Learning With Side Information: Elastic Multi-Resource Control for the Open RAN.
IEEE J. Sel. Areas Commun.(2024)
摘要
The open radio access network (O-RAN) architecture provides enhanced opportunities for integrating machine learning in 5G/6G resource management by decomposing RAN functionalities. Yet, generic learning mechanisms either do not fully exploit the disaggregated non-real-time and near-real-time RAN controllers or ignore the potential elasticity of application demands, another degree of freedom in managing RAN resources. We introduce a two-timescale framework aimed at optimizing users’ long-term total QoS. Rather than reactive resource allocation, our approach proactively modifies multi-resource user demands using congestion indicators, prior to enforcing any allocation rules. Addressing the issue of insufficient user feedback on individual resource utilities, we employ a bandit-feedback version of the combinatorial multi-armed bandit framework to deduce resource-specific signals. Also, to compensate for insufficient and infrequent feedback, we’ve developed an algorithm that gleans side information from live network traffic to refine predictions on user resource sensitivities. This streamlines the algorithm’s optimality convergence and leverages the two-tier O-RAN controller structure. We validate our algorithms’ efficacy through analysis and 5G usage experiments, revealing our proposed method improves application utility by 13-60%, throughput by 8-19%, and reduces latency by 10-18%.
更多查看译文
关键词
Open radio access network (Open RAN),elastic multi-resource management,online learning
AI 理解论文
溯源树
样例
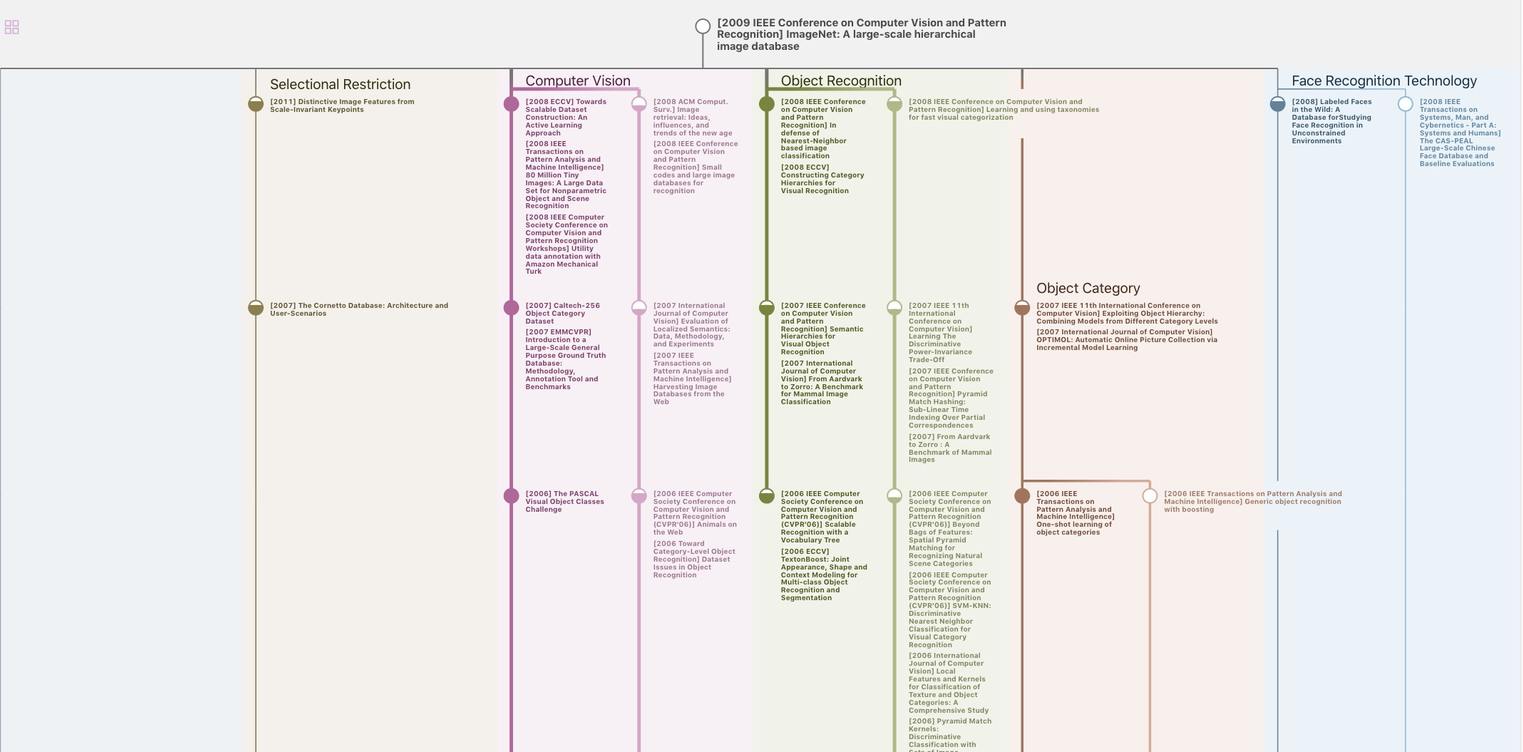
生成溯源树,研究论文发展脉络
Chat Paper
正在生成论文摘要