Improving OCR Quality in 19th Century Historical Documents Using a Combined Machine Learning Based Approach
CoRR(2024)
摘要
This paper addresses a major challenge to historical research on the 19th
century. Large quantities of sources have become digitally available for the
first time, while extraction techniques are lagging behind. Therefore, we
researched machine learning (ML) models to recognise and extract complex data
structures in a high-value historical primary source, the Schematismus. It
records every single person in the Habsburg civil service above a certain
hierarchical level between 1702 and 1918 and documents the genesis of the
central administration over two centuries. Its complex and intricate structure
as well as its enormous size have so far made any more comprehensive analysis
of the administrative and social structure of the later Habsburg Empire on the
basis of this source impossible. We pursued two central objectives: Primarily,
the improvement of the OCR quality, for which we considered an improved
structure recognition to be essential; in the further course, it turned out
that this also made the extraction of the data structure possible. We chose
Faster R-CNN as base for the ML architecture for structure recognition. In
order to obtain the required amount of training data quickly and economically,
we synthesised Hof- und Staatsschematismus-style data, which we used to train
our model. The model was then fine-tuned with a smaller set of manually
annotated historical source data. We then used Tesseract-OCR, which was further
optimised for the style of our documents, to complete the combined structure
extraction and OCR process. Results show a significant decrease in the two
standard parameters of OCR-performance, WER and CER (where lower values are
better). Combined structure detection and fine-tuned OCR improved CER and WER
values by remarkable 71.98 percent (CER) respectively 52.49 percent (WER).
更多查看译文
AI 理解论文
溯源树
样例
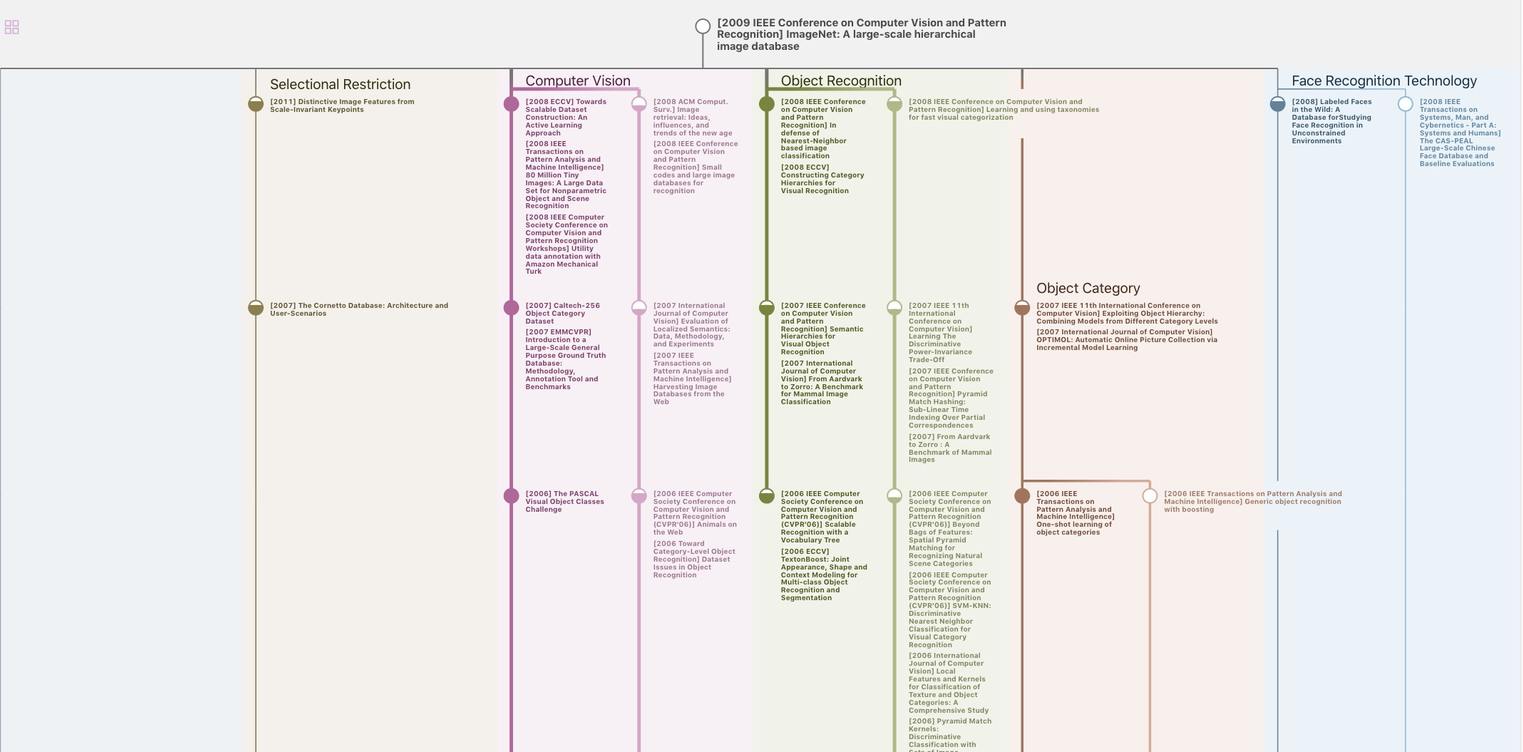
生成溯源树,研究论文发展脉络
Chat Paper
正在生成论文摘要