A data-knowledge-driven interval type-2 fuzzy neural network with interpretability and self-adaptive structure
INFORMATION SCIENCES(2024)
摘要
Interval type -2 fuzzy neural networks (IT2FNNs) have gained sustainable attention and wide applications because of their power of adaptive fuzzy modeling. Although the existing models accurately simulate the relationships between inputs and outputs by various adaptive learning algorithms, the parameters and fuzzy rules characterizing the model behaviors no longer have explicit interpretations. To achieve good interpretability and generalization performance, this paper proposes a novel data -knowledge -driven IT2FNN (DKIT2FNN). Unlike accuracy -oriented model fitting, DKIT2FNN pioneers the use of famous association rule mining techniques to automatically explore the interpretable rule knowledge, which intuitively presents conditional relation patterns among features. Using the obtained knowledge, the DKIT2FNN model is accomplished in a single -hidden layer network framework, in which the antecedent structure of each fuzzy rule is determined by the relation patterns provided by rule knowledge. In addition, an adversarial incremental learning algorithm is proposed for the self -organizing modeling of DKIT2FNN. Besides automatically assembling a compact rule base, it integrates the label adversarial training to reinforce the model's capability against label noise. We validate the DKIT2FNN on classification benchmark datasets with label noise and compare it with state-ofthe-art non -fuzzy and neuro-fuzzy methods. The results demonstrate that the proposed DKIT2FNN achieves robust classification performance and good interpretability.
更多查看译文
关键词
Fuzzy neural network,Interval type-2 fuzzy system,Association rule mining,Incremental learning
AI 理解论文
溯源树
样例
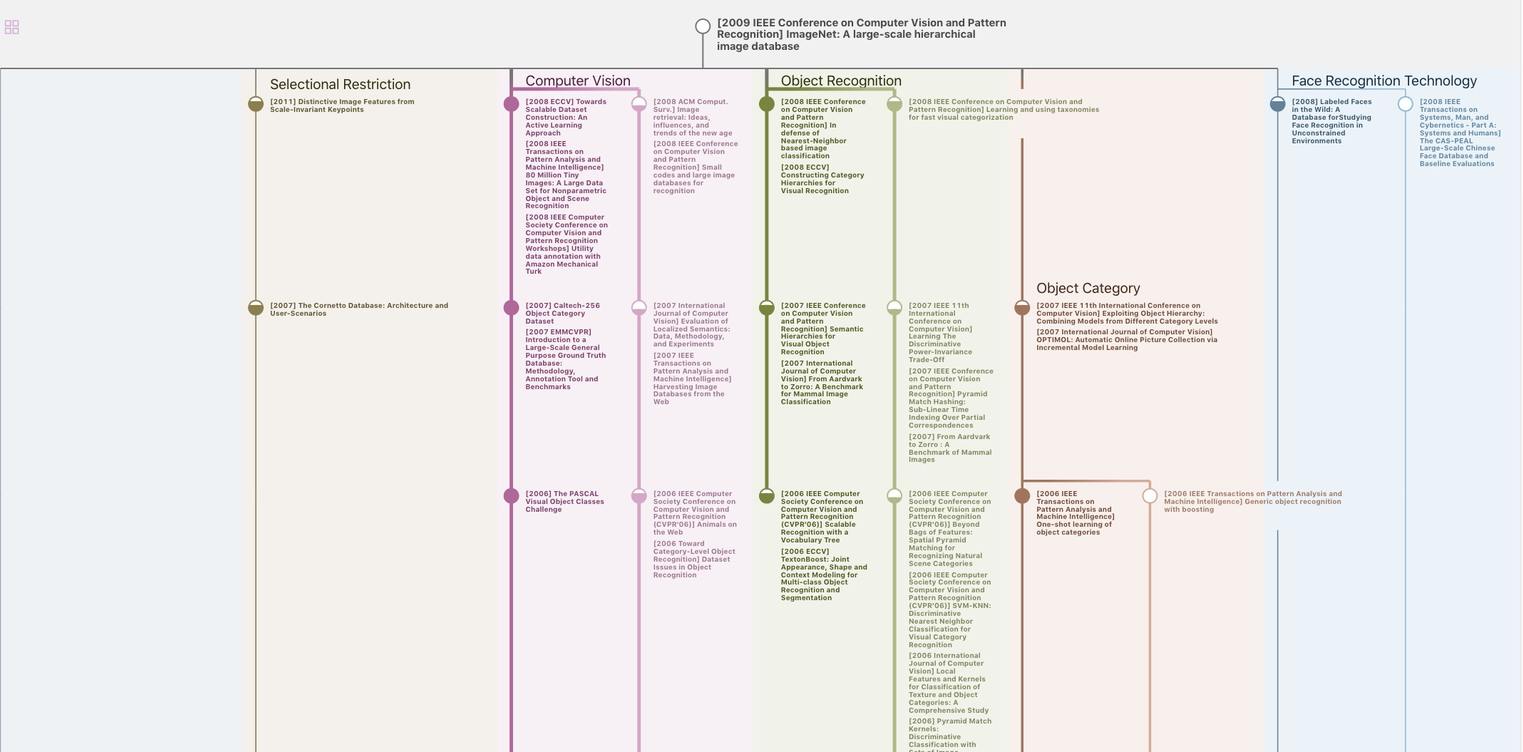
生成溯源树,研究论文发展脉络
Chat Paper
正在生成论文摘要