Accelerated Gradient Methods with Gradient Restart: Global Linear Convergence
arxiv(2024)
摘要
Gradient restarting has been shown to improve the numerical performance of
accelerated gradient methods. This paper provides a mathematical analysis to
understand these advantages. First, we establish global linear convergence
guarantees for the gradient restarted accelerated proximal gradient method when
solving strongly convex composite optimization problems. Second, through
analysis of the corresponding ordinary differential equation model, we prove
the continuous trajectory of gradient restarted Nesterov's accelerated gradient
method exhibits global linear convergence for quadratic strongly convex
objectives, while the non-restarted version provably lacks this property by
[Su, Boyd, and Candés, J. Mach. Learn. Res., 2016, 17(153), 1-43].
更多查看译文
AI 理解论文
溯源树
样例
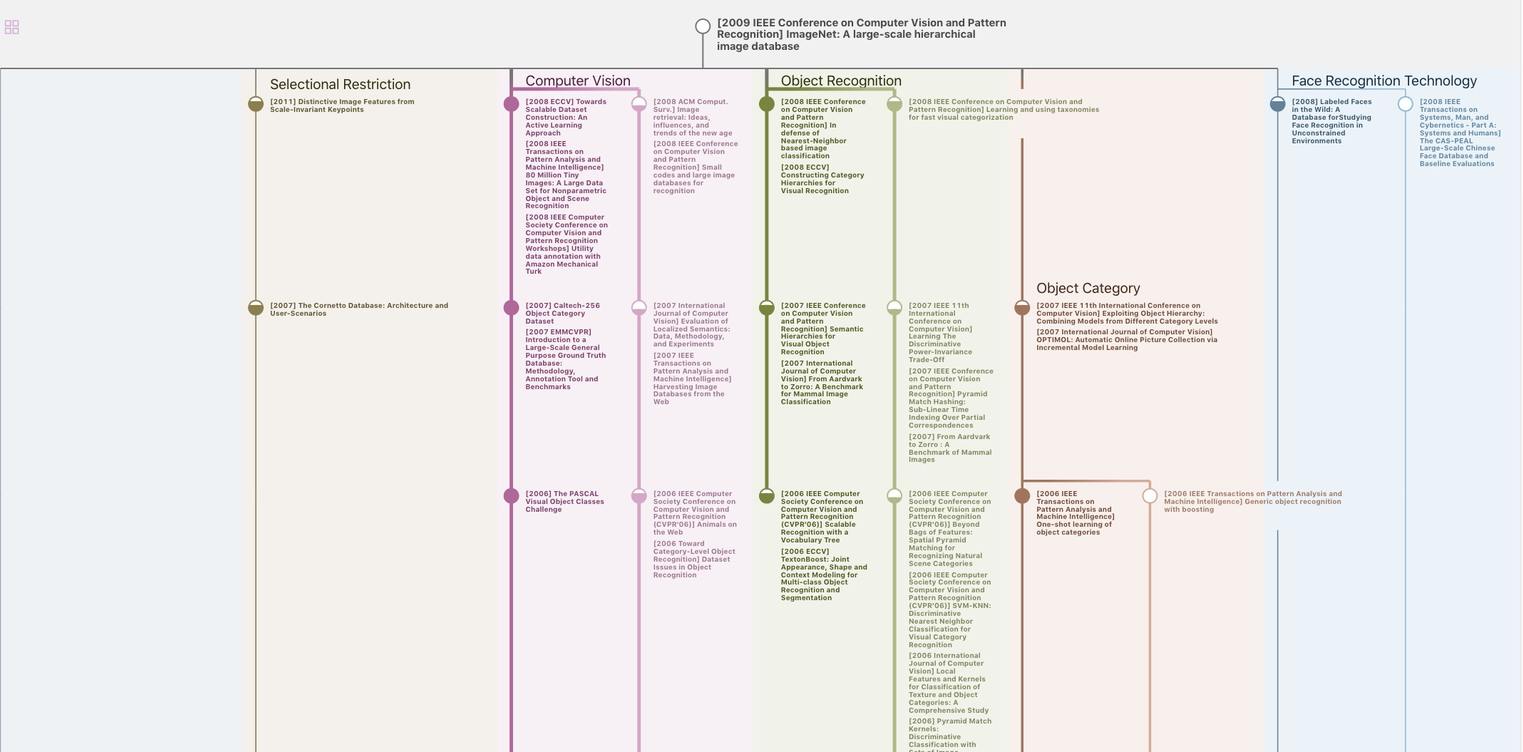
生成溯源树,研究论文发展脉络
Chat Paper
正在生成论文摘要