iG-LIO: An Incremental GICP-Based Tightly-Coupled LiDAR-Inertial Odometry
IEEE ROBOTICS AND AUTOMATION LETTERS(2024)
摘要
This work proposes an incremental Generalized Iterative Closest Point (GICP) based tightly-coupled LiDAR-inertial odometry (LIO), iG-LIO, which integrates the GICP constraints and inertial constraints into a unified estimation framework. iG-LIO uses a voxel-based surface covariance estimator to estimate the surface covariances of scans, and utilizes an incremental voxel map to represent the probabilistic models of surrounding environments. These methods successfully reduce the time consumption of the covariance estimation, nearest neighbor search, and map management. Extensive datasets collected from mechanical LiDARs and solid-state LiDARs are employed to evaluate the efficiency and accuracy of the proposed LIO. Even though iG-LIO keeps identical parameters across all datasets, the results show that it is more efficient than Faster-LIO while maintaining comparable accuracy with state-of-the-art LIO systems. The source code for iG-LIO has been open-sourced on GitHub: https://github.com/zijiechenrobotics/ig_lio.
更多查看译文
关键词
Odometry,Estimation,Laser radar,Measurement,Probabilistic logic,Indexes,Surface treatment,SLAM,sensor fusion,LiDAR-inertial odometry
AI 理解论文
溯源树
样例
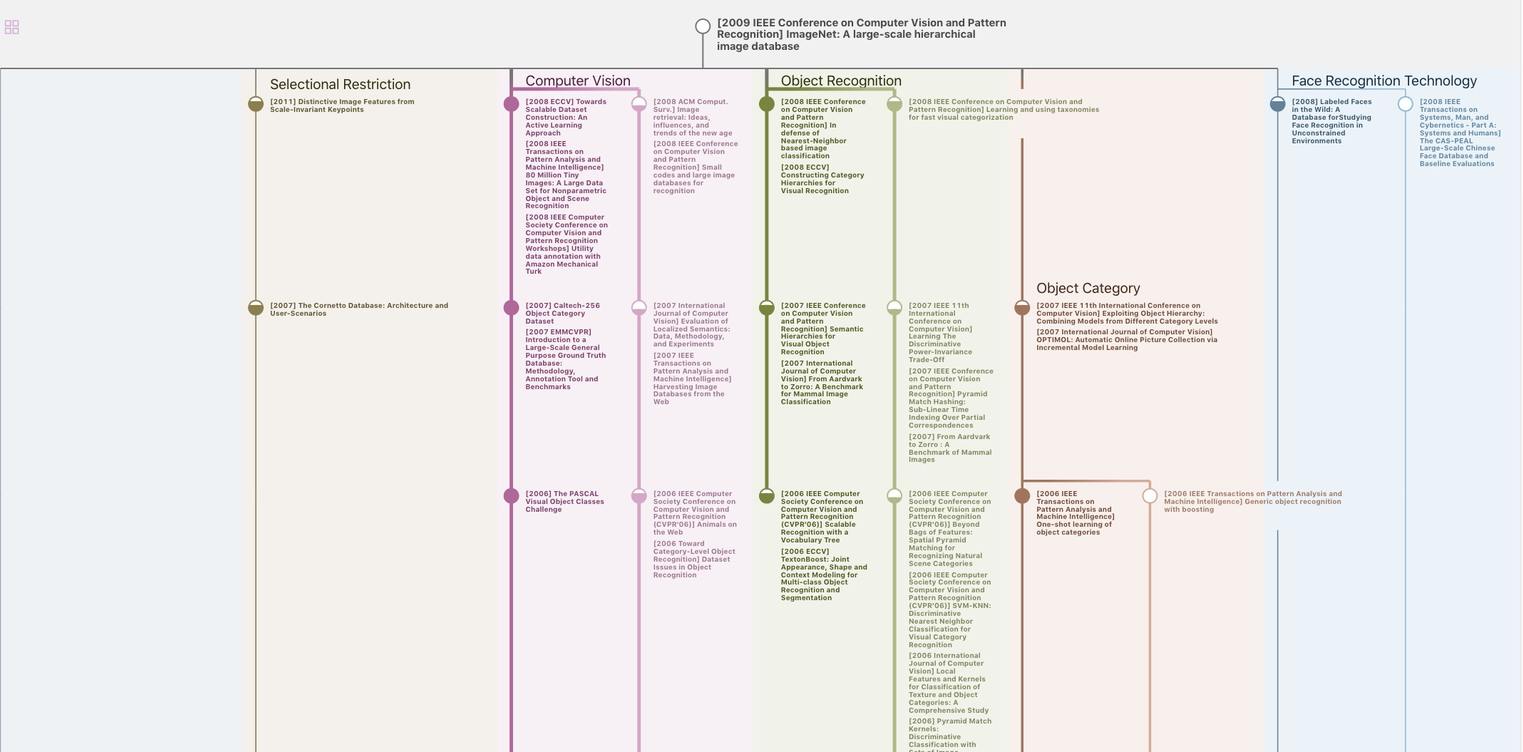
生成溯源树,研究论文发展脉络
Chat Paper
正在生成论文摘要