A Dual-Branch Framework With Prior Knowledge for Precise Segmentation of Lung Nodules in Challenging CT Scans
IEEE JOURNAL OF BIOMEDICAL AND HEALTH INFORMATICS(2024)
摘要
Lung cancer is one of the deadliest cancers globally, and early diagnosis is crucial for patient survival. Pulmonary nodules are the main manifestation of early lung cancer, usually assessed using CT scans. Nowadays, computer-aided diagnostic systems are widely used to assist physicians in disease diagnosis. The accurate segmentation of pulmonary nodules is affected by internal heterogeneity and external data factors. In order to overcome the segmentation challenges of subtle, mixed, adhesion-type, benign, and uncertain categories of nodules, a new mixed manual feature network that enhances sensitivity and accuracy is proposed. This method integrates feature information through a dual-branch network framework and multi-dimensional fusion module. By training and validating with multiple data sources and different data qualities, our method demonstrates leading performance on the LUNA16, Multi-thickness Slice Image dataset, LIDC, and UniToChest, with Dice similarity coefficients reaching 86.89%, 75.72%, 84.12%, and 80.74% respectively, surpassing most current methods for pulmonary nodule segmentation. Our method further improved the accuracy, reliability, and stability of lung nodule segmentation tasks even on challenging CT scans.
更多查看译文
关键词
Lung,Computed tomography,Image segmentation,Lung cancer,Medical services,Training,Lesions,Deep neural network,dual branch,feature fusion,lung nodule segmentation,mixed feature
AI 理解论文
溯源树
样例
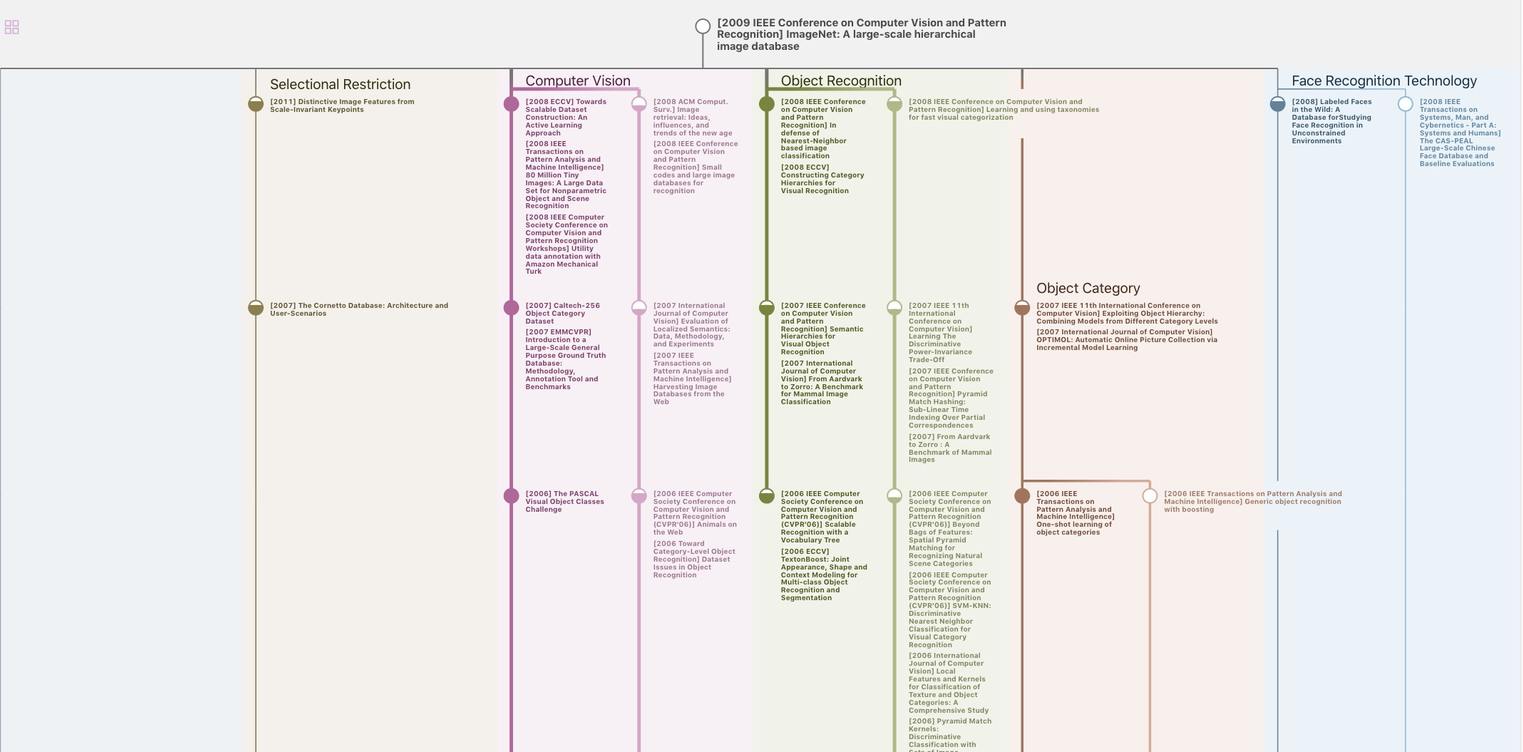
生成溯源树,研究论文发展脉络
Chat Paper
正在生成论文摘要