ECAPA2: A Hybrid Neural Network Architecture and Training Strategy for Robust Speaker Embeddings
2023 IEEE Automatic Speech Recognition and Understanding Workshop (ASRU)(2024)
摘要
In this paper, we present ECAPA2, a novel hybrid neural network architecture
and training strategy to produce robust speaker embeddings. Most speaker
verification models are based on either the 1D- or 2D-convolutional operation,
often manifested as Time Delay Neural Networks or ResNets, respectively. Hybrid
models are relatively unexplored without an intuitive explanation what
constitutes best practices in regard to its architectural choices. We motivate
the proposed ECAPA2 model in this paper with an analysis of current speaker
verification architectures. In addition, we propose a training strategy which
makes the speaker embeddings more robust against overlapping speech and short
utterance lengths. The presented ECAPA2 architecture and training strategy
attains state-of-the-art performance on the VoxCeleb1 test sets with
significantly less parameters than current models. Finally, we make a
pre-trained model publicly available to promote research on downstream tasks.
更多查看译文
关键词
speaker verification,speaker embeddings,ECAPA2
AI 理解论文
溯源树
样例
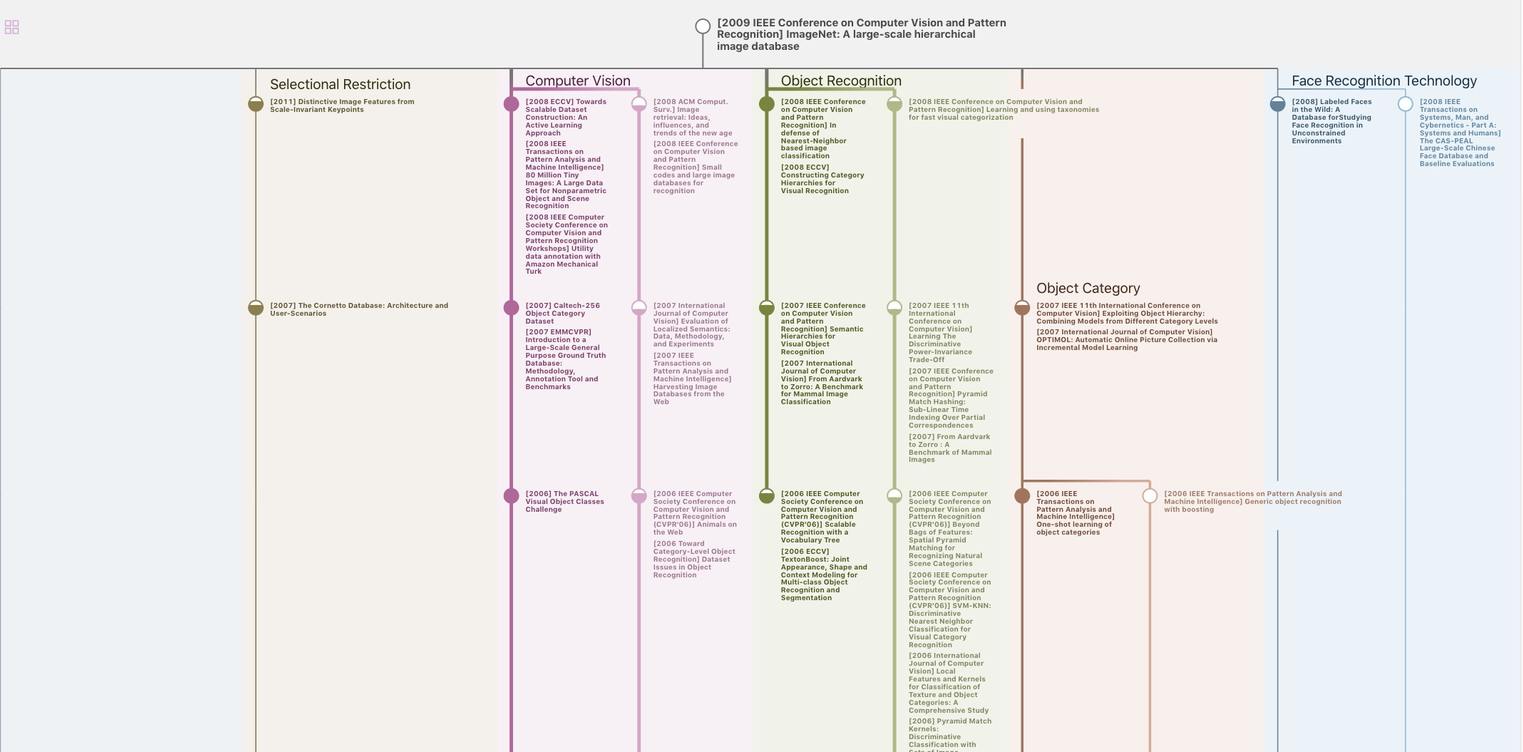
生成溯源树,研究论文发展脉络
Chat Paper
正在生成论文摘要