Vehicle Selection for C-V2X Mode 4 Based Federated Edge Learning Systems
CoRR(2024)
摘要
Federated learning (FL) is a promising technology for vehicular networks to
protect vehicles' privacy in Internet of Vehicles (IoV). Vehicles with limited
computation capacity may face a large computational burden associated with FL.
Federated edge learning (FEEL) systems are introduced to solve such a problem.
In FEEL systems, vehicles adopt the cellular-vehicle to everything (C-V2X) mode
4 to upload encrypted data to road side units' (RSUs)' cache queue. Then RSUs
train the data transmitted by vehicles, update the locally model
hyperparameters and send back results to vehicles, thus vehicles' computational
burden can be released. However, each RSU has limited cache queue. To maintain
the stability of cache queue and maximize the accuracy of model, it is
essential to select appropriate vehicles to upload data. The vehicle selection
method for FEEL systems faces challenges due to the random departure of data
from the cache queue caused by the stochastic channel and the different system
status of vehicles, such as remaining data amount, transmission delay, packet
collision probability and survival ability. This paper proposes a vehicle
selection method for FEEL systems that aims to maximize the accuracy of model
while keeping the cache queue stable. Extensive simulation experiments
demonstrate that our proposed method outperforms other baseline selection
methods.
更多查看译文
AI 理解论文
溯源树
样例
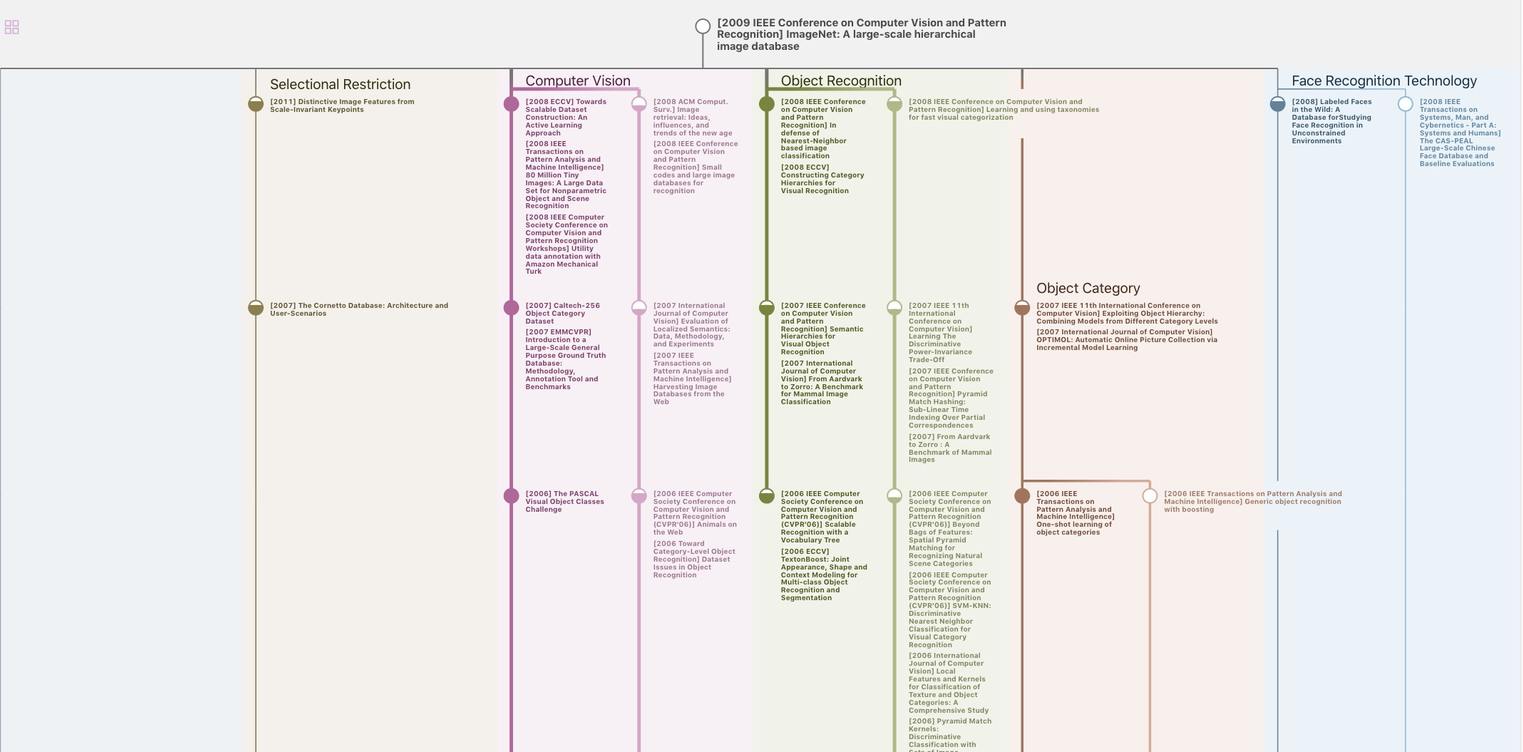
生成溯源树,研究论文发展脉络
Chat Paper
正在生成论文摘要