Simple Hack for Transformers against Heavy Long-Text Classification on a Time- and Memory-Limited GPU Service
2023 10th International Conference on Advanced Informatics: Concept, Theory and Application (ICAICTA)(2024)
摘要
Many NLP researchers rely on free computational services, such as Google
Colab, to fine-tune their Transformer models, causing a limitation for
hyperparameter optimization (HPO) in long-text classification due to the method
having quadratic complexity and needing a bigger resource. In Indonesian, only
a few works were found on long-text classification using Transformers. Most
only use a small amount of data and do not report any HPO. In this study, using
18k news articles, we investigate which pretrained models are recommended to
use based on the output length of the tokenizer. We then compare some hacks to
shorten and enrich the sequences, which are the removals of stopwords,
punctuation, low-frequency words, and recurring words. To get a fair
comparison, we propose and run an efficient and dynamic HPO procedure that can
be done gradually on a limited resource and does not require a long-running
optimization library. Using the best hack found, we then compare 512, 256, and
128 tokens length. We find that removing stopwords while keeping punctuation
and low-frequency words is the best hack. Some of our setups manage to
outperform taking 512 first tokens using a smaller 128 or 256 first tokens
which manage to represent the same information while requiring less
computational resources. The findings could help developers to efficiently
pursue optimal performance of the models using limited resources.
更多查看译文
关键词
indonesian,long text,classification,transformer,optimization
AI 理解论文
溯源树
样例
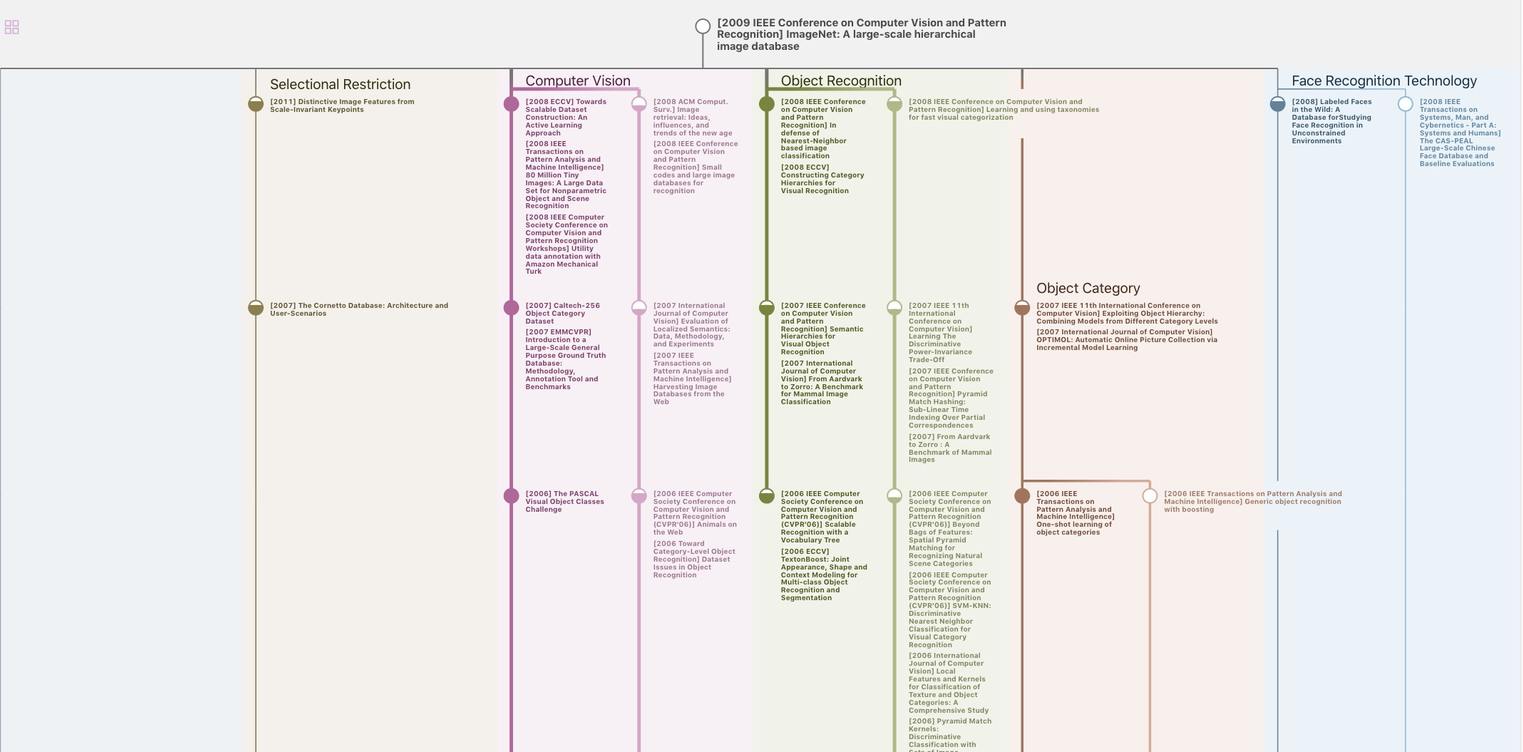
生成溯源树,研究论文发展脉络
Chat Paper
正在生成论文摘要