Development of a combined radiomics and CT feature-based model for differentiating malignant from benign subcentimeter solid pulmonary nodules
European Radiology Experimental(2024)
摘要
Background We aimed to develop a combined model based on radiomics and computed tomography (CT) imaging features for use in differential diagnosis of benign and malignant subcentimeter (≤ 10 mm) solid pulmonary nodules (SSPNs). Methods A total of 324 patients with SSPNs were analyzed retrospectively between May 2016 and June 2022. Malignant nodules ( n = 158) were confirmed by pathology, and benign nodules ( n = 166) were confirmed by follow-up or pathology. SSPNs were divided into training ( n = 226) and testing ( n = 98) cohorts. A total of 2107 radiomics features were extracted from contrast-enhanced CT. The clinical and CT characteristics retained after univariate and multivariable logistic regression analyses were used to develop the clinical model. The combined model was established by associating radiomics features with CT imaging features using logistic regression. The performance of each model was evaluated using the area under the receiver-operating characteristic curve (AUC). Results Six CT imaging features were independent predictors of SSPNs, and four radiomics features were selected after a dimensionality reduction. The combined model constructed by the logistic regression method had the best performance in differentiating malignant from benign SSPNs, with an AUC of 0.942 (95% confidence interval 0.918–0.966) in the training group and an AUC of 0.930 (0.902–0.957) in the testing group. The decision curve analysis showed that the combined model had clinical application value. Conclusions The combined model incorporating radiomics and CT imaging features had excellent discriminative ability and can potentially aid radiologists in diagnosing malignant from benign SSPNs. Relevance statement The model combined radiomics features and clinical features achieved good efficiency in predicting malignant from benign SSPNs, having the potential to assist in early diagnosis of lung cancer and improving follow-up strategies in clinical work. Key points • We developed a pulmonary nodule diagnostic model including radiomics and CT features. • The model yielded the best performance in differentiating malignant from benign nodules. • The combined model had clinical application value and excellent discriminative ability. • The model can assist radiologists in diagnosing malignant from benign pulmonary nodules. Graphical Abstract
更多查看译文
关键词
Diagnosis (differential),Machine learning,Nomograms,Solitary pulmonary nodule,Tomography (x-ray computed)
AI 理解论文
溯源树
样例
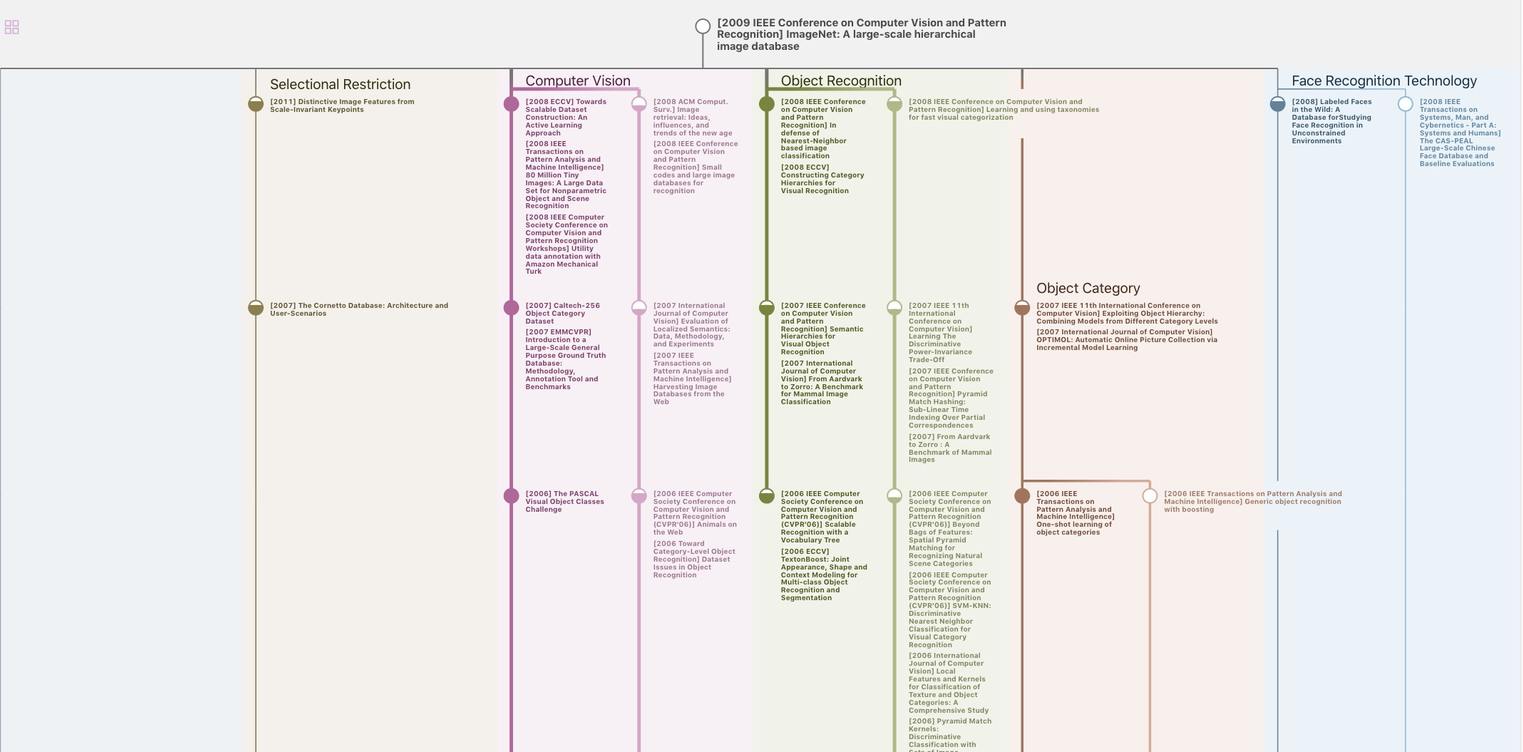
生成溯源树,研究论文发展脉络
Chat Paper
正在生成论文摘要