Physics-Informed Convolutional Decoder (PICD): A novel approach for direct inversion of heterogeneous subsurface flow
arxiv(2024)
摘要
In this study, we present the development and application of the
physics-informed convolutional decoder (PICD) framework for inverse modeling of
heterogenous groundwater flow. PICD stands out as a direct inversion method,
eliminating the need for repeated forward model simulations. The framework
leverages both data-driven and physics-driven approaches by integrating
monitoring data and domain knowledge (governing equation, boundary conditions,
and initial conditions) into the inversion process. PICD utilizes a
convolutional decoder to effectively approximate the spatial distribution of
hydraulic heads, while Karhunen Loeve expansion (KLE) is employed to
parameterize hydraulic conductivities. During the training process, the
stochastic vector in KLE and the parameters of the convolutional decoder are
adjusted simultaneously, ensuring that the predictions align with available
measurements and adhere to domain-specific knowledge. The final optimized
stochastic vectors correspond to the estimation of hydraulic conductivities,
and the trained convolutional decoder demonstrates the ability to predict the
evolution and distribution of hydraulic heads in heterogeneous fields. To
validate the effectiveness of the proposed PICD framework, various scenarios of
groundwater flow are examined. Results demonstrate the framework's capability
to accurately estimate heterogeneous hydraulic conductivities and to deliver
satisfactory predictions of hydraulic heads, even with sparse measurements. The
proposed PICD framework emerges as a promising tool for efficient and informed
groundwater flow inverse modeling.
更多查看译文
AI 理解论文
溯源树
样例
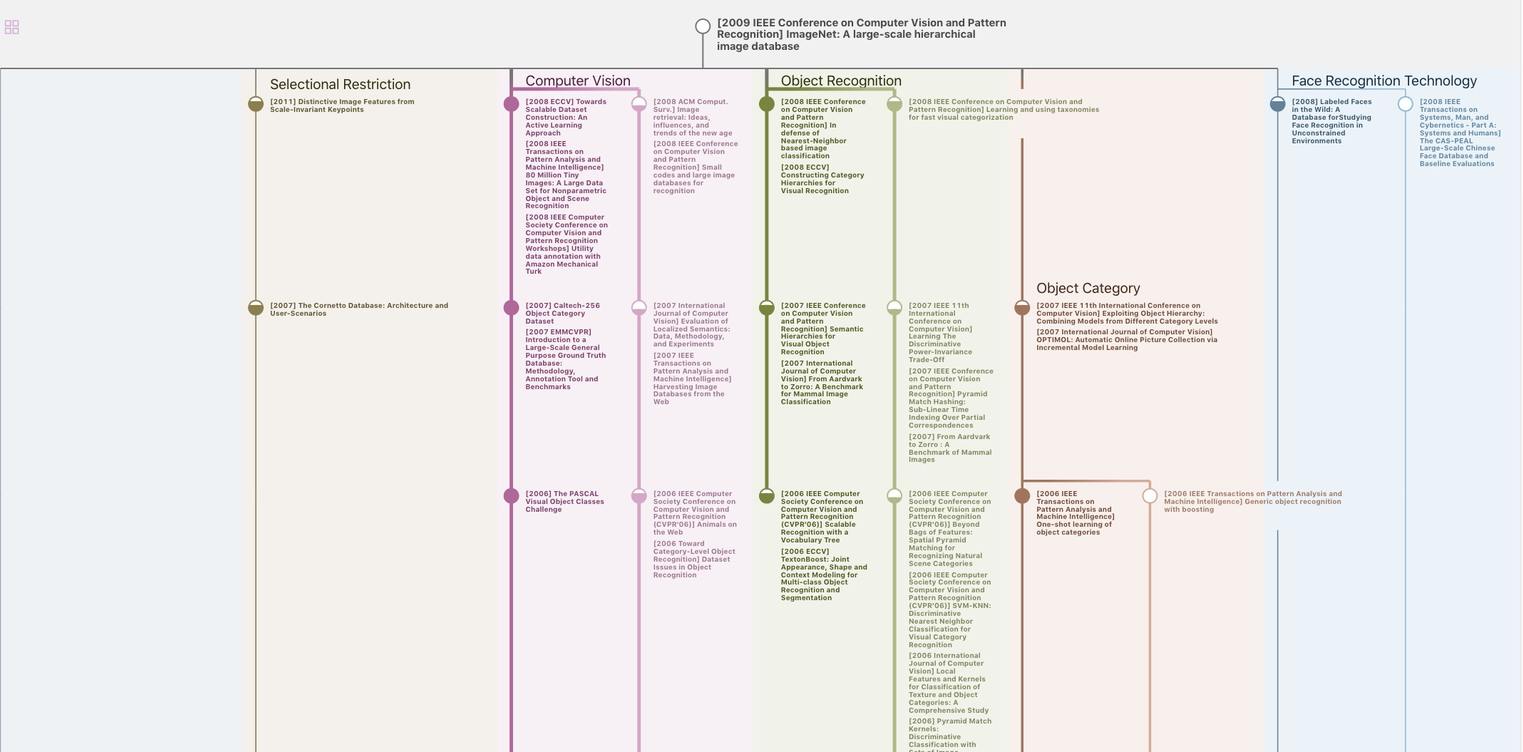
生成溯源树,研究论文发展脉络
Chat Paper
正在生成论文摘要