Accurate Loss Prediction of Realistic Hollow-Core Anti-Resonant Fibers Using Machine Learning
IEEE JOURNAL OF SELECTED TOPICS IN QUANTUM ELECTRONICS(2024)
摘要
Hollow-core anti-resonant fibers (HC-ARFs) have proven to be an indispensable platform for various emerging applications due to their unique and extraordinary optical properties. However, accurately estimating the propagation loss of nested HC-ARFs remains a challenging task due to their complex structure and the lack of precise analytical and theoretical models. To address this challenge, we propose a supervised machine-learning framework that presents an effective solution to accurately predict the propagation loss of a 5-tube nested HC-ARF. Multiple supervised learning models, including random forest, logistic regression, quadratic discriminant analysis, tree-based methods, extreme gradient boosting, and K-nearest neighbors are implemented and compared using a simulated dataset. Among these methods, the random forest algorithm is identified as the most effective, delivering accurate predictions. Notably, this study considers the impact of random structural perturbations on fiber geometry, encompassing random variations in tube wall thicknesses and tube gap separations. In particular, these perturbations involve randomly varying outer and nested tube wall thicknesses, tube angle offsets, and randomly distributed non-circular, anisotropic shapes within the cladding structure. It is worth noting that these specific perturbations have not been previously investigated. Each tube exhibits its unique set of random values, leading to longer simulation times for combinations of these values compared to regular random variables in HC-ARFs with similar tube characteristics. The comprehensive consideration of these factors allows for precise predictions, significantly contributing to the advancement of HC-ARFs for many emerging applications.
更多查看译文
关键词
Hollow-core anti-resonant fiber,machine learning,fiber geometry misalignment,finite-element simulation
AI 理解论文
溯源树
样例
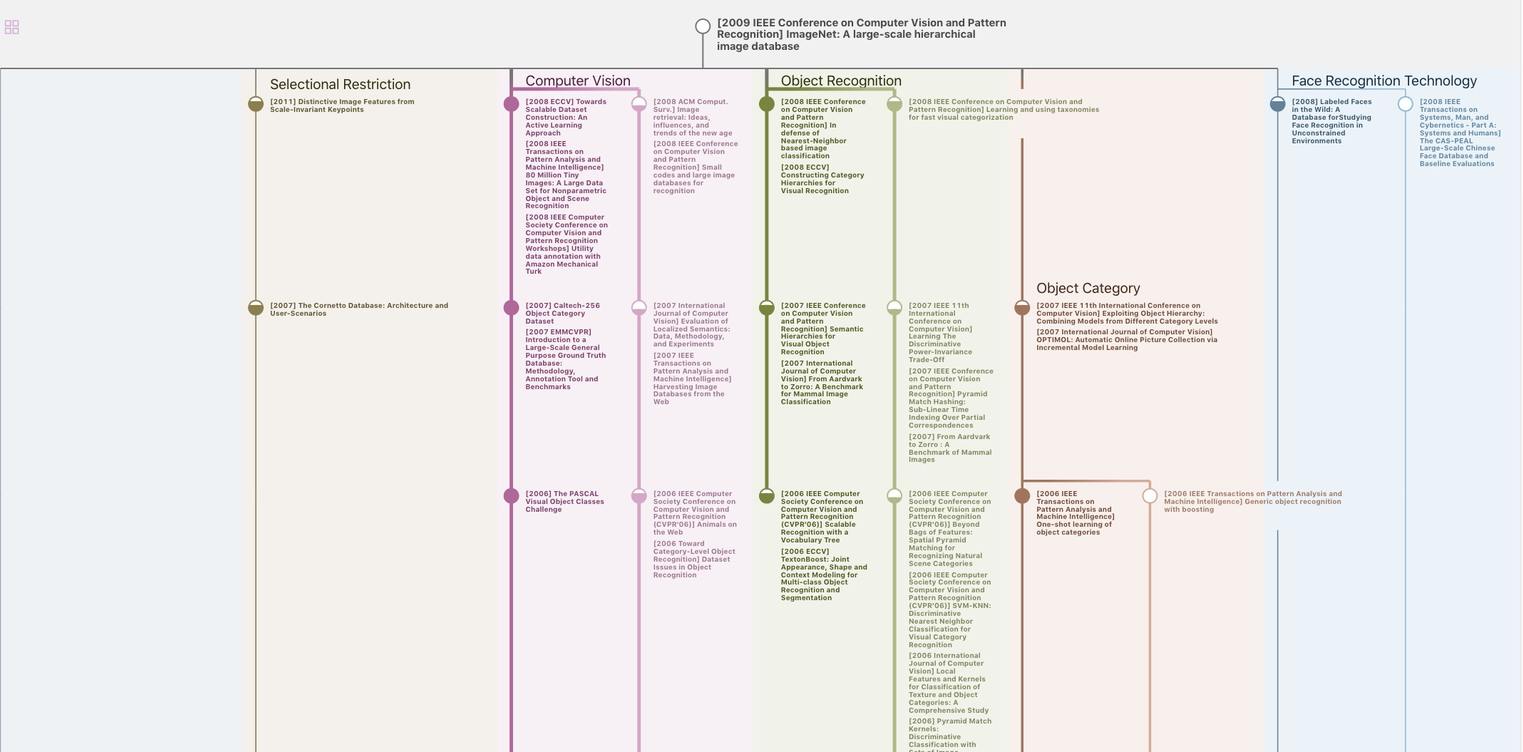
生成溯源树,研究论文发展脉络
Chat Paper
正在生成论文摘要