Graph Transformer GANs with Graph Masked Modeling for Architectural Layout Generation.
IEEE transactions on pattern analysis and machine intelligence(2024)
摘要
We present a novel graph Transformer generative adversarial network (GTGAN) to learn effective graph node relations in an end-to-end fashion for challenging graph-constrained architectural layout generation tasks. The proposed graph-Transformer-based generator includes a novel graph Transformer encoder that combines graph convolutions and self-attentions in a Transformer to model both local and global interactions across connected and non-connected graph nodes. Specifically, the proposed connected node attention (CNA) and non-connected node attention (NNA) aim to capture the global relations across connected nodes and non-connected nodes in the input graph, respectively. The proposed graph modeling block (GMB) aims to exploit local vertex interactions based on a house layout topology. Moreover, we propose a new node classification-based discriminator to preserve the high-level semantic and discriminative node features for different house components. To maintain the relative spatial relationships between ground truth and predicted graphs, we also propose a novel graph-based cycle-consistency loss. Finally, we propose a novel self-guided pre-training method for graph representation learning. This approach involves simultaneous masking of nodes and edges at an elevated mask ratio (i.e., 40%) and their subsequent reconstruction using an asymmetric graph-centric autoencoder architecture. This method markedly improves the model's learning proficiency and expediency. Experiments on three challenging graph-constrained architectural layout generation tasks (i.e., house layout generation, house roof generation, and building layout generation) with three public datasets demonstrate the effectiveness of the proposed method in terms of objective quantitative scores and subjective visual realism. New state-of-the-art results are established by large margins on these three tasks.
更多查看译文
关键词
GANs,Graph,Transformer,Masked Modeling,Architectural Layout Generation
AI 理解论文
溯源树
样例
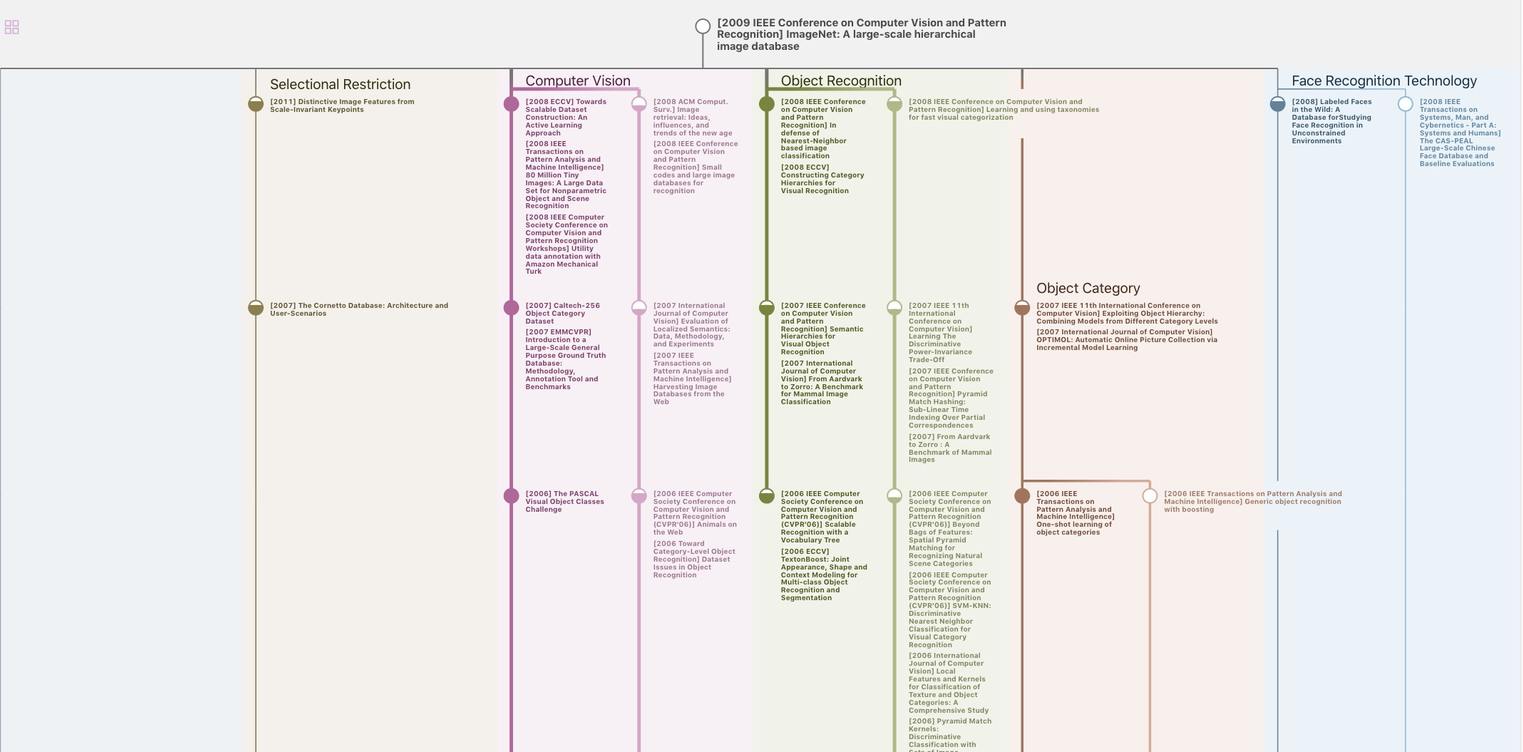
生成溯源树,研究论文发展脉络
Chat Paper
正在生成论文摘要