Understanding User Experience in Large Language Model Interactions
CoRR(2024)
摘要
In the rapidly evolving landscape of large language models (LLMs), most
research has primarily viewed them as independent individuals, focusing on
assessing their capabilities through standardized benchmarks and enhancing
their general intelligence. This perspective, however, tends to overlook the
vital role of LLMs as user-centric services in human-AI collaboration. This gap
in research becomes increasingly critical as LLMs become more integrated into
people's everyday and professional interactions. This study addresses the
important need to understand user satisfaction with LLMs by exploring four key
aspects: comprehending user intents, scrutinizing user experiences, addressing
major user concerns about current LLM services, and charting future research
paths to bolster human-AI collaborations. Our study develops a taxonomy of 7
user intents in LLM interactions, grounded in analysis of real-world user
interaction logs and human verification. Subsequently, we conduct a user survey
to gauge their satisfaction with LLM services, encompassing usage frequency,
experiences across intents, and predominant concerns. This survey, compiling
411 anonymous responses, uncovers 11 first-hand insights into the current state
of user engagement with LLMs. Based on this empirical analysis, we pinpoint 6
future research directions prioritizing the user perspective in LLM
developments. This user-centered approach is essential for crafting LLMs that
are not just technologically advanced but also resonate with the intricate
realities of human interactions and real-world applications.
更多查看译文
AI 理解论文
溯源树
样例
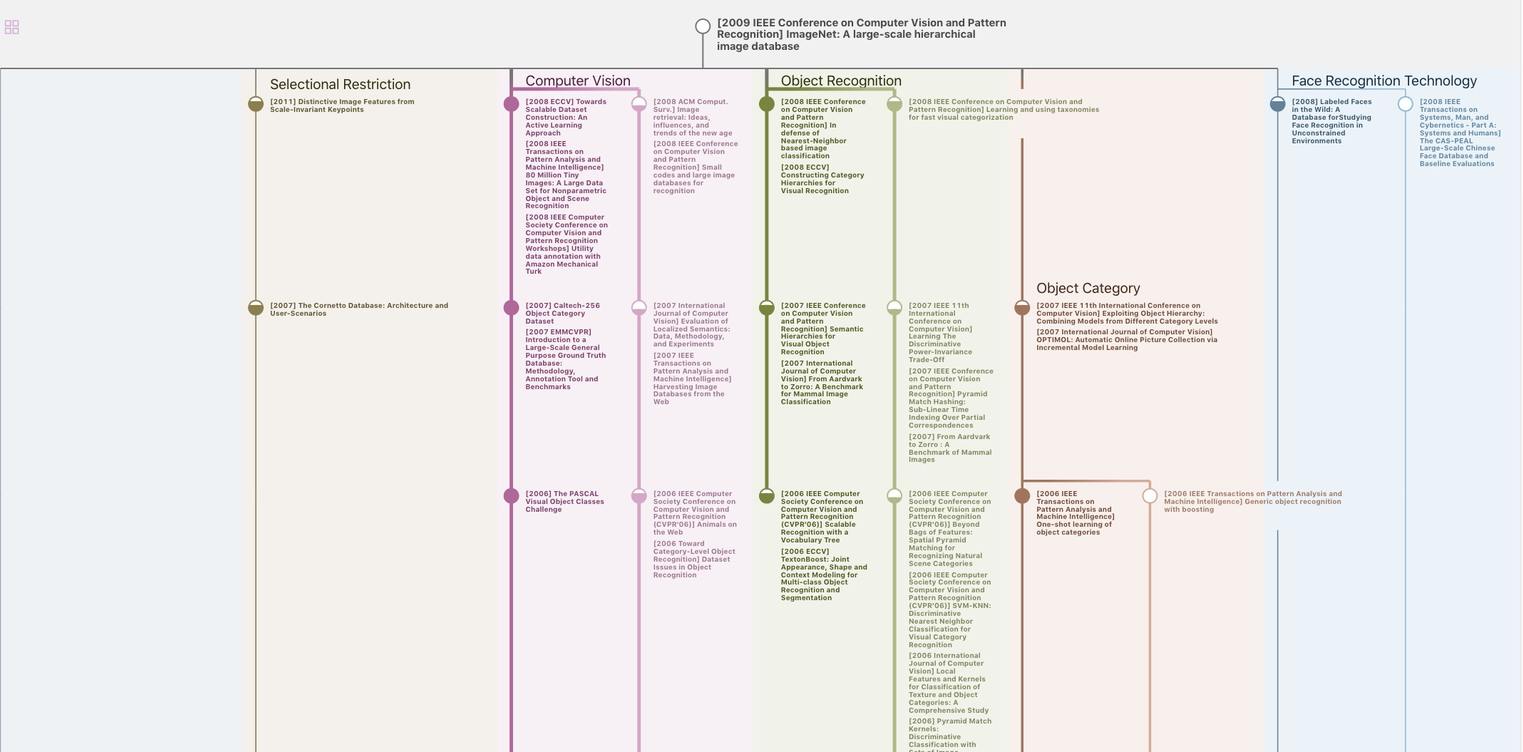
生成溯源树,研究论文发展脉络
Chat Paper
正在生成论文摘要