Sequential Monte-Carlo testing by betting
arxiv(2024)
摘要
In a Monte-Carlo test, the observed dataset is fixed, and several resampled
or permuted versions of the dataset are generated in order to test a null
hypothesis that the original dataset is exchangeable with the
resampled/permuted ones. Sequential Monte-Carlo tests aim to save computational
resources by generating these additional datasets sequentially one by one, and
potentially stopping early. While earlier tests yield valid inference at a
particular prespecified stopping rule, our work develops a new anytime-valid
Monte-Carlo test that can be continuously monitored, yielding a p-value or
e-value at any stopping time possibly not specified in advance. Despite the
added flexibility, it significantly outperforms the well-known method by Besag
and Clifford, stopping earlier under both the null and the alternative without
compromising power. The core technical advance is the development of new test
martingales (nonnegative martingales with initial value one) for testing
exchangeability against a very particular alternative. These test martingales
are constructed using new and simple betting strategies that smartly bet on the
relative ranks of generated test statistics. The betting strategies are guided
by the derivation of a simple log-optimal betting strategy, have closed form
expressions for the wealth process, provable guarantees on resampling risk, and
display excellent power in practice.
更多查看译文
AI 理解论文
溯源树
样例
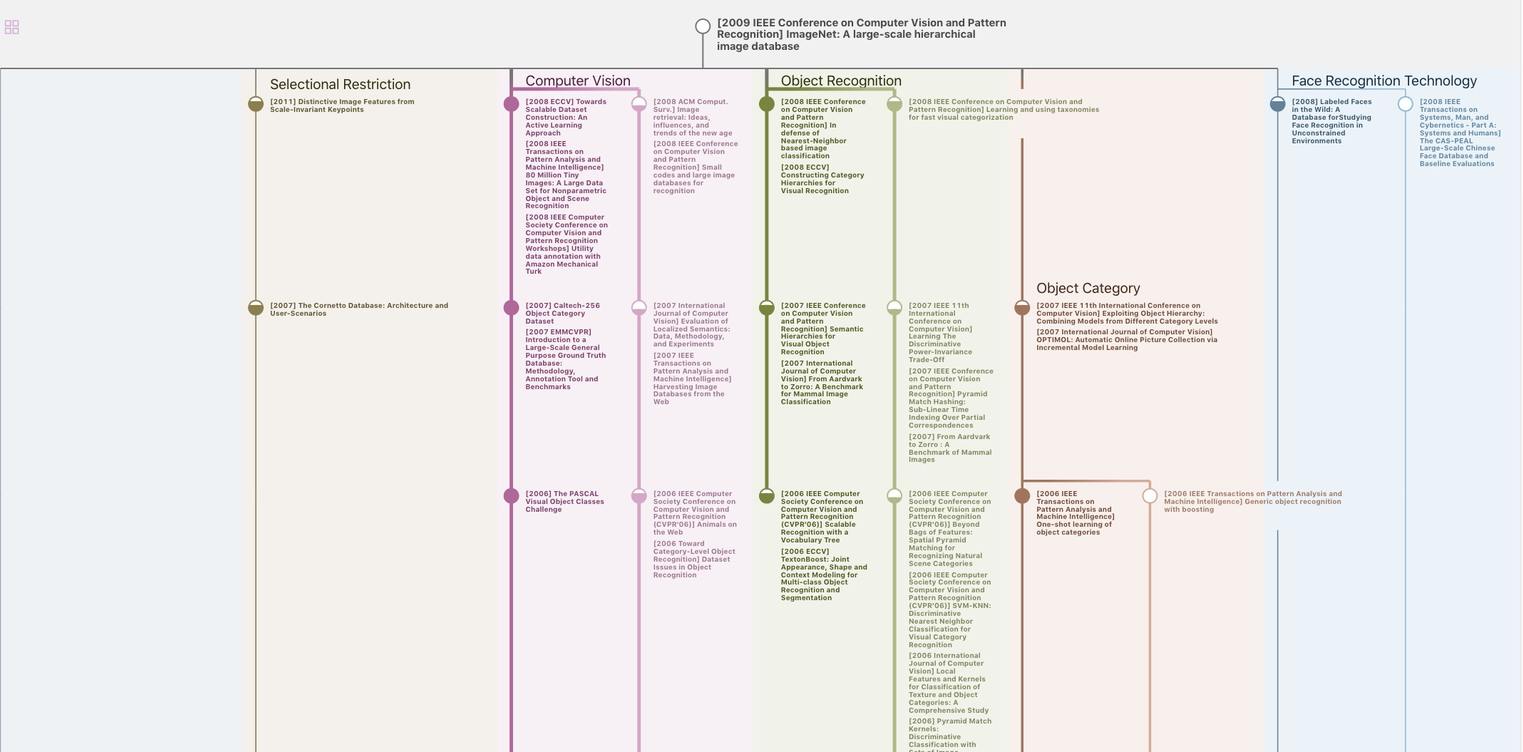
生成溯源树,研究论文发展脉络
Chat Paper
正在生成论文摘要