Greedy Newton: Newton's Method with Exact Line Search
arxiv(2024)
摘要
A defining characteristic of Newton's method is local superlinear convergence
within a neighbourhood of a strict local minimum. However, outside this
neighborhood Newton's method can converge slowly or even diverge. A common
approach to dealing with non-convergence is using a step size that is set by an
Armijo backtracking line search. With suitable initialization the line-search
preserves local superlinear convergence, but may give sub-optimal progress when
not near a solution. In this work we consider Newton's method under an exact
line search, which we call "greedy Newton" (GN). We show that this leads to an
improved global convergence rate, while retaining a local superlinear
convergence rate. We empirically show that GN may work better than backtracking
Newton by allowing significantly larger step sizes.
更多查看译文
AI 理解论文
溯源树
样例
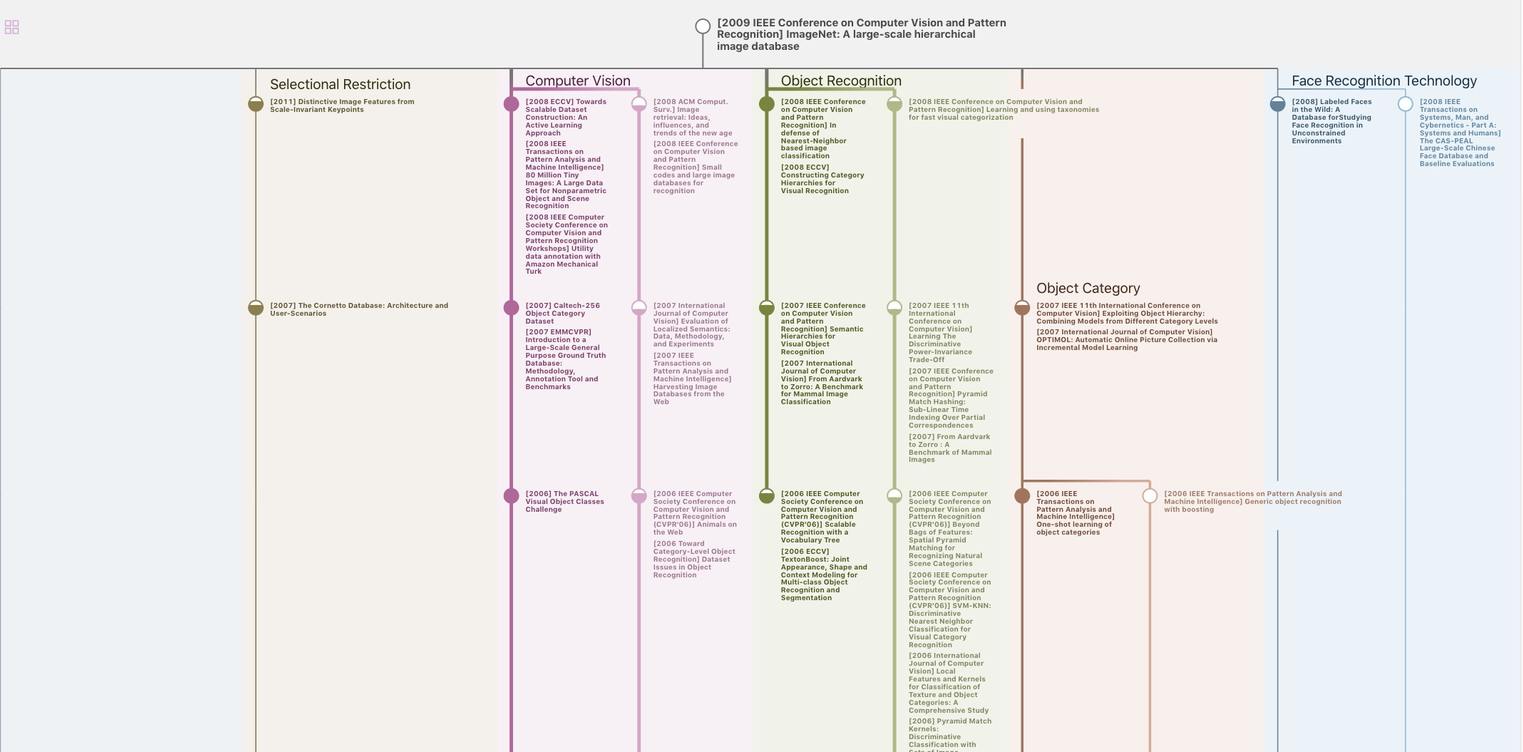
生成溯源树,研究论文发展脉络
Chat Paper
正在生成论文摘要