A Noise-Robust Heart Sound Segmentation Algorithm Based on Shannon Energy
IEEE ACCESS(2024)
摘要
Heart sound segmentation has been shown to improve the performance of artificial intelligence (AI)-based auscultation decision support systems increasingly viewed as a solution to compensate for eroding auscultatory skills and the associated subjectivity. Various segmentation approaches with demonstrated performance can be utilized for this task, but their robustness can suffer in the presence of noise. A noise-robust heart sound segmentation algorithm was developed and its accuracy was tested using two datasets: the CirCor DigiScope Phonocardiogram dataset and an in-house dataset - a heart murmur library collected at the Children's National Hospital (CNH). On the CirCor dataset, our segmentation algorithm marked the boundaries of the primary heart sounds S1 and S2 with an accuracy of 0.28 ms and 0.29 ms, respectively, and correctly identified the actual positive segments with a sensitivity of 97.44%. The algorithm also executed four times faster than a logistic regression hidden semi-Markov model. On the CNH dataset, the algorithm succeeded in 87.4% cases, achieving a 6% increase in segmentation success rate demonstrated by our original Shannon energy-based algorithm. Accurate heart sound segmentation is critical to supporting and accelerating AI research in cardiovascular diseases. The proposed algorithm increases the robustness of heart sound segmentation to noise and viability for clinical use.
更多查看译文
关键词
Auscultation,heart sound segmentation,Shannon energy,CirCor DigiScope phonocardiogram dataset,artificial intelligence,deep learning,stethoscope,heart murmur classification
AI 理解论文
溯源树
样例
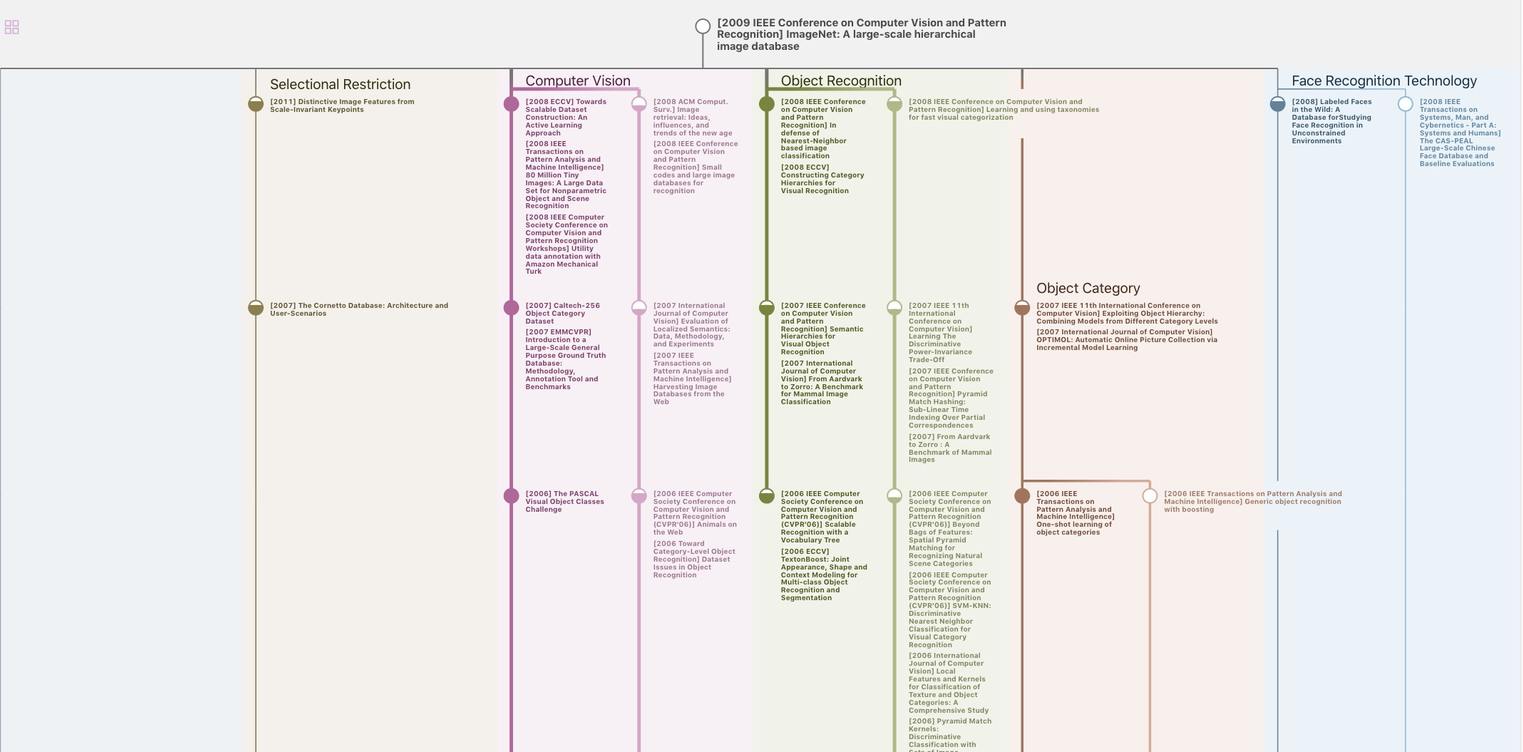
生成溯源树,研究论文发展脉络
Chat Paper
正在生成论文摘要