Meta-fourier neural operators for multi-task modeling of film cooling in gas turbine endwalls
ENGINEERING APPLICATIONS OF ARTIFICIAL INTELLIGENCE(2024)
摘要
Film cooling was a key technology to protect gas turbine endwalls from thermal ablation. Precise local temperature control was important for film cooling design on turbine endwalls, which required fast prediction of the two-dimensional cooling effectiveness. Supervised deep learning methods were feasible methods to fulfill such demand, but still faced challenges in the lack of data and generalization. A prediction model trained for a specific endwall could not be generalized to others at a low cost. To break through this bottleneck, this study proposed a meta learning method for film cooling prediction, which leveraged historical data to reduce the modeling cost and improve the generalization on a new film cooling prediction task. Four historical tasks and two new tasks for the film cooling prediction were created by changing the endwall pressure gradients. The number of samples available for modeling was limited to less than 10 for each new task. A Fourier Neural Operator was adopted to regress the film cooling effectiveness on endwall surfaces. Results showed that the proposed method reduced the amount of data required by 80% and the prediction error by 55% on the new film cooling design tasks.
更多查看译文
关键词
Gas turbine,Film cooling prediction,Pressure gradient,Historical data,Meta learning
AI 理解论文
溯源树
样例
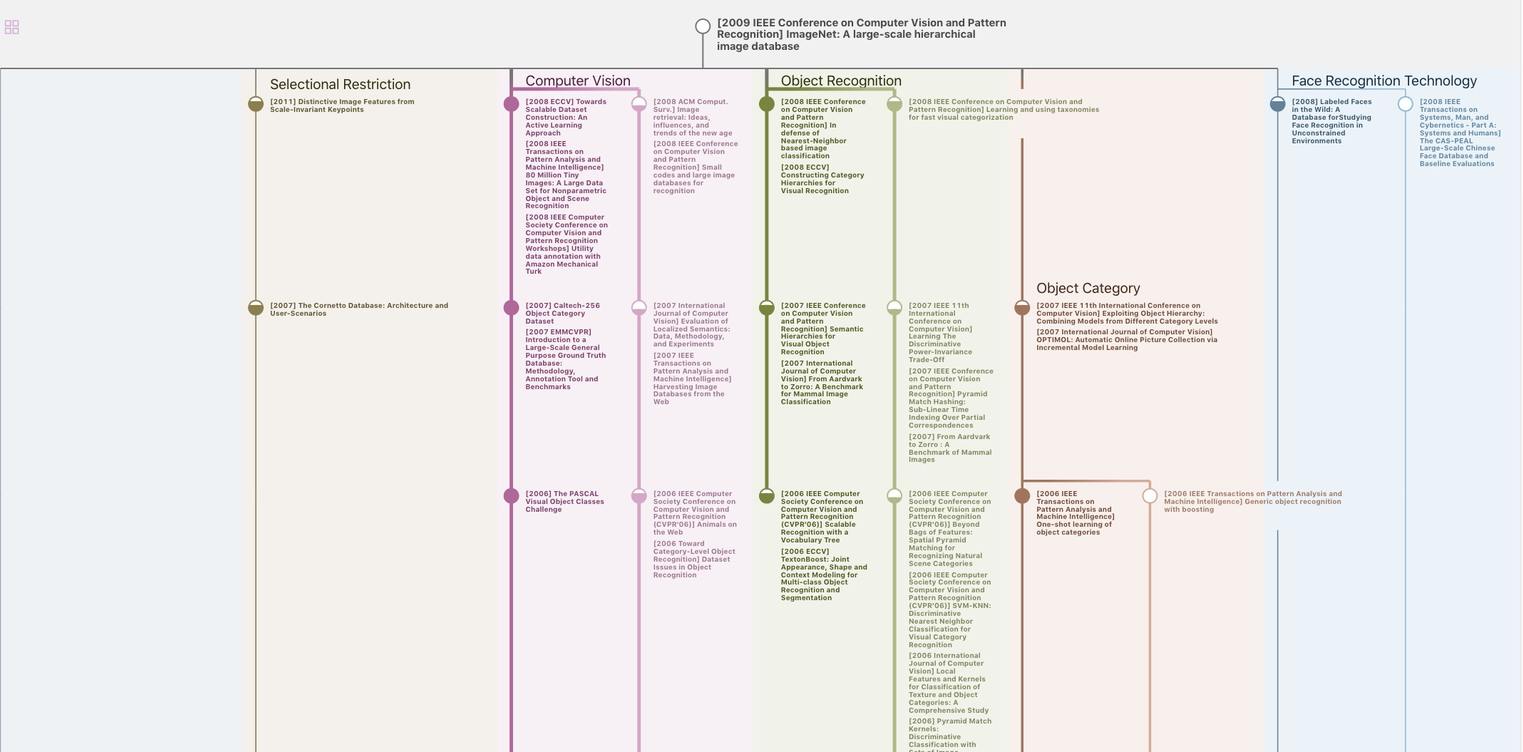
生成溯源树,研究论文发展脉络
Chat Paper
正在生成论文摘要