Enhancing Deep Reinforcement Learning with Compressed Sensing-based State Estimation
2023 IEEE 16TH INTERNATIONAL SYMPOSIUM ON EMBEDDED MULTICORE/MANY-CORE SYSTEMS-ON-CHIP, MCSOC(2023)
摘要
In various real-world applications, sensor data collected for adaptive control using Reinforcement Learning (RL) often suffer from missing information due to sensor failures, data transmission errors, or other sources of noise. Such missing data can significantly hinder the agent's ability to make informed decisions and degrade performance. In this paper, we propose a novel approach to address this challenge by leveraging Compressed Sensing (CS) techniques to recover missing information from the sensor data and reconstruct the state observation. The reconstructed state is then fed to the RL agents. As a result, they exhibit enhanced robustness and intelligence, surpassing the performance achievable when solely presented with noisy data as state input.
更多查看译文
关键词
Reinforcement Learning,Compressed Sensing,Compressive Sensing,Deep Reinforcement Learning
AI 理解论文
溯源树
样例
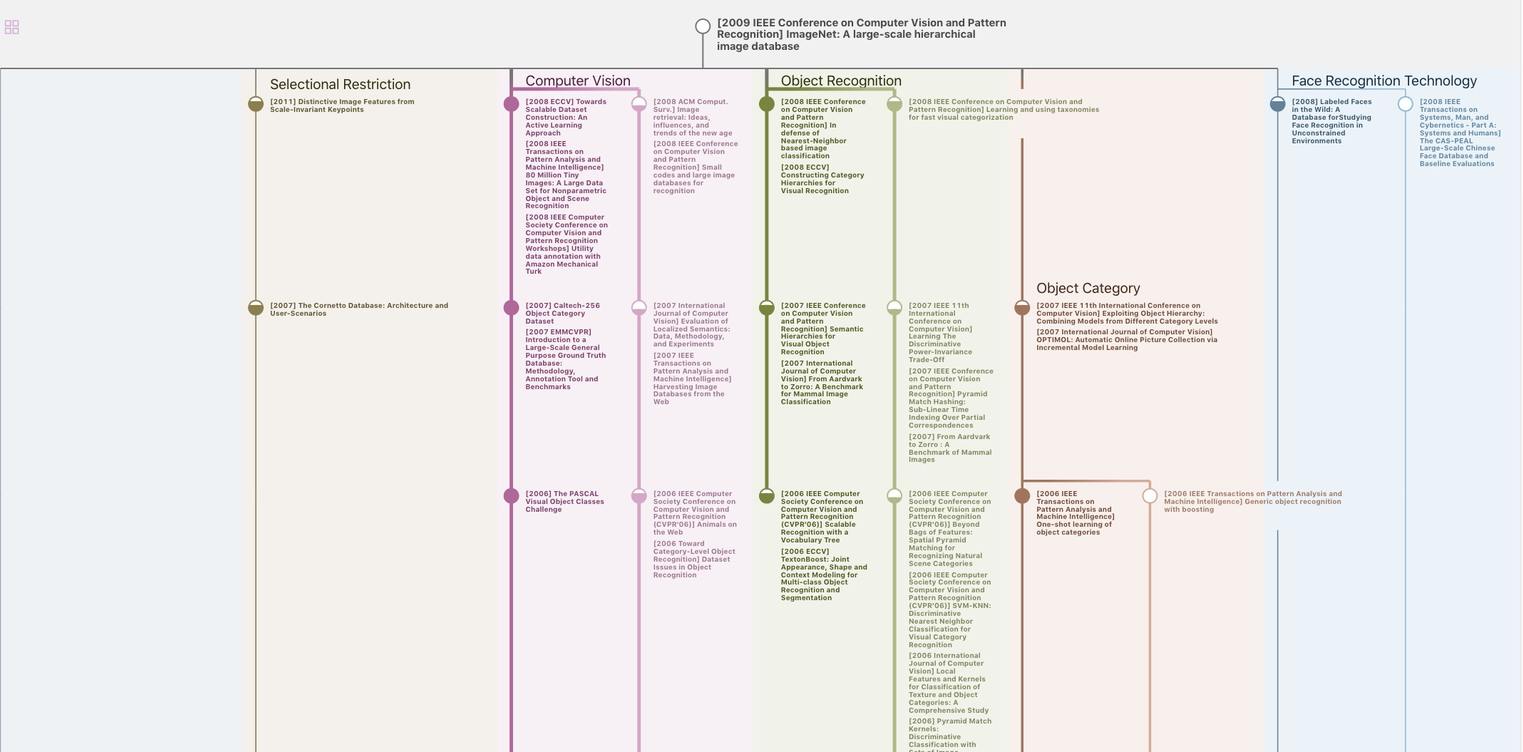
生成溯源树,研究论文发展脉络
Chat Paper
正在生成论文摘要