Improving Object Detection From Remote Sensing Images via Self-Supervised Adaptive Fusion Networks
IEEE GEOSCIENCE AND REMOTE SENSING LETTERS(2024)
摘要
Detecting objects in remote sensing images is essential for intelligent interpretation. Although deep neural networks have made significant progress in recent years, they often struggle with complex backgrounds in remote sensing images, which can lead to inaccurate detection. To tackle this problem, a self-supervised adaptive fusion network (SSAFN) has been developed. The SSAFN includes an adaptive fusion module (AFM) and a self-supervised task module (SSTM). The AFM mainly fuses the deep semantic information to the shallow features with appropriate weights to enhance the semantic information of the shallow features. The SSTM is mainly to constrain the AFM through self-supervised tasks to fulfill the function similar to the attention mechanism: to make the AFM enhance the target feature representation and suppress the background information. The SSAFN reduces the impact of complex backgrounds on object representation, resulting in better detection results for various types of objects such as buildings, ships, and more. The proposed method has been tested on various datasets and has not only improved the detection accuracy for different types of objects but also enhanced the performance of popular object detection algorithms.
更多查看译文
关键词
Adaptive fusion module (AFM),object detec- tion,remote sensing image,self-supervised task module (SSTM)
AI 理解论文
溯源树
样例
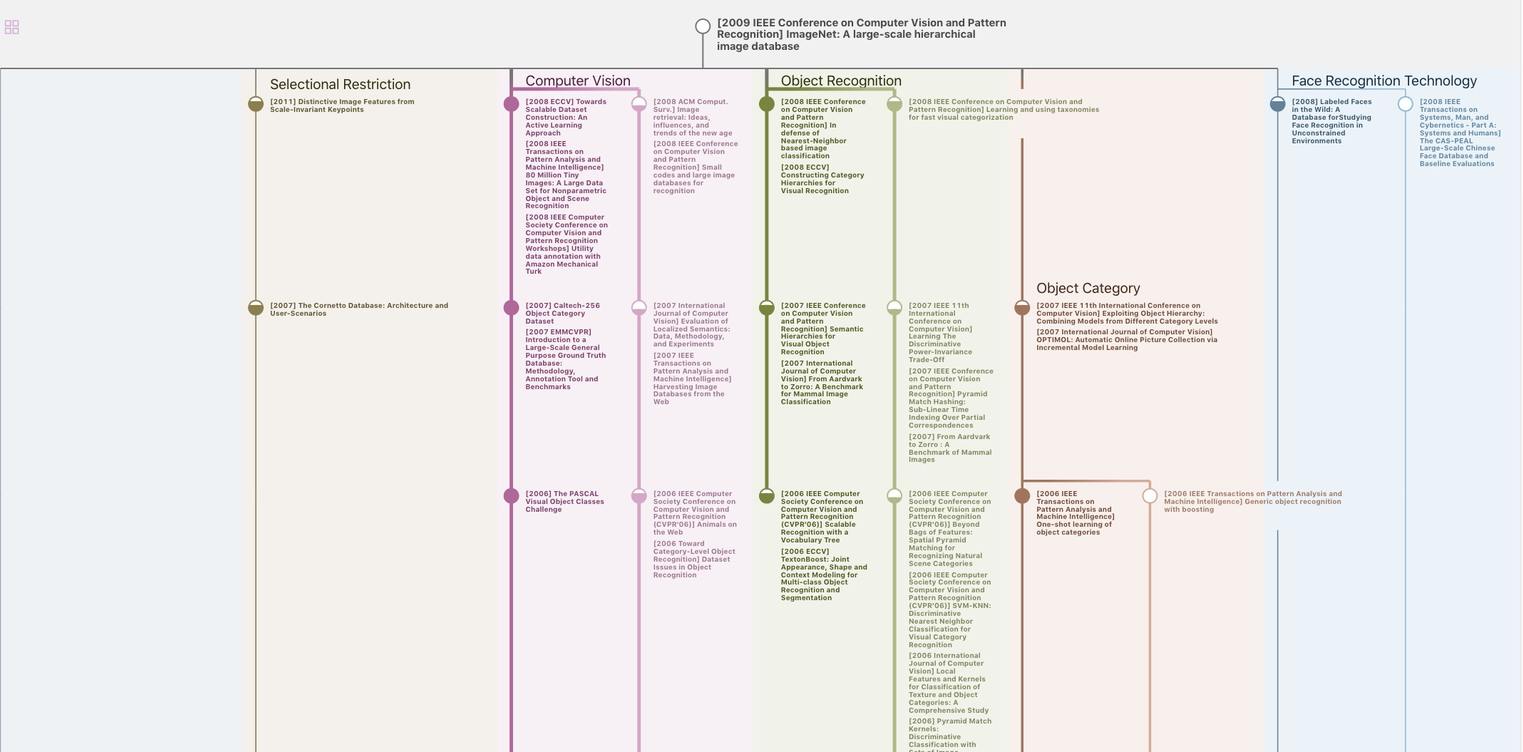
生成溯源树,研究论文发展脉络
Chat Paper
正在生成论文摘要