Bayesian design of concrete with amortized Gaussian processes and multi-objective optimization
CEMENT AND CONCRETE RESEARCH(2024)
摘要
Here, we present a computational framework, combining machine learning models with inverse optimization, which can accelerate and optimize concrete mix design with respect to climate impact and/or cost. Our approach leverages a novel amortized Gaussian process (GP) model trained on a large industry dataset to predict concrete strength based on mix proportions. The resulting GP model has an R2 value, RMSE, and MAPE of -0.88, -909 psi (6.3 MPa), and -10.8 %, respectively. We integrated the GP model with an inverse optimization scheme to predict optimal mix designs that minimize cost and/or climate impact. The results show that this integrated framework can generate reasonable concrete mixes that offer up to -30 % and -60 % reductions in cost and climate impact, respectively, compared with industry mixes with similar 28-day strength. This study highlights the potential environmental and economic benefits of data-driven approaches to designing and optimizing concrete mixes.
更多查看译文
关键词
Concrete mix design,Industrial data set,Machine learning,Gaussian process,Multi-objective optimization
AI 理解论文
溯源树
样例
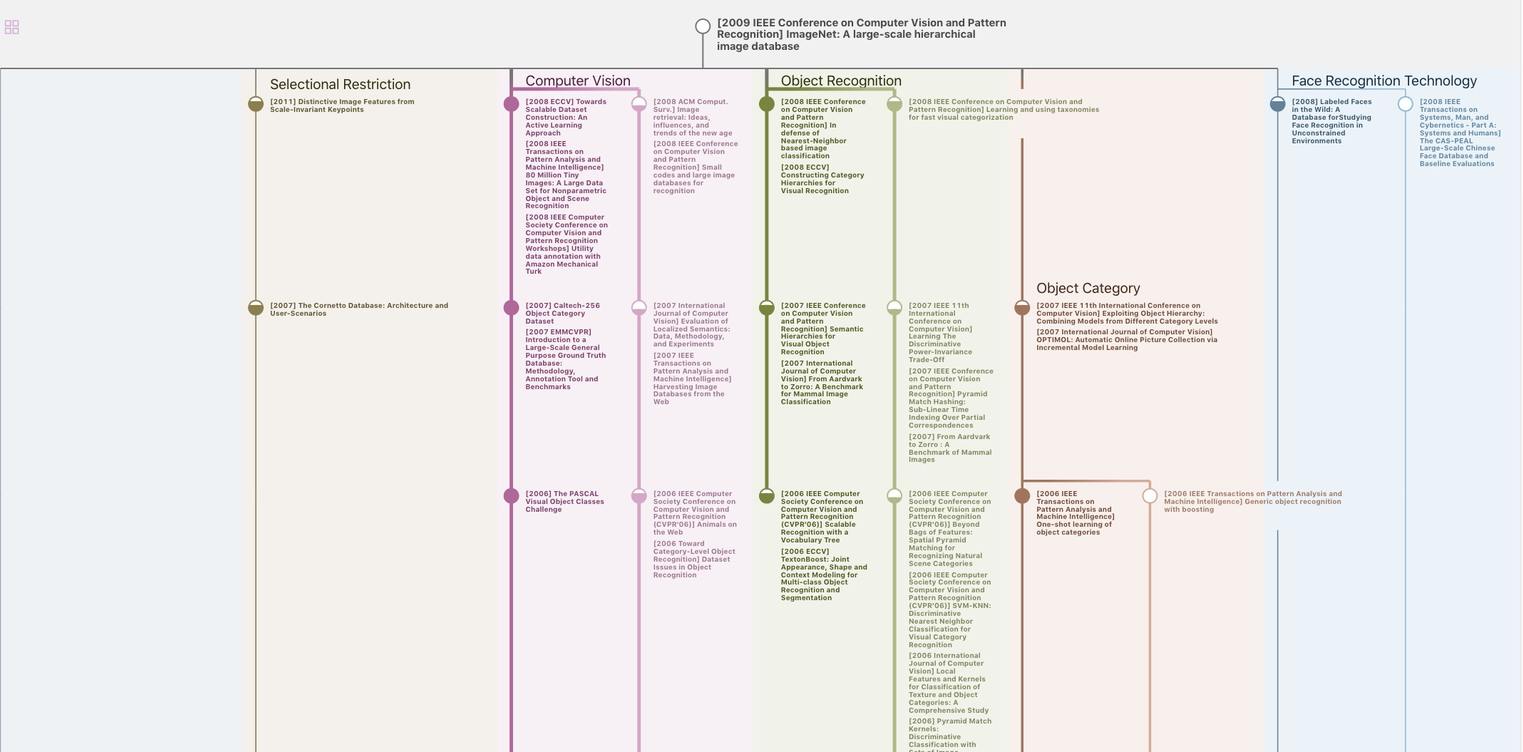
生成溯源树,研究论文发展脉络
Chat Paper
正在生成论文摘要