PolMERLIN: Self-Supervised Polarimetric Complex SAR Image Despeckling With Masked Networks
IEEE GEOSCIENCE AND REMOTE SENSING LETTERS(2024)
摘要
Despeckling is a crucial noise reduction task in improving the quality of synthetic aperture radar (SAR) images. Directly obtaining noise-free SAR images is a challenging task that has hindered the development of accurate despeckling algorithms. The advent of deep learning has facilitated the study of denoising models that learn from only noisy SAR images. However, existing methods deal solely with single-polarization images and cannot handle the multipolarization images captured by modern satellites. In this work, we present an extension of the existing model for generating single-polarization SAR images to handle multipolarization SAR images. Specifically, we propose a novel self-supervised despeckling approach called channel masking, which exploits the relationship between polarizations. Additionally, we utilize a spatial masking method that addresses pixel-to-pixel correlations to further enhance the performance of our approach. By effectively incorporating multiple polarization information, our method surpasses current state-of-the-art methods in quantitative evaluation in both synthetic and real-world scenarios.
更多查看译文
关键词
Radar polarimetry,Training,Speckle,Correlation,Noise reduction,Noise measurement,Mathematical models,Despeckling,polarimetric synthetic aperture radar (SAR) image
AI 理解论文
溯源树
样例
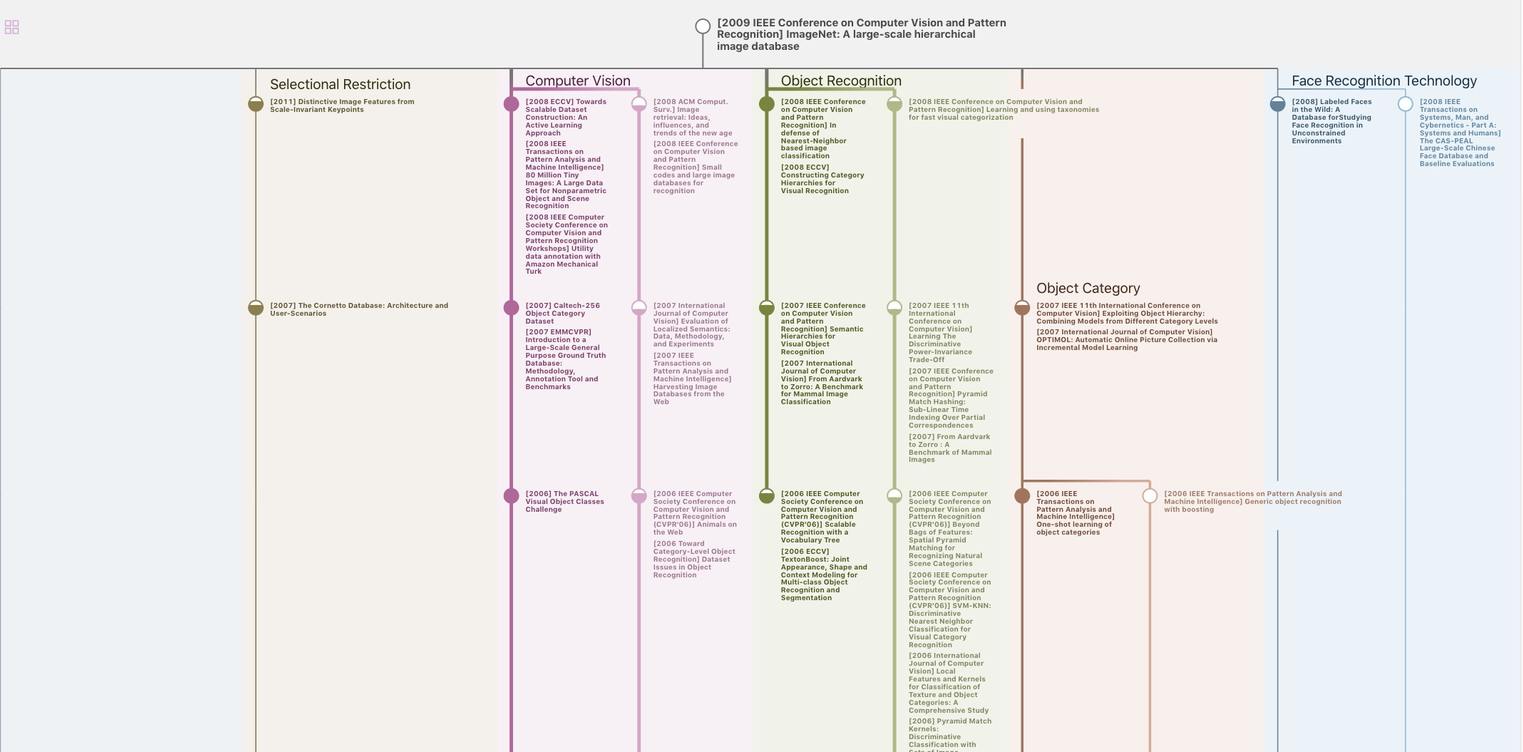
生成溯源树,研究论文发展脉络
Chat Paper
正在生成论文摘要