Seasonal threshold to reduce false positives for prediction-based outlier detection in building energy data
JOURNAL OF BUILDING ENGINEERING(2024)
摘要
Recent studies have shown that artificial intelligence-based model predictive control systems have the potential to optimize building operations for energy and cost. But for these systems to function as designed, they require high-quality datasets, which can be challenging when working with actual sensor data that may contain missing or outlier values. Using contaminated data in these carefully tuned machine learning algorithms can cause errors in the prediction results, leading to unexpected building operations. There is a need to clean incoming data in real-time by detecting the outliers in incoming sensor data and correcting them to an appropriate value before incorporating them into the prediction algorithm. This research proposes using a seasonal threshold for prediction-based point outlier detection methods to accurately detect the outliers while maintaining the reliability of the data by reducing false positive detections. The approach combining the global and seasonal threshold showed improvement to the detection accuracy by 83 % and reduced the falsely positives by up to 78 % for the best improvement, making the method a more trustworthy way to detect outliers in building energy data. This method can utilize an already existing model to detect outliers is a promising approach, especially for buildings that have already been implemented or are in the process of implementing an artificial intelligencebased control systems. This will allow the system to predict and control building energy without interruption and with reduced risk of unexpected operation due to corrupted data.
更多查看译文
关键词
Outlier detection,Demand prediction,Artificial intelligence,Model predictive control,Deep neural network
AI 理解论文
溯源树
样例
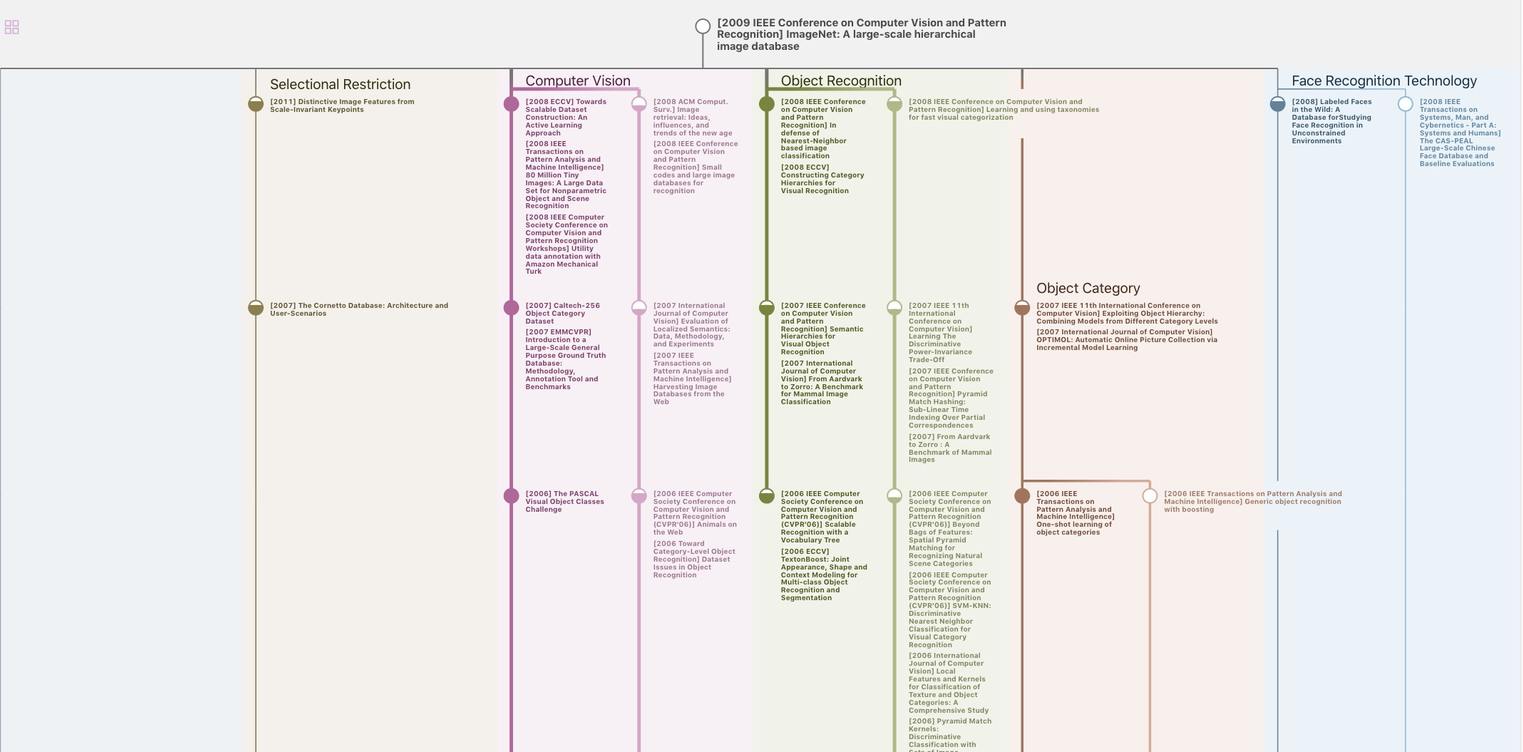
生成溯源树,研究论文发展脉络
Chat Paper
正在生成论文摘要