Towards Continual Learning Desiderata via HSIC-Bottleneck Orthogonalization and Equiangular Embedding
AAAI 2024(2024)
摘要
Deep neural networks are susceptible to catastrophic forgetting when trained on sequential tasks. Various continual learning (CL) methods often rely on exemplar buffers or/and network expansion for balancing model stability and plasticity, which, however, compromises their practical value due to privacy and memory concerns. Instead, this paper considers a strict yet realistic setting, where the training data from previous tasks is unavailable and the model size remains relatively constant during sequential training. To achieve such desiderata, we propose a conceptually simple yet effective method that attributes forgetting to layer-wise parameter overwriting and the resulting decision boundary distortion. This is achieved by the synergy between two key components: HSIC-Bottleneck Orthogonalization (HBO) implements non-overwritten parameter updates mediated by Hilbert-Schmidt independence criterion in an orthogonal space and EquiAngular Embedding (EAE) enhances decision boundary adaptation between old and new tasks with predefined basis vectors. Extensive experiments demonstrate that our method achieves competitive accuracy performance, even with absolute superiority of zero exemplar buffer and 1.02x the base model.
更多查看译文
关键词
ML: Life-Long and Continual Learning,ML: Time-Series/Data Streams,ML: Classification and Regression,ML: Other Foundations of Machine Learning
AI 理解论文
溯源树
样例
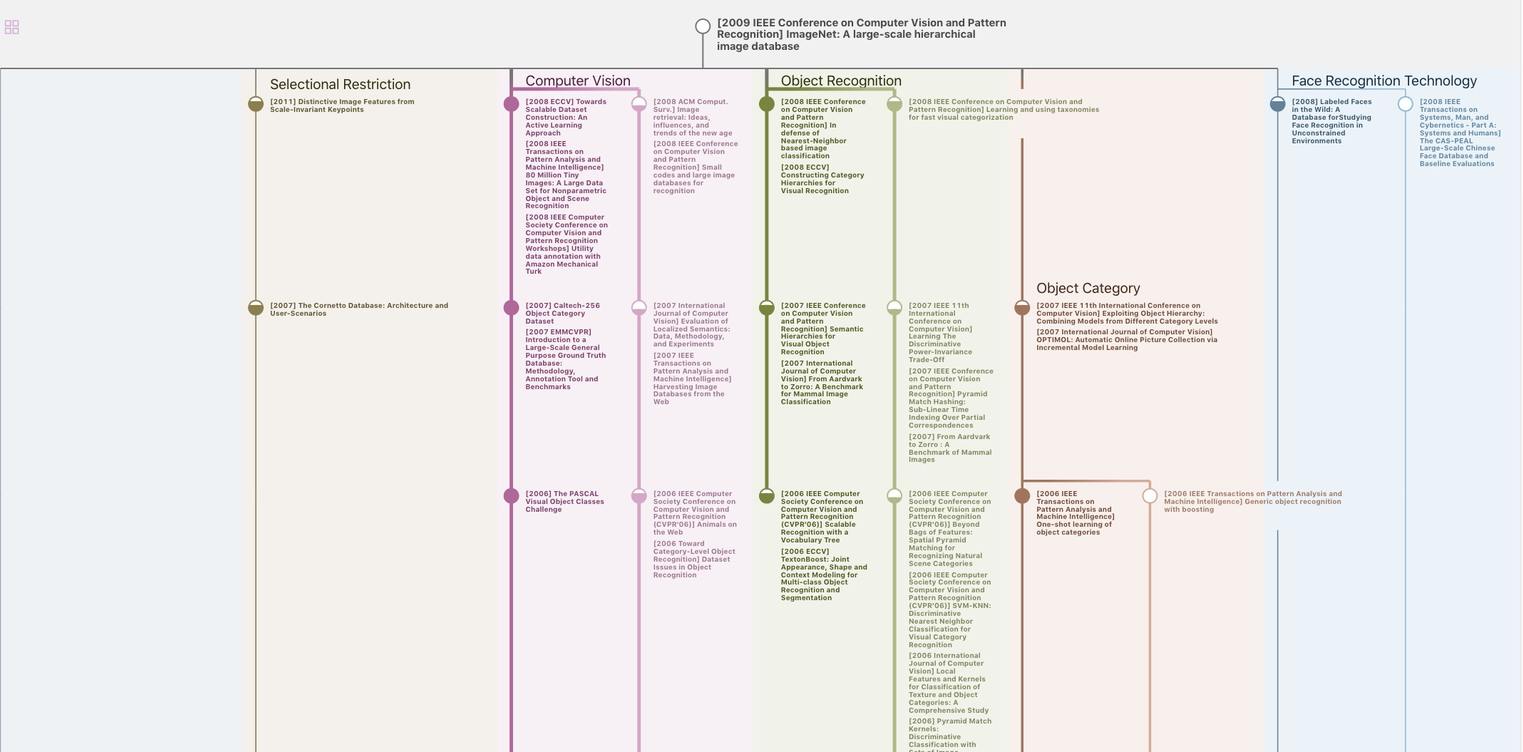
生成溯源树,研究论文发展脉络
Chat Paper
正在生成论文摘要