License Forecasting and Scheduling for HPC.
2023 31st International Symposium on Modeling, Analysis, and Simulation of Computer and Telecommunication Systems (MASCOTS)(2023)
摘要
This work focuses on forecasting future license usage for high-performance computing environments and using such predictions to improve the effectiveness of job scheduling. Specifically, we propose a model that carries out both short-term and long-term license usage forecasting and a method of using forecasts to improve job scheduling. Our long-term forecasting model achieves a Mean Absolute Percentage Error (MAPE) as low as 0.26 for a 12-month forecast of daily peak license usage. Our job scheduling experimental results also indicate that wasted work from jobs with insufficient licenses can be reduced by up to 92% without increasing the average license-using job completion times, during periods of high license usage, with our proposed license-aware scheduler.
更多查看译文
关键词
Software-as-a-Service (SaaS),High-Performance Computing (HPC),License Management,Machine Learning
AI 理解论文
溯源树
样例
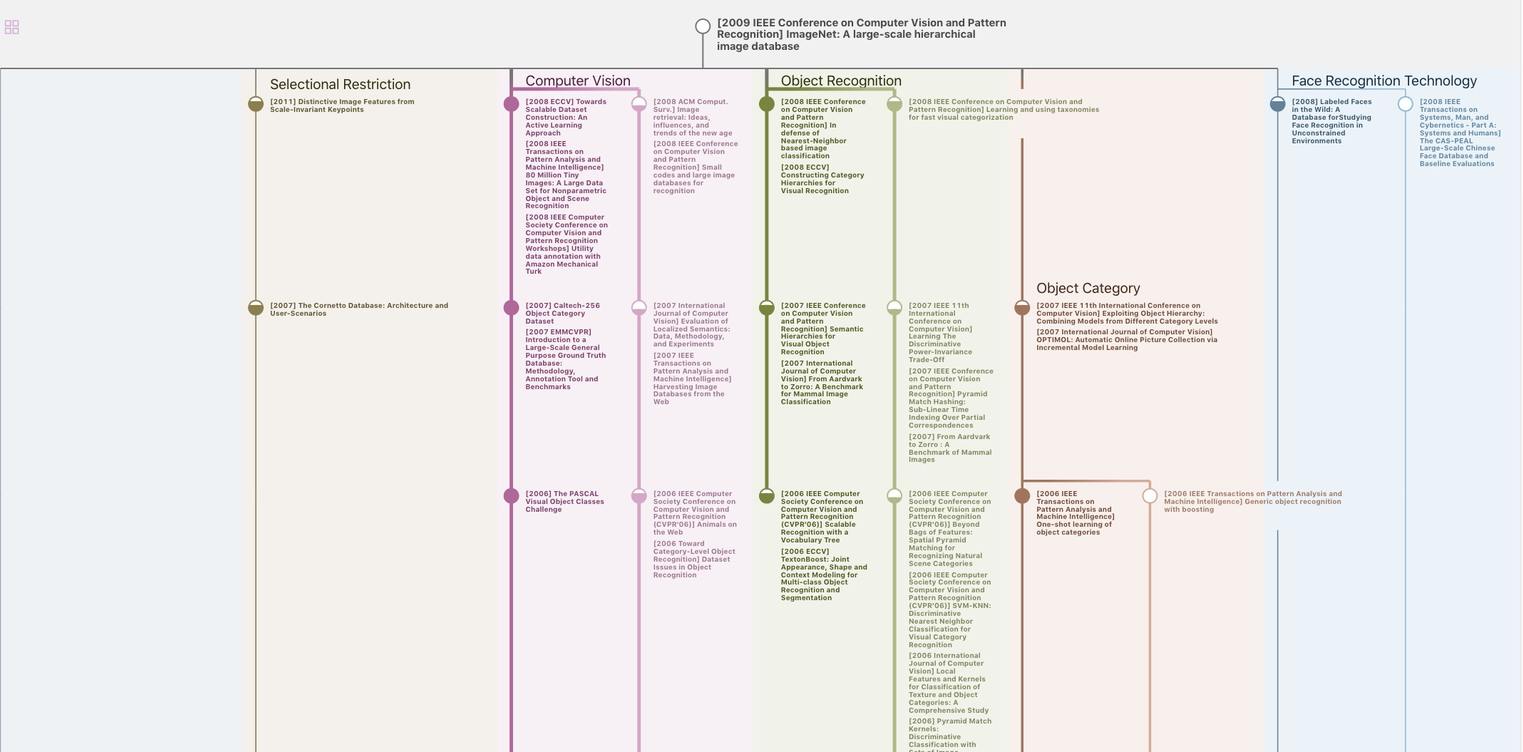
生成溯源树,研究论文发展脉络
Chat Paper
正在生成论文摘要