropriate in a hiring context. We propose a methodology for leveraging the use of crowdsourcing as an approach for auditing what is socially acceptable and unacceptable for tags generated by black-box image taggers. As a first step, we attempt to understand how the crowdworkers perceive (un)acceptable tags in two scenarios: (i) a hiring DSS, and (ii) a dating DSS. Through our analysis, we investigate how answers (in our case tags) received by crowdworkers representing different demographic groups (i.e., country of residence and gender) impact the auditing process. Results indicate that varying the DSS scenario, and the demographics of the crowdworkers, yields a different fairness perspective concurrent to the "acceptable" and "unacceptable" social norms.
更多查看译文
关键词
AI auditing,crowdsourcing,diversity,images
AI 理解论文
溯源树
样例
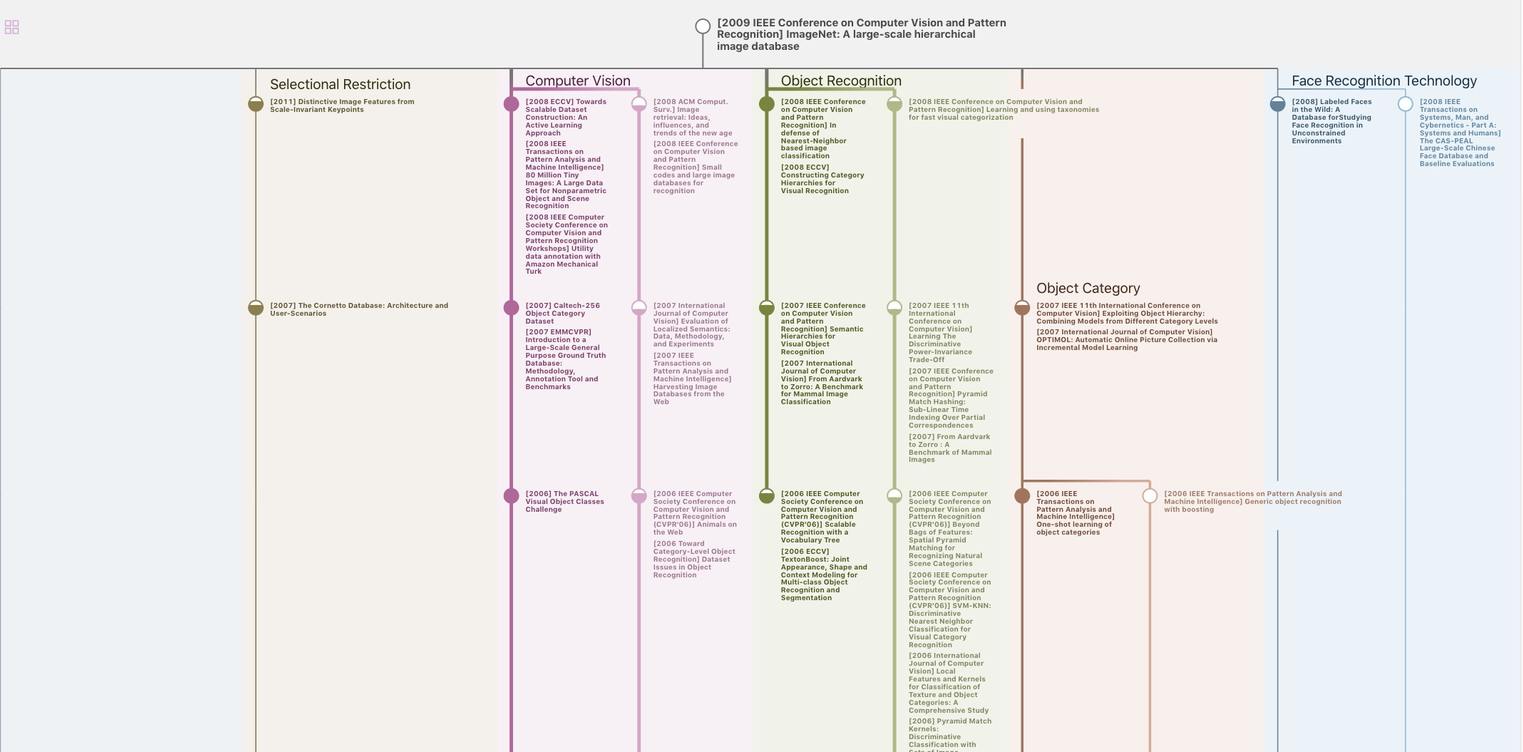
生成溯源树,研究论文发展脉络
Chat Paper
正在生成论文摘要