Exploring the Reasoning Abilities of Multimodal Large Language Models (MLLMs): A Comprehensive Survey on Emerging Trends in Multimodal Reasoning
CoRR(2024)
摘要
Strong Artificial Intelligence (Strong AI) or Artificial General Intelligence
(AGI) with abstract reasoning ability is the goal of next-generation AI. Recent
advancements in Large Language Models (LLMs), along with the emerging field of
Multimodal Large Language Models (MLLMs), have demonstrated impressive
capabilities across a wide range of multimodal tasks and applications.
Particularly, various MLLMs, each with distinct model architectures, training
data, and training stages, have been evaluated across a broad range of MLLM
benchmarks. These studies have, to varying degrees, revealed different aspects
of the current capabilities of MLLMs. However, the reasoning abilities of MLLMs
have not been systematically investigated. In this survey, we comprehensively
review the existing evaluation protocols of multimodal reasoning, categorize
and illustrate the frontiers of MLLMs, introduce recent trends in applications
of MLLMs on reasoning-intensive tasks, and finally discuss current practices
and future directions. We believe our survey establishes a solid base and sheds
light on this important topic, multimodal reasoning.
更多查看译文
AI 理解论文
溯源树
样例
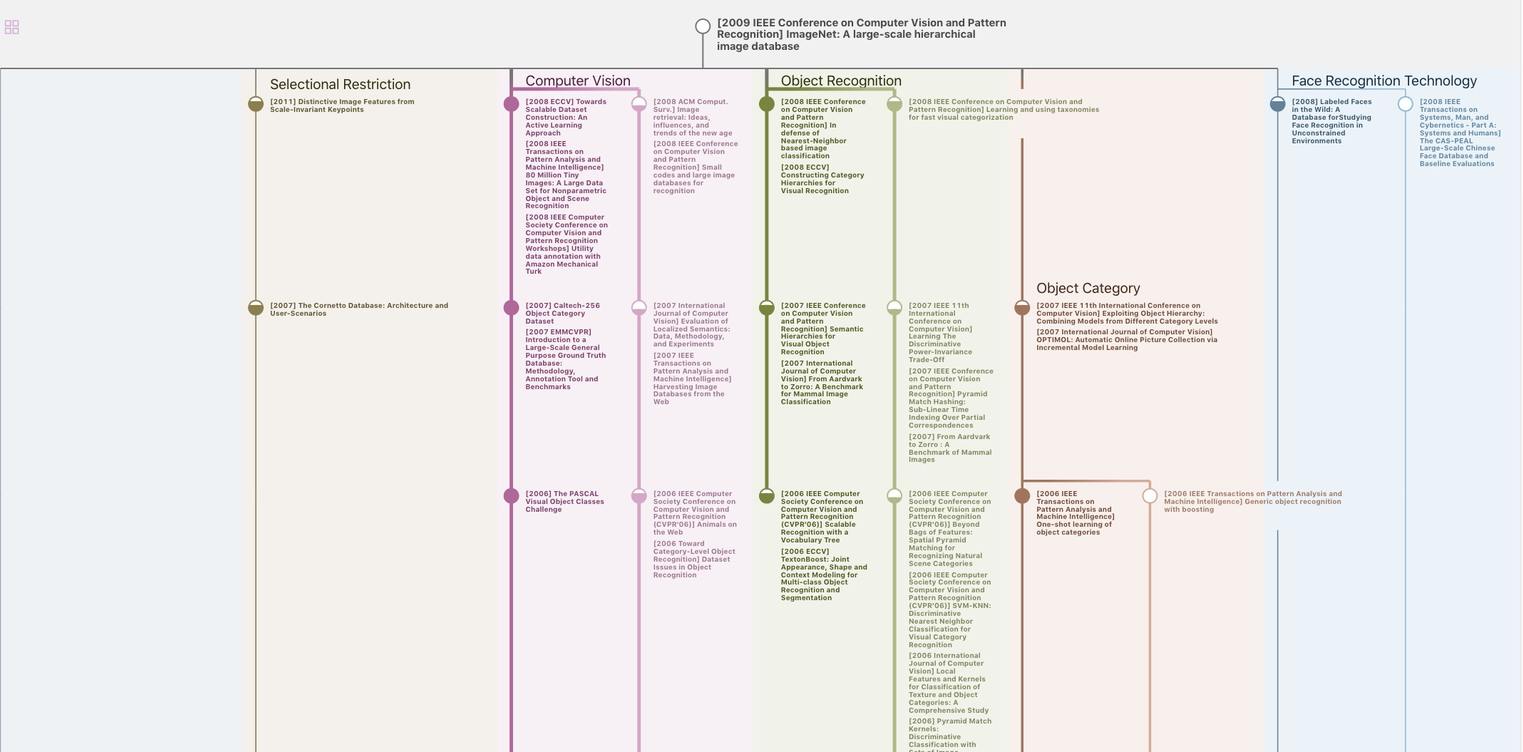
生成溯源树,研究论文发展脉络
Chat Paper
正在生成论文摘要