A Hypernetwork Based Framework for Non-Stationary Channel Prediction
IEEE Transactions on Vehicular Technology(2024)
摘要
In order to break through the development bottleneck of modern wireless
communication networks, a critical issue is the out-of-date channel state
information (CSI) in high mobility scenarios. In general, non-stationary CSI
has statistical properties which vary with time, implying that the data
distribution changes continuously over time. This temporal distribution shift
behavior undermines the accurate channel prediction and it is still an open
problem in the related literature. In this paper, a hypernetwork based
framework is proposed for non-stationary channel prediction. The framework aims
to dynamically update the neural network (NN) parameters as the wireless
channel changes to automatically adapt to various input CSI distributions.
Based on this framework, we focus on low-complexity hypernetwork design and
present a deep learning (DL) based channel prediction method, termed as LPCNet,
which improves the CSI prediction accuracy with acceptable complexity.
Moreover, to maximize the achievable downlink spectral efficiency (SE), a joint
channel prediction and beamforming (BF) method is developed, termed as JLPCNet,
which seeks to predict the BF vector. Our numerical results showcase the
effectiveness and flexibility of the proposed framework, and demonstrate the
superior performance of LPCNet and JLPCNet in various scenarios for fixed and
varying user speeds.
更多查看译文
关键词
Deep learning,high mobility,hypernetwork,non-stationary channel prediction,temporal distribution shift
AI 理解论文
溯源树
样例
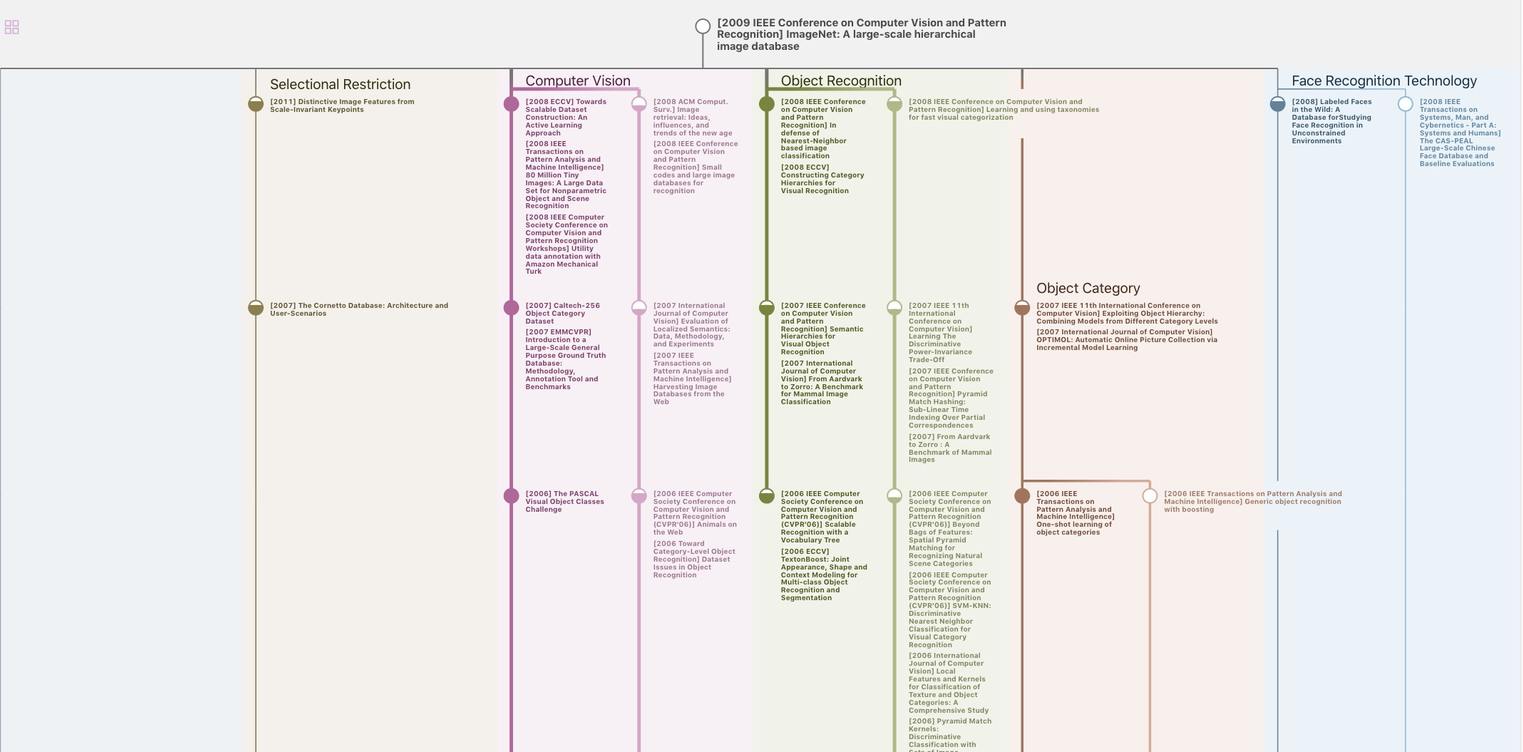
生成溯源树,研究论文发展脉络
Chat Paper
正在生成论文摘要